کد مقاله | کد نشریه | سال انتشار | مقاله انگلیسی | نسخه تمام متن |
---|---|---|---|---|
384363 | 660846 | 2012 | 11 صفحه PDF | دانلود رایگان |
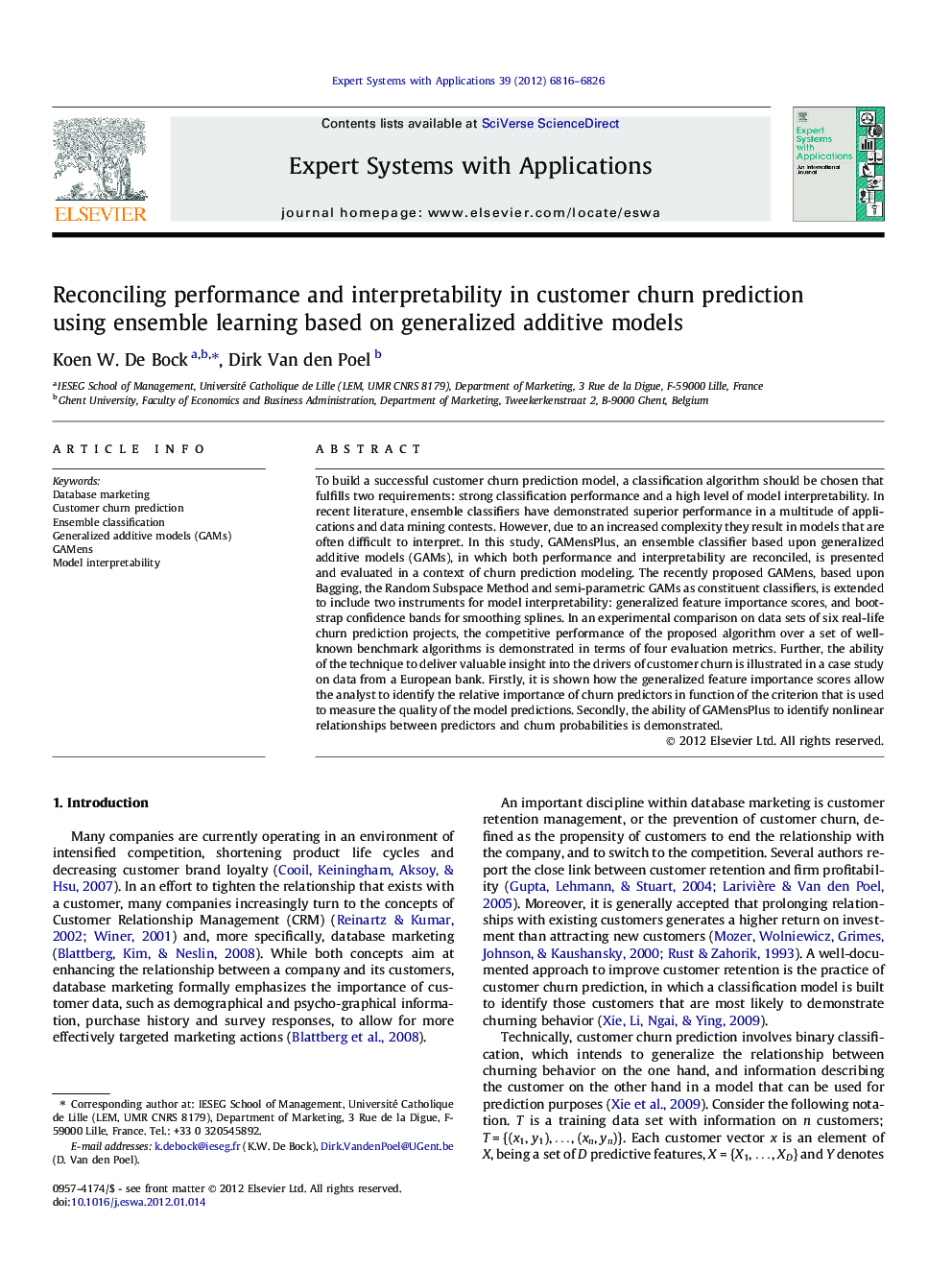
To build a successful customer churn prediction model, a classification algorithm should be chosen that fulfills two requirements: strong classification performance and a high level of model interpretability. In recent literature, ensemble classifiers have demonstrated superior performance in a multitude of applications and data mining contests. However, due to an increased complexity they result in models that are often difficult to interpret. In this study, GAMensPlus, an ensemble classifier based upon generalized additive models (GAMs), in which both performance and interpretability are reconciled, is presented and evaluated in a context of churn prediction modeling. The recently proposed GAMens, based upon Bagging, the Random Subspace Method and semi-parametric GAMs as constituent classifiers, is extended to include two instruments for model interpretability: generalized feature importance scores, and bootstrap confidence bands for smoothing splines. In an experimental comparison on data sets of six real-life churn prediction projects, the competitive performance of the proposed algorithm over a set of well-known benchmark algorithms is demonstrated in terms of four evaluation metrics. Further, the ability of the technique to deliver valuable insight into the drivers of customer churn is illustrated in a case study on data from a European bank. Firstly, it is shown how the generalized feature importance scores allow the analyst to identify the relative importance of churn predictors in function of the criterion that is used to measure the quality of the model predictions. Secondly, the ability of GAMensPlus to identify nonlinear relationships between predictors and churn probabilities is demonstrated.
Highlight
► Ensemble classification based on GAMs is introduced to customer churn prediction.
► Bagging and the Random Subspace Method combine semi-parametric additive logits.
► The algorithm is extended with two techniques to make models comprehensible.
► Experiments demonstrate superior predictions and enhanced interpretability.
Journal: Expert Systems with Applications - Volume 39, Issue 8, 15 June 2012, Pages 6816–6826