کد مقاله | کد نشریه | سال انتشار | مقاله انگلیسی | نسخه تمام متن |
---|---|---|---|---|
1133550 | 1489079 | 2015 | 10 صفحه PDF | دانلود رایگان |
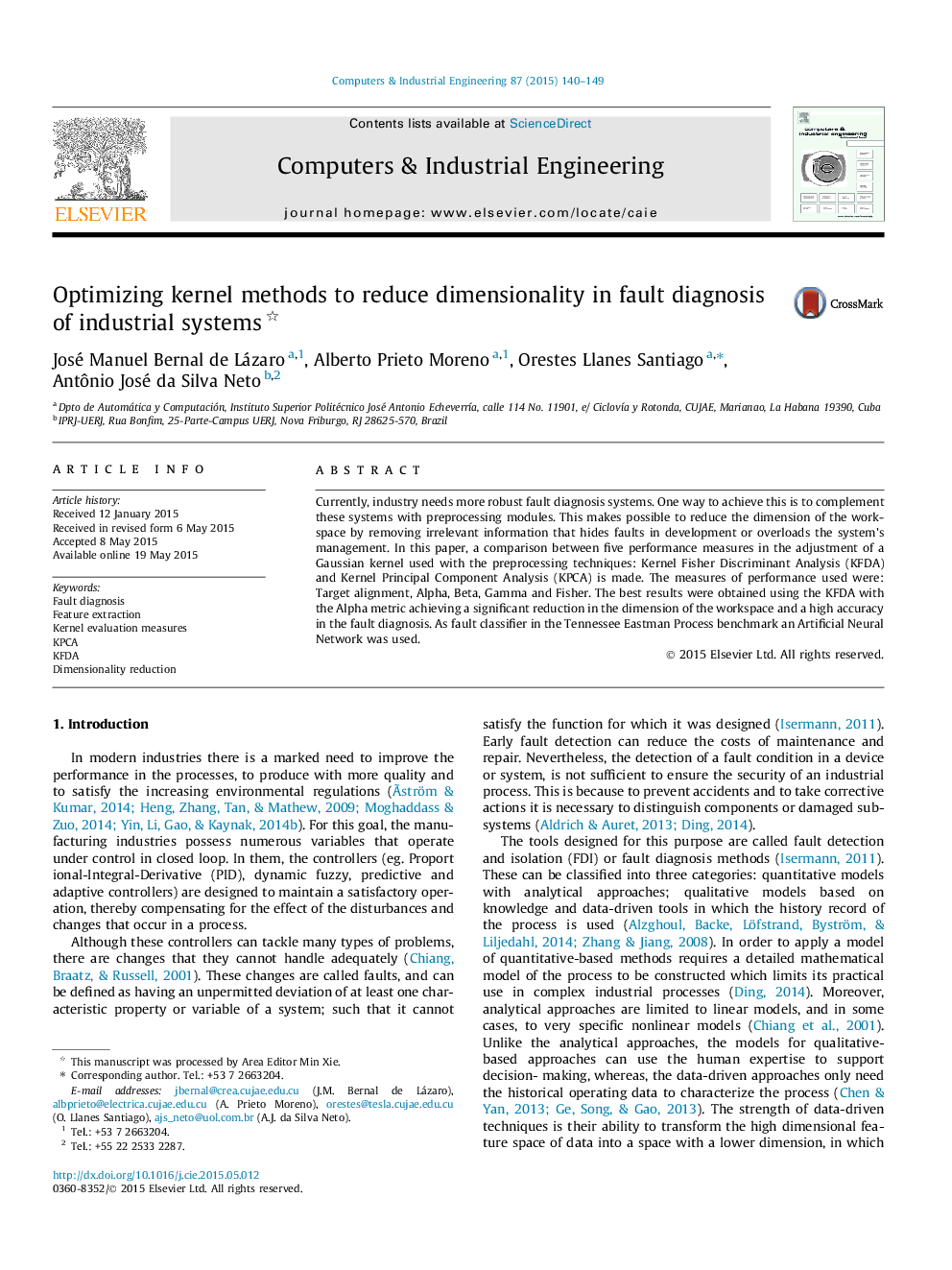
• Five measures to adjust a RBF Kernel using KFDA and KPCA in FDI problems are compared.
• The influence of the KPCA and KFDA parameters in FDI problems are analyzed.
• The adjust of the kernel parameters with the measures α, β and γ is presented.
• The combination KFDAα obtained the best results in the classification.
Currently, industry needs more robust fault diagnosis systems. One way to achieve this is to complement these systems with preprocessing modules. This makes possible to reduce the dimension of the workspace by removing irrelevant information that hides faults in development or overloads the system’s management. In this paper, a comparison between five performance measures in the adjustment of a Gaussian kernel used with the preprocessing techniques: Kernel Fisher Discriminant Analysis (KFDA) and Kernel Principal Component Analysis (KPCA) is made. The measures of performance used were: Target alignment, Alpha, Beta, Gamma and Fisher. The best results were obtained using the KFDA with the Alpha metric achieving a significant reduction in the dimension of the workspace and a high accuracy in the fault diagnosis. As fault classifier in the Tennessee Eastman Process benchmark an Artificial Neural Network was used.
Journal: Computers & Industrial Engineering - Volume 87, September 2015, Pages 140–149