کد مقاله | کد نشریه | سال انتشار | مقاله انگلیسی | نسخه تمام متن |
---|---|---|---|---|
379179 | 659272 | 2009 | 9 صفحه PDF | دانلود رایگان |
عنوان انگلیسی مقاله ISI
An optimization of ReliefF for classification in large datasets
دانلود مقاله + سفارش ترجمه
دانلود مقاله ISI انگلیسی
رایگان برای ایرانیان
کلمات کلیدی
موضوعات مرتبط
مهندسی و علوم پایه
مهندسی کامپیوتر
هوش مصنوعی
پیش نمایش صفحه اول مقاله
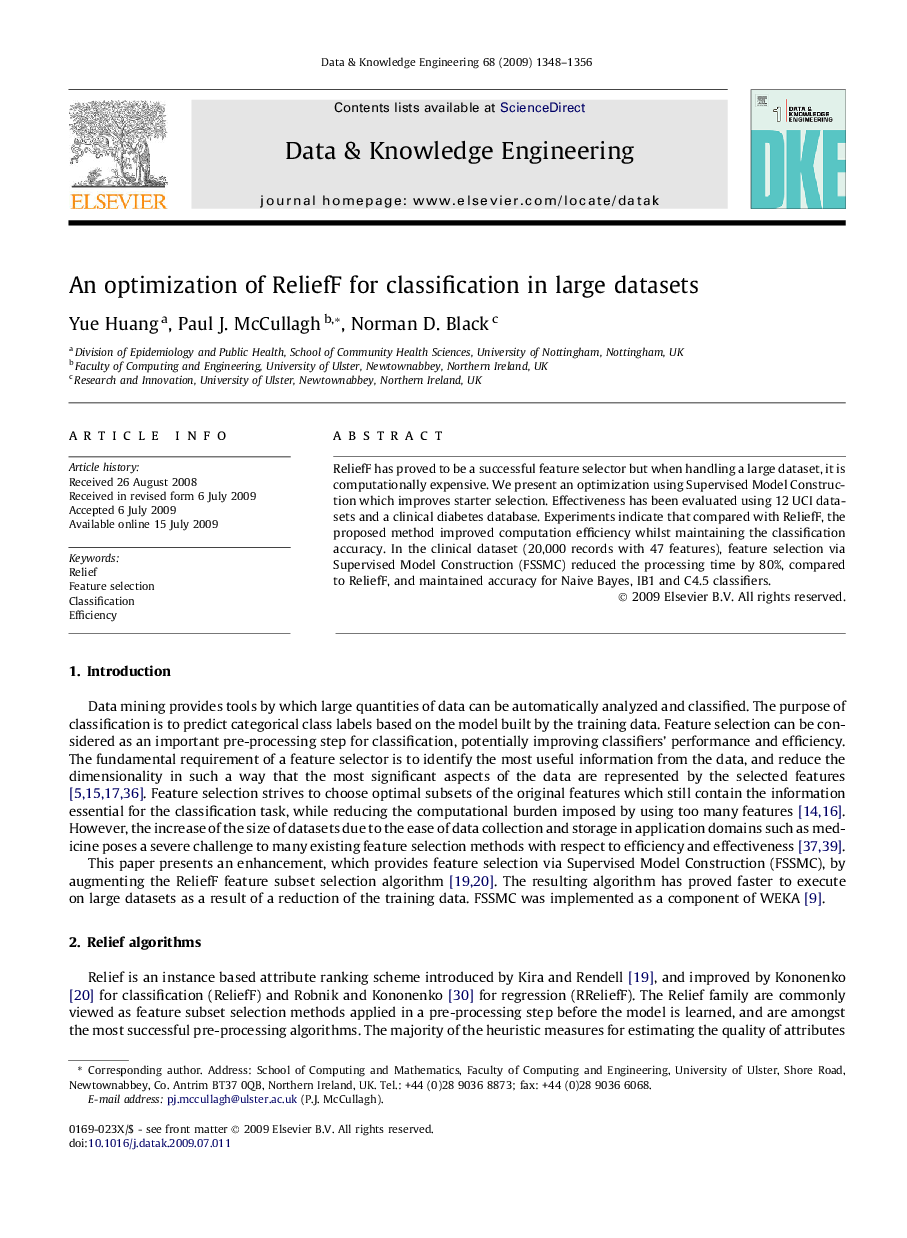
چکیده انگلیسی
ReliefF has proved to be a successful feature selector but when handling a large dataset, it is computationally expensive. We present an optimization using Supervised Model Construction which improves starter selection. Effectiveness has been evaluated using 12 UCI datasets and a clinical diabetes database. Experiments indicate that compared with ReliefF, the proposed method improved computation efficiency whilst maintaining the classification accuracy. In the clinical dataset (20,000 records with 47 features), feature selection via Supervised Model Construction (FSSMC) reduced the processing time by 80%, compared to ReliefF, and maintained accuracy for Naive Bayes, IB1 and C4.5 classifiers.
ناشر
Database: Elsevier - ScienceDirect (ساینس دایرکت)
Journal: Data & Knowledge Engineering - Volume 68, Issue 11, November 2009, Pages 1348–1356
Journal: Data & Knowledge Engineering - Volume 68, Issue 11, November 2009, Pages 1348–1356
نویسندگان
Yue Huang, Paul J. McCullagh, Norman D. Black,