کد مقاله | کد نشریه | سال انتشار | مقاله انگلیسی | نسخه تمام متن |
---|---|---|---|---|
13455230 | 1844322 | 2020 | 18 صفحه PDF | دانلود رایگان |
عنوان انگلیسی مقاله ISI
Investigating single image super-resolution algorithm with deep learning using convolutional neural network for chest digital tomosynthesis
دانلود مقاله + سفارش ترجمه
دانلود مقاله ISI انگلیسی
رایگان برای ایرانیان
کلمات کلیدی
موضوعات مرتبط
مهندسی و علوم پایه
سایر رشته های مهندسی
مهندسی (عمومی)
پیش نمایش صفحه اول مقاله
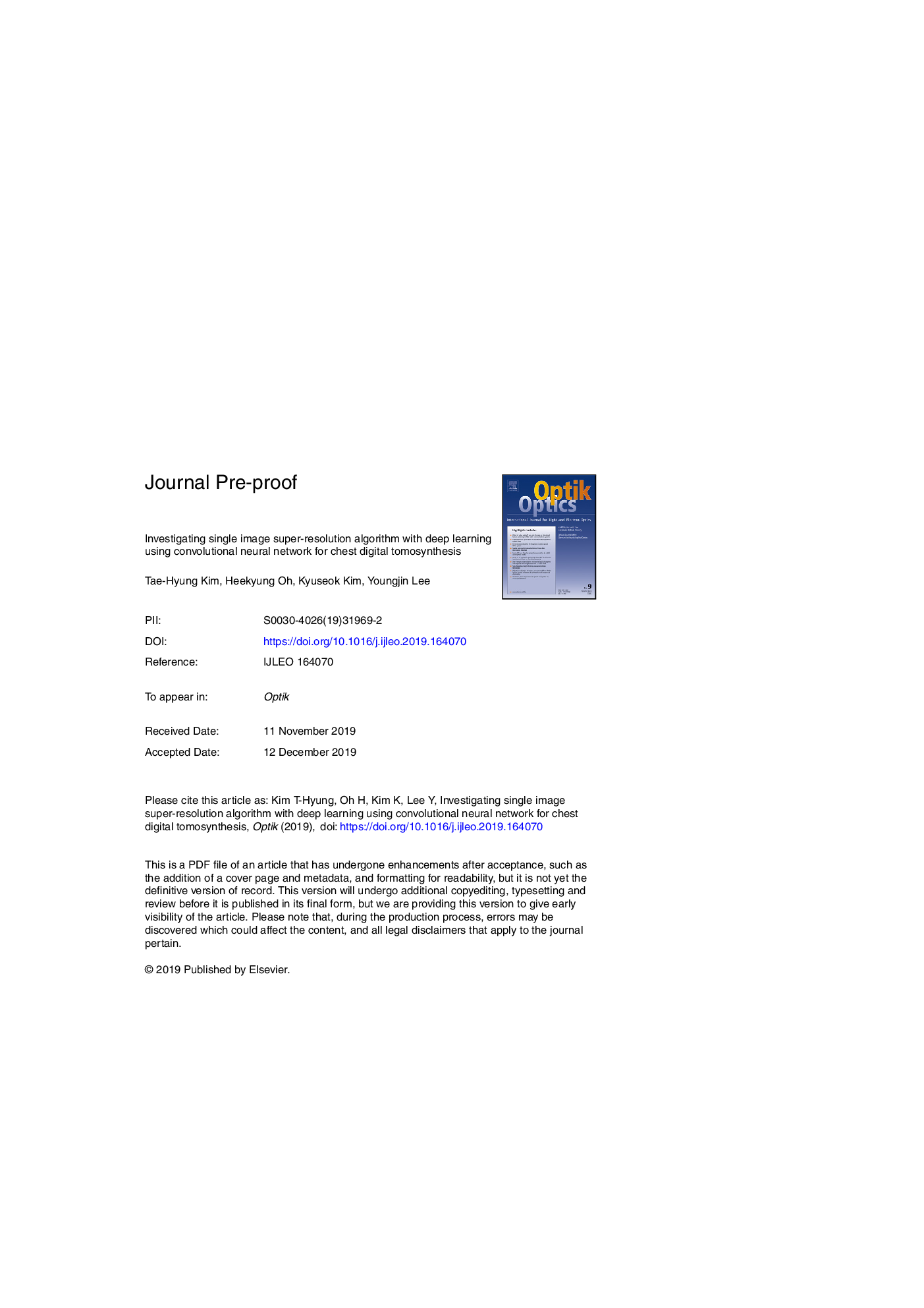
چکیده انگلیسی
Numerous efforts have been continuously made toward the realization of high spatial resolution images for medical imaging devices. Specifically, the image super-resolution technique with deep learning using convolutional neural network (CNN) has been making excellent advancements recently. Accordingly, this study is focused on developing a single image super-resolution (SISR) algorithm using deep CNN (DCNN) with supervised learning that can drastically improve the spatial resolution of chest digital tomosynthesis (CDT) images. In addition, we attempt to demonstrate the superiority of the SISR algorithm by a quantitative analysis. The proposed SISR algorithm uses a total of 5000 training CDT images (low-resolution and high-resolution) and a fully CNN based on residual structure. The image performance was analyzed using various parameters, such as intensity profile (full width at half maximum), contrast to noise ratio, coefficient of variation, and normalized noise power spectrum parameters, and the results demonstrated that the proposed SISR algorithm significantly improves the spatial resolution of the images. Further, the noise properties of the images obtained with the SISR algorithm were similar to those of the low-resolution images with up-sampling. Thus, we successfully developed the deep learning architectures in this study to improve the spatial resolution of the CDT reconstructed images.
ناشر
Database: Elsevier - ScienceDirect (ساینس دایرکت)
Journal: Optik - Volume 203, February 2020, 164070
Journal: Optik - Volume 203, February 2020, 164070
نویسندگان
Tae-Hyung Kim, Heekyung Oh, Kyuseok Kim, Youngjin Lee,