کد مقاله | کد نشریه | سال انتشار | مقاله انگلیسی | نسخه تمام متن |
---|---|---|---|---|
1993251 | 1541243 | 2015 | 7 صفحه PDF | دانلود رایگان |
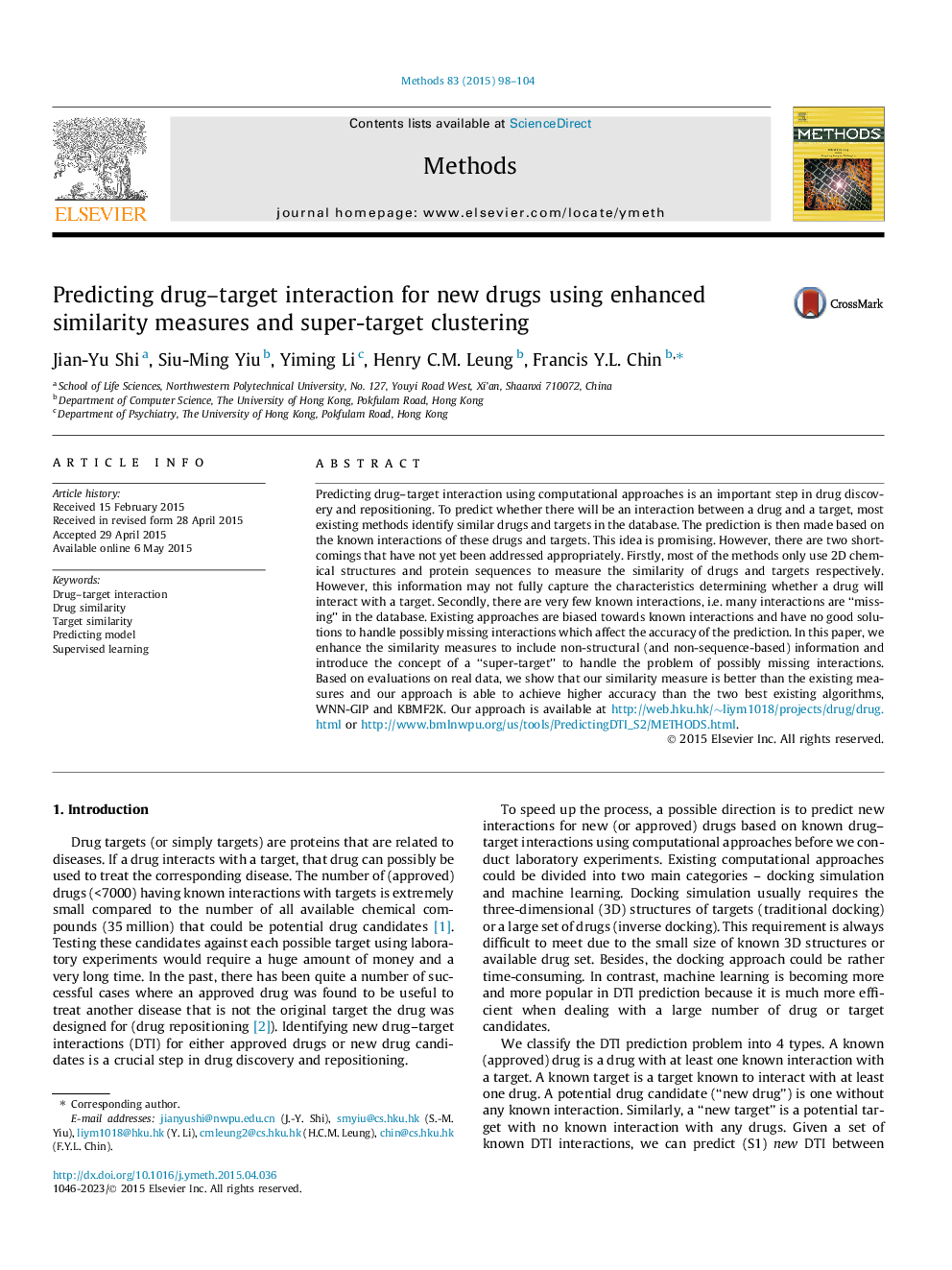
• DTI prediction is modeled via estimating a posterior probability of being a DTI.
• ATC-based and structural similarities mutually reinforce drug similarity metric.
• Functional and sequence similarities jointly enhance target similarity metric.
• The “super-target” can relax the hardness caused by possibly missing interactions.
Predicting drug–target interaction using computational approaches is an important step in drug discovery and repositioning. To predict whether there will be an interaction between a drug and a target, most existing methods identify similar drugs and targets in the database. The prediction is then made based on the known interactions of these drugs and targets. This idea is promising. However, there are two shortcomings that have not yet been addressed appropriately. Firstly, most of the methods only use 2D chemical structures and protein sequences to measure the similarity of drugs and targets respectively. However, this information may not fully capture the characteristics determining whether a drug will interact with a target. Secondly, there are very few known interactions, i.e. many interactions are “missing” in the database. Existing approaches are biased towards known interactions and have no good solutions to handle possibly missing interactions which affect the accuracy of the prediction. In this paper, we enhance the similarity measures to include non-structural (and non-sequence-based) information and introduce the concept of a “super-target” to handle the problem of possibly missing interactions. Based on evaluations on real data, we show that our similarity measure is better than the existing measures and our approach is able to achieve higher accuracy than the two best existing algorithms, WNN-GIP and KBMF2K. Our approach is available at http://web.hku.hk/∼liym1018/projects/drug/drug.html or http://www.bmlnwpu.org/us/tools/PredictingDTI_S2/METHODS.html.
Journal: Methods - Volume 83, 15 July 2015, Pages 98–104