کد مقاله | کد نشریه | سال انتشار | مقاله انگلیسی | نسخه تمام متن |
---|---|---|---|---|
6268082 | 1614612 | 2016 | 10 صفحه PDF | دانلود رایگان |
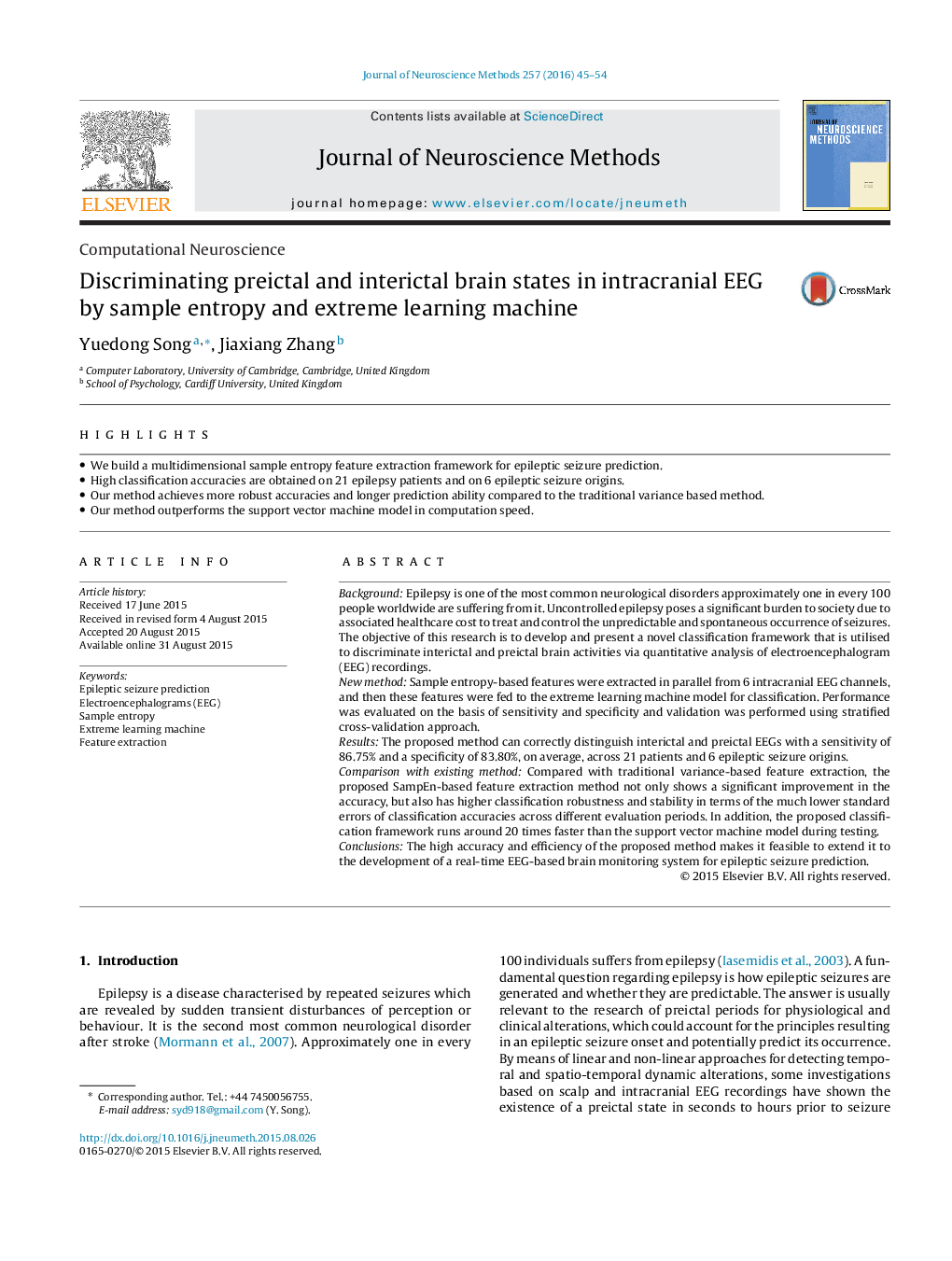
- We build a multidimensional sample entropy feature extraction framework for epileptic seizure prediction.
- High classification accuracies are obtained on 21 epilepsy patients and on 6 epileptic seizure origins.
- Our method achieves more robust accuracies and longer prediction ability compared to the traditional variance based method.
- Our method outperforms the support vector machine model in computation speed.
BackgroundEpilepsy is one of the most common neurological disorders approximately one in every 100 people worldwide are suffering from it. Uncontrolled epilepsy poses a significant burden to society due to associated healthcare cost to treat and control the unpredictable and spontaneous occurrence of seizures. The objective of this research is to develop and present a novel classification framework that is utilised to discriminate interictal and preictal brain activities via quantitative analysis of electroencephalogram (EEG) recordings.New methodSample entropy-based features were extracted in parallel from 6 intracranial EEG channels, and then these features were fed to the extreme learning machine model for classification. Performance was evaluated on the basis of sensitivity and specificity and validation was performed using stratified cross-validation approach.ResultsThe proposed method can correctly distinguish interictal and preictal EEGs with a sensitivity of 86.75% and a specificity of 83.80%, on average, across 21 patients and 6 epileptic seizure origins.Comparison with existing methodCompared with traditional variance-based feature extraction, the proposed SampEn-based feature extraction method not only shows a significant improvement in the accuracy, but also has higher classification robustness and stability in terms of the much lower standard errors of classification accuracies across different evaluation periods. In addition, the proposed classification framework runs around 20 times faster than the support vector machine model during testing.ConclusionsThe high accuracy and efficiency of the proposed method makes it feasible to extend it to the development of a real-time EEG-based brain monitoring system for epileptic seizure prediction.
Journal: Journal of Neuroscience Methods - Volume 257, 15 January 2016, Pages 45-54