کد مقاله | کد نشریه | سال انتشار | مقاله انگلیسی | نسخه تمام متن |
---|---|---|---|---|
382097 | 660729 | 2015 | 14 صفحه PDF | دانلود رایگان |
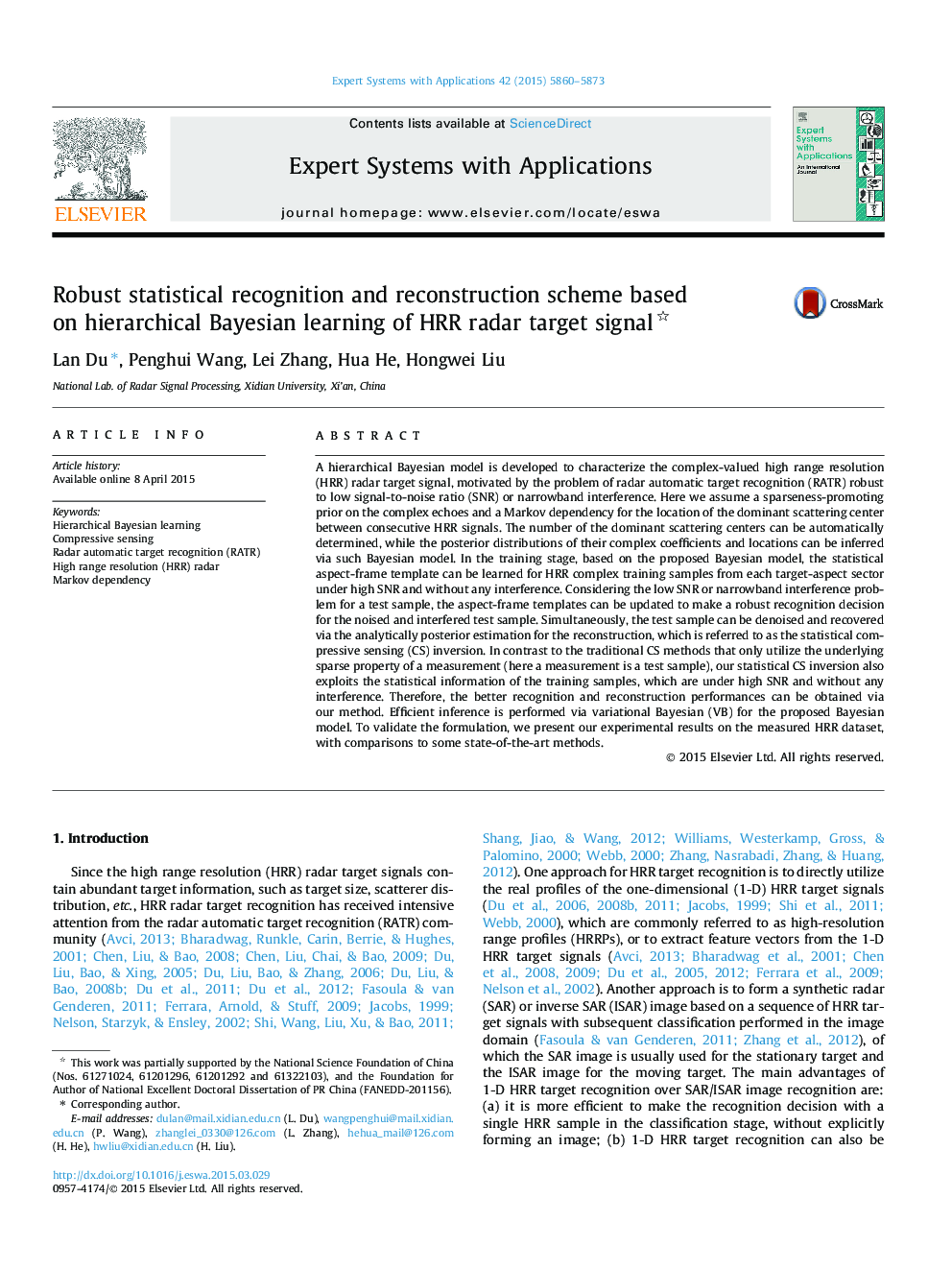
• We develop a Bayesian model for complex data of high range resolution (HRR) radar.
• A recognition scheme robust to noise and narrowband interference is proposed.
• A statistical compressive sensing inversion is derived for recovery of HRR data.
• Efficient inference is performed via variational Bayesian.
• Experimental results show our methods perform better than some existing methods.
A hierarchical Bayesian model is developed to characterize the complex-valued high range resolution (HRR) radar target signal, motivated by the problem of radar automatic target recognition (RATR) robust to low signal-to-noise ratio (SNR) or narrowband interference. Here we assume a sparseness-promoting prior on the complex echoes and a Markov dependency for the location of the dominant scattering center between consecutive HRR signals. The number of the dominant scattering centers can be automatically determined, while the posterior distributions of their complex coefficients and locations can be inferred via such Bayesian model. In the training stage, based on the proposed Bayesian model, the statistical aspect-frame template can be learned for HRR complex training samples from each target-aspect sector under high SNR and without any interference. Considering the low SNR or narrowband interference problem for a test sample, the aspect-frame templates can be updated to make a robust recognition decision for the noised and interfered test sample. Simultaneously, the test sample can be denoised and recovered via the analytically posterior estimation for the reconstruction, which is referred to as the statistical compressive sensing (CS) inversion. In contrast to the traditional CS methods that only utilize the underlying sparse property of a measurement (here a measurement is a test sample), our statistical CS inversion also exploits the statistical information of the training samples, which are under high SNR and without any interference. Therefore, the better recognition and reconstruction performances can be obtained via our method. Efficient inference is performed via variational Bayesian (VB) for the proposed Bayesian model. To validate the formulation, we present our experimental results on the measured HRR dataset, with comparisons to some state-of-the-art methods.
Journal: Expert Systems with Applications - Volume 42, Issue 14, 15 August 2015, Pages 5860–5873