کد مقاله | کد نشریه | سال انتشار | مقاله انگلیسی | نسخه تمام متن |
---|---|---|---|---|
11023909 | 1701235 | 2019 | 16 صفحه PDF | دانلود رایگان |
عنوان انگلیسی مقاله ISI
SSTS: A syntactic tool for pattern search on time series
دانلود مقاله + سفارش ترجمه
دانلود مقاله ISI انگلیسی
رایگان برای ایرانیان
کلمات کلیدی
موضوعات مرتبط
مهندسی و علوم پایه
مهندسی کامپیوتر
نرم افزارهای علوم کامپیوتر
پیش نمایش صفحه اول مقاله
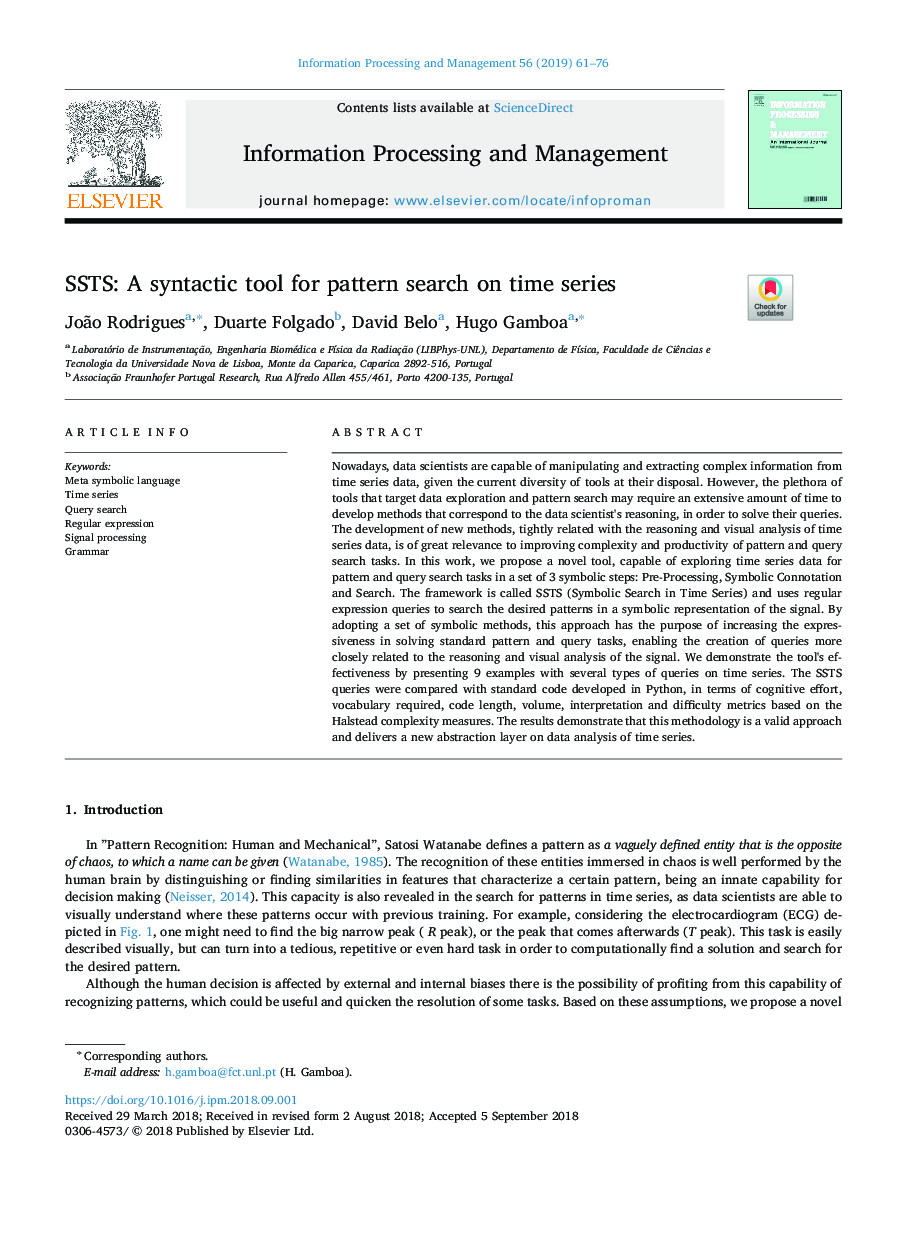
چکیده انگلیسی
Nowadays, data scientists are capable of manipulating and extracting complex information from time series data, given the current diversity of tools at their disposal. However, the plethora of tools that target data exploration and pattern search may require an extensive amount of time to develop methods that correspond to the data scientist's reasoning, in order to solve their queries. The development of new methods, tightly related with the reasoning and visual analysis of time series data, is of great relevance to improving complexity and productivity of pattern and query search tasks. In this work, we propose a novel tool, capable of exploring time series data for pattern and query search tasks in a set of 3 symbolic steps: Pre-Processing, Symbolic Connotation and Search. The framework is called SSTS (Symbolic Search in Time Series) and uses regular expression queries to search the desired patterns in a symbolic representation of the signal. By adopting a set of symbolic methods, this approach has the purpose of increasing the expressiveness in solving standard pattern and query tasks, enabling the creation of queries more closely related to the reasoning and visual analysis of the signal. We demonstrate the tool's effectiveness by presenting 9 examples with several types of queries on time series. The SSTS queries were compared with standard code developed in Python, in terms of cognitive effort, vocabulary required, code length, volume, interpretation and difficulty metrics based on the Halstead complexity measures. The results demonstrate that this methodology is a valid approach and delivers a new abstraction layer on data analysis of time series.
ناشر
Database: Elsevier - ScienceDirect (ساینس دایرکت)
Journal: Information Processing & Management - Volume 56, Issue 1, January 2019, Pages 61-76
Journal: Information Processing & Management - Volume 56, Issue 1, January 2019, Pages 61-76
نویسندگان
João Rodrigues, Duarte Folgado, David Belo, Hugo Gamboa,