کد مقاله | کد نشریه | سال انتشار | مقاله انگلیسی | نسخه تمام متن |
---|---|---|---|---|
243347 | 501927 | 2012 | 12 صفحه PDF | دانلود رایگان |
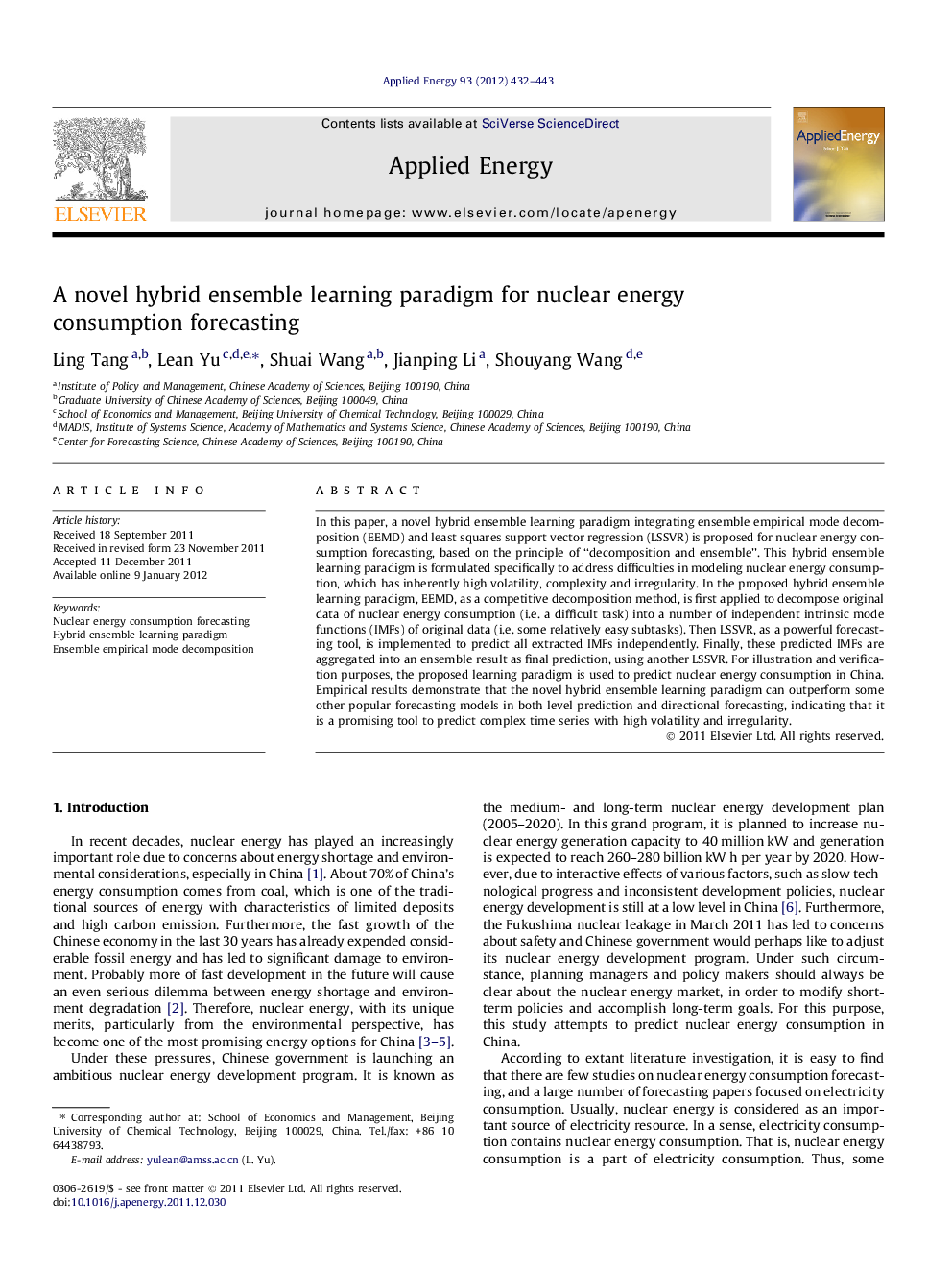
In this paper, a novel hybrid ensemble learning paradigm integrating ensemble empirical mode decomposition (EEMD) and least squares support vector regression (LSSVR) is proposed for nuclear energy consumption forecasting, based on the principle of “decomposition and ensemble”. This hybrid ensemble learning paradigm is formulated specifically to address difficulties in modeling nuclear energy consumption, which has inherently high volatility, complexity and irregularity. In the proposed hybrid ensemble learning paradigm, EEMD, as a competitive decomposition method, is first applied to decompose original data of nuclear energy consumption (i.e. a difficult task) into a number of independent intrinsic mode functions (IMFs) of original data (i.e. some relatively easy subtasks). Then LSSVR, as a powerful forecasting tool, is implemented to predict all extracted IMFs independently. Finally, these predicted IMFs are aggregated into an ensemble result as final prediction, using another LSSVR. For illustration and verification purposes, the proposed learning paradigm is used to predict nuclear energy consumption in China. Empirical results demonstrate that the novel hybrid ensemble learning paradigm can outperform some other popular forecasting models in both level prediction and directional forecasting, indicating that it is a promising tool to predict complex time series with high volatility and irregularity.
► A hybrid ensemble learning paradigm integrating EEMD and LSSVR is proposed.
► The hybrid ensemble method is useful to predict time series with high volatility.
► The ensemble method can be used for both one-step and multi-step ahead forecasting.
Journal: Applied Energy - Volume 93, May 2012, Pages 432–443