کد مقاله | کد نشریه | سال انتشار | مقاله انگلیسی | نسخه تمام متن |
---|---|---|---|---|
13430214 | 1842389 | 2020 | 12 صفحه PDF | دانلود رایگان |
عنوان انگلیسی مقاله ISI
Combining attention-based bidirectional gated recurrent neural network and two-dimensional convolutional neural network for document-level sentiment classification
دانلود مقاله + سفارش ترجمه
دانلود مقاله ISI انگلیسی
رایگان برای ایرانیان
موضوعات مرتبط
مهندسی و علوم پایه
مهندسی کامپیوتر
هوش مصنوعی
پیش نمایش صفحه اول مقاله
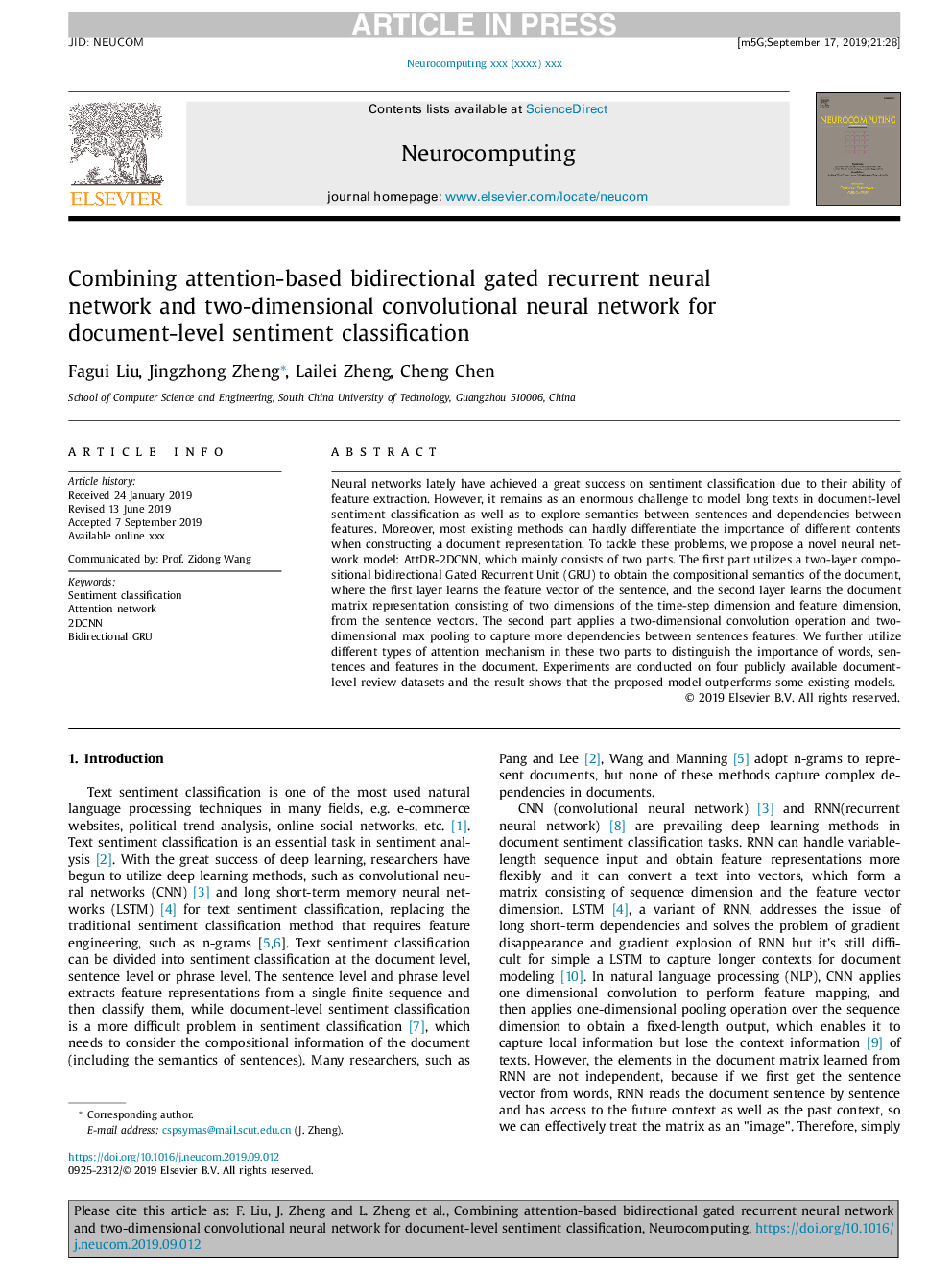
چکیده انگلیسی
Neural networks lately have achieved a great success on sentiment classification due to their ability of feature extraction. However, it remains as an enormous challenge to model long texts in document-level sentiment classification as well as to explore semantics between sentences and dependencies between features. Moreover, most existing methods can hardly differentiate the importance of different contents when constructing a document representation. To tackle these problems, we propose a novel neural network model: AttDR-2DCNN, which mainly consists of two parts. The first part utilizes a two-layer compositional bidirectional Gated Recurrent Unit (GRU) to obtain the compositional semantics of the document, where the first layer learns the feature vector of the sentence, and the second layer learns the document matrix representation consisting of two dimensions of the time-step dimension and feature dimension, from the sentence vectors. The second part applies a two-dimensional convolution operation and two-dimensional max pooling to capture more dependencies between sentences features. We further utilize different types of attention mechanism in these two parts to distinguish the importance of words, sentences and features in the document. Experiments are conducted on four publicly available document-level review datasets and the result shows that the proposed model outperforms some existing models.
ناشر
Database: Elsevier - ScienceDirect (ساینس دایرکت)
Journal: Neurocomputing - Volume 371, 2 January 2020, Pages 39-50
Journal: Neurocomputing - Volume 371, 2 January 2020, Pages 39-50
نویسندگان
Liu Fagui, Zheng Jingzhong, Zheng Lailei, Chen Cheng,