کد مقاله | کد نشریه | سال انتشار | مقاله انگلیسی | نسخه تمام متن |
---|---|---|---|---|
3042791 | 1184961 | 2015 | 8 صفحه PDF | دانلود رایگان |
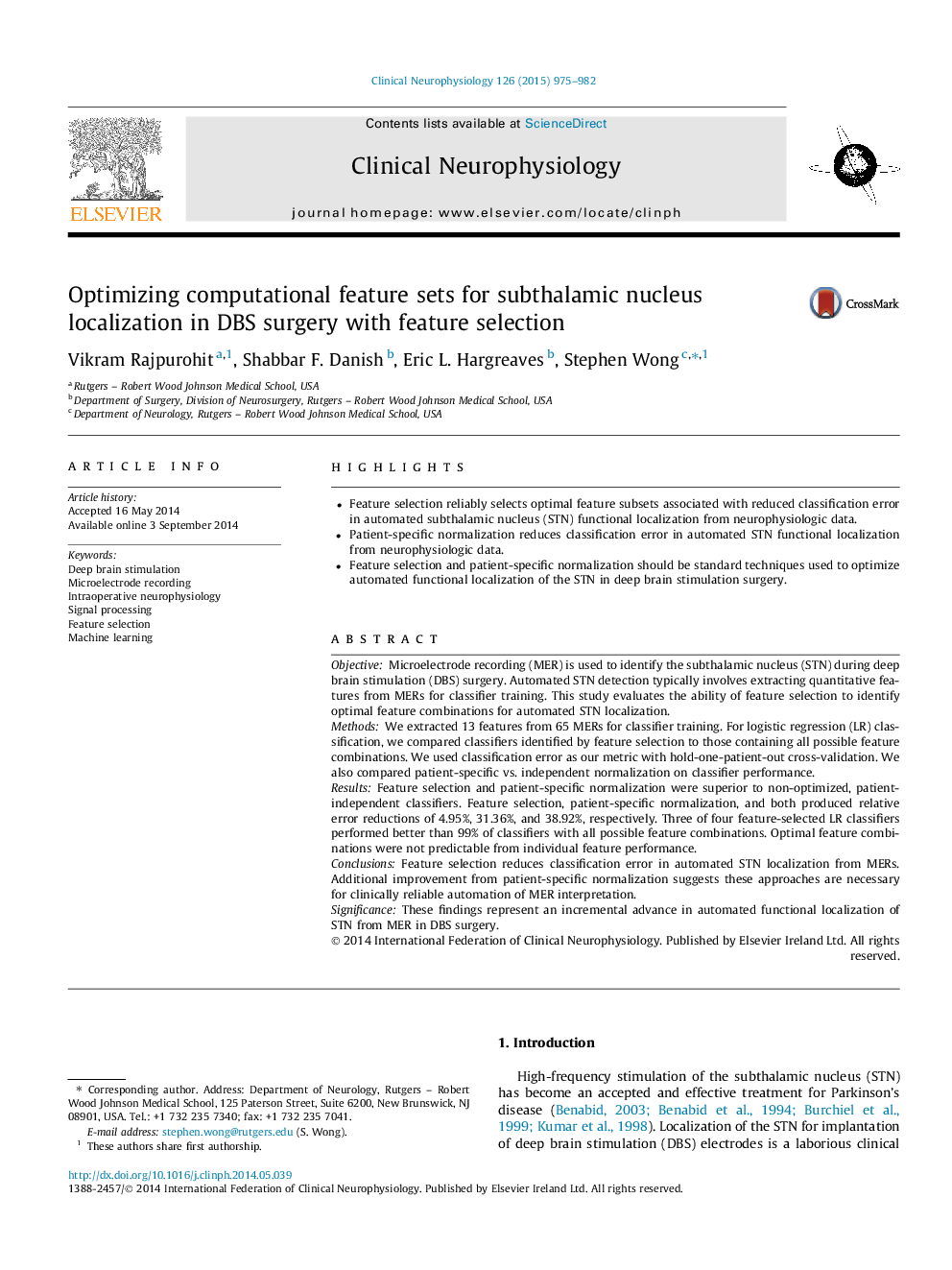
• Feature selection reliably selects optimal feature subsets associated with reduced classification error in automated subthalamic nucleus (STN) functional localization from neurophysiologic data.
• Patient-specific normalization reduces classification error in automated STN functional localization from neurophysiologic data.
• Feature selection and patient-specific normalization should be standard techniques used to optimize automated functional localization of the STN in deep brain stimulation surgery.
ObjectiveMicroelectrode recording (MER) is used to identify the subthalamic nucleus (STN) during deep brain stimulation (DBS) surgery. Automated STN detection typically involves extracting quantitative features from MERs for classifier training. This study evaluates the ability of feature selection to identify optimal feature combinations for automated STN localization.MethodsWe extracted 13 features from 65 MERs for classifier training. For logistic regression (LR) classification, we compared classifiers identified by feature selection to those containing all possible feature combinations. We used classification error as our metric with hold-one-patient-out cross-validation. We also compared patient-specific vs. independent normalization on classifier performance.ResultsFeature selection and patient-specific normalization were superior to non-optimized, patient-independent classifiers. Feature selection, patient-specific normalization, and both produced relative error reductions of 4.95%, 31.36%, and 38.92%, respectively. Three of four feature-selected LR classifiers performed better than 99% of classifiers with all possible feature combinations. Optimal feature combinations were not predictable from individual feature performance.ConclusionsFeature selection reduces classification error in automated STN localization from MERs. Additional improvement from patient-specific normalization suggests these approaches are necessary for clinically reliable automation of MER interpretation.SignificanceThese findings represent an incremental advance in automated functional localization of STN from MER in DBS surgery.
Journal: Clinical Neurophysiology - Volume 126, Issue 5, May 2015, Pages 975–982