کد مقاله | کد نشریه | سال انتشار | مقاله انگلیسی | نسخه تمام متن |
---|---|---|---|---|
1133228 | 1489071 | 2016 | 12 صفحه PDF | دانلود رایگان |
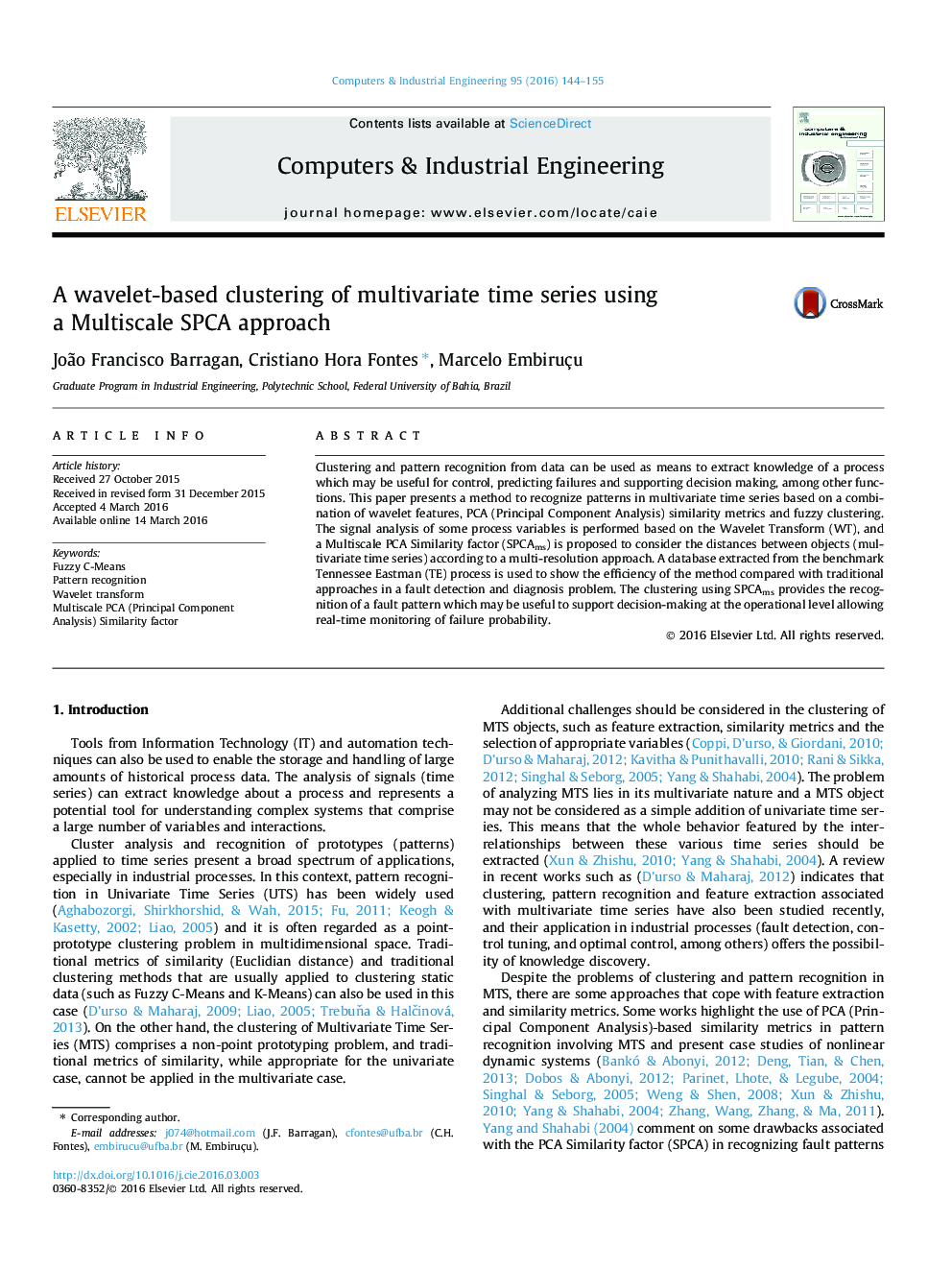
• Case study comprising the fault detection in a benchmark industrial process.
• Comprehensive and novel method for clustering multivariate time series.
• Multiscale approach provides improvement in clustering quality.
• Multiscale approach represents a useful technique in FDD problems.
• Results show the ability of the method in recognizing normal and fault patterns.
Clustering and pattern recognition from data can be used as means to extract knowledge of a process which may be useful for control, predicting failures and supporting decision making, among other functions. This paper presents a method to recognize patterns in multivariate time series based on a combination of wavelet features, PCA (Principal Component Analysis) similarity metrics and fuzzy clustering. The signal analysis of some process variables is performed based on the Wavelet Transform (WT), and a Multiscale PCA Similarity factor (SPCAms) is proposed to consider the distances between objects (multivariate time series) according to a multi-resolution approach. A database extracted from the benchmark Tennessee Eastman (TE) process is used to show the efficiency of the method compared with traditional approaches in a fault detection and diagnosis problem. The clustering using SPCAms provides the recognition of a fault pattern which may be useful to support decision-making at the operational level allowing real-time monitoring of failure probability.
Journal: Computers & Industrial Engineering - Volume 95, May 2016, Pages 144–155