کد مقاله | کد نشریه | سال انتشار | مقاله انگلیسی | نسخه تمام متن |
---|---|---|---|---|
1712190 | 1013123 | 2009 | 17 صفحه PDF | دانلود رایگان |
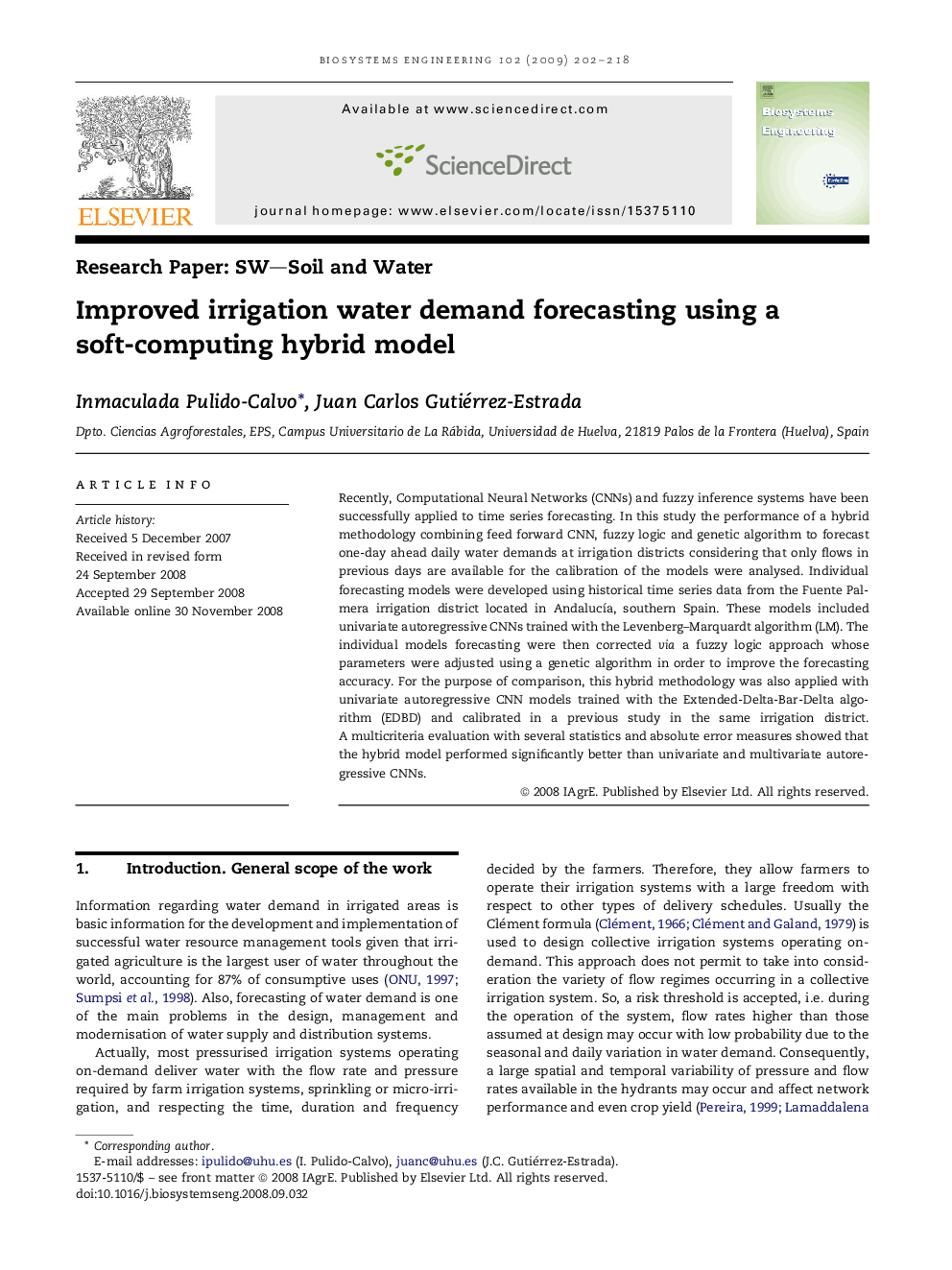
Recently, Computational Neural Networks (CNNs) and fuzzy inference systems have been successfully applied to time series forecasting. In this study the performance of a hybrid methodology combining feed forward CNN, fuzzy logic and genetic algorithm to forecast one-day ahead daily water demands at irrigation districts considering that only flows in previous days are available for the calibration of the models were analysed. Individual forecasting models were developed using historical time series data from the Fuente Palmera irrigation district located in Andalucía, southern Spain. These models included univariate autoregressive CNNs trained with the Levenberg–Marquardt algorithm (LM). The individual models forecasting were then corrected via a fuzzy logic approach whose parameters were adjusted using a genetic algorithm in order to improve the forecasting accuracy. For the purpose of comparison, this hybrid methodology was also applied with univariate autoregressive CNN models trained with the Extended-Delta-Bar-Delta algorithm (EDBD) and calibrated in a previous study in the same irrigation district. A multicriteria evaluation with several statistics and absolute error measures showed that the hybrid model performed significantly better than univariate and multivariate autoregressive CNNs.
Journal: Biosystems Engineering - Volume 102, Issue 2, February 2009, Pages 202–218