کد مقاله | کد نشریه | سال انتشار | مقاله انگلیسی | نسخه تمام متن |
---|---|---|---|---|
385113 | 660860 | 2011 | 8 صفحه PDF | دانلود رایگان |
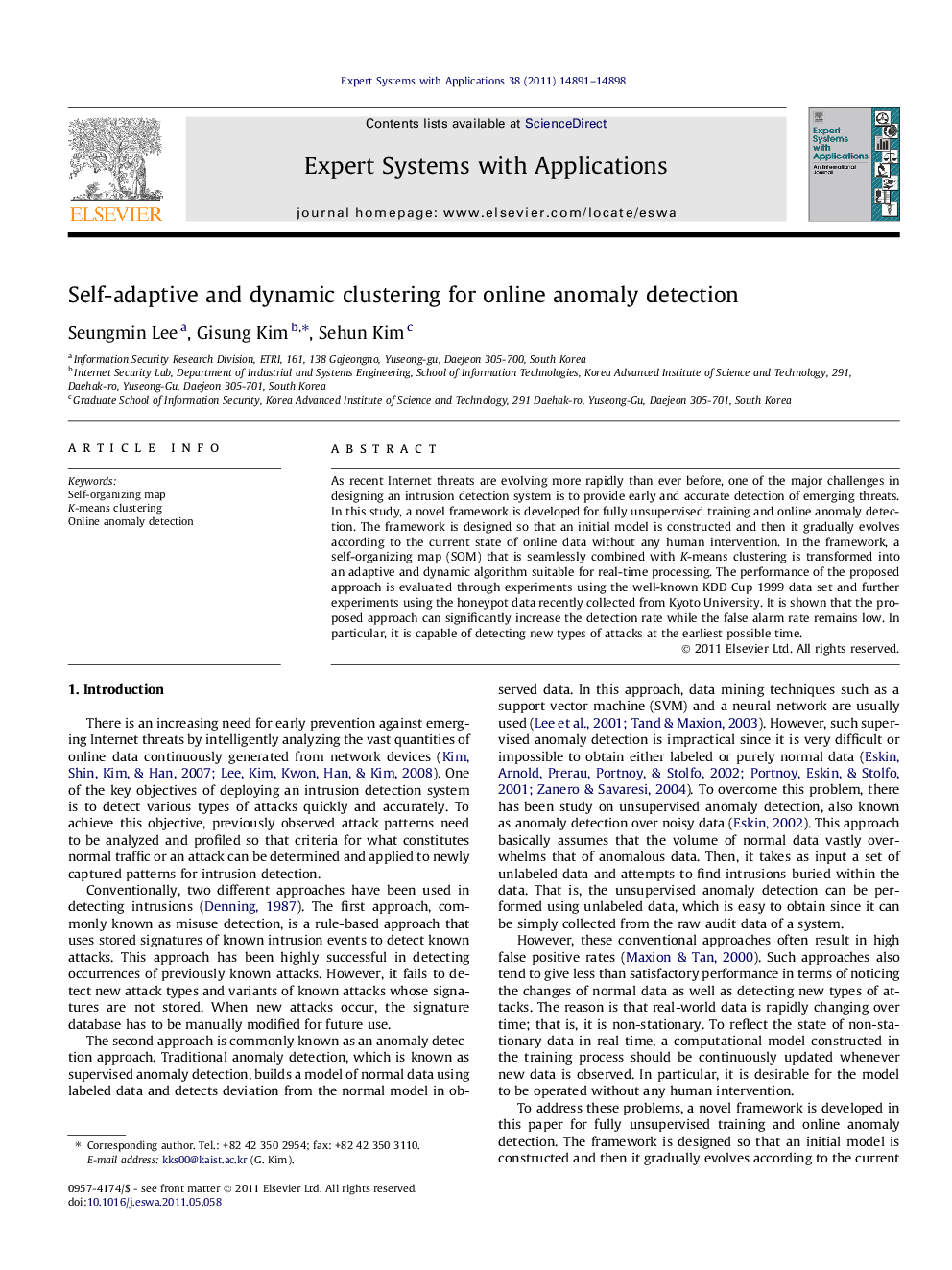
As recent Internet threats are evolving more rapidly than ever before, one of the major challenges in designing an intrusion detection system is to provide early and accurate detection of emerging threats. In this study, a novel framework is developed for fully unsupervised training and online anomaly detection. The framework is designed so that an initial model is constructed and then it gradually evolves according to the current state of online data without any human intervention. In the framework, a self-organizing map (SOM) that is seamlessly combined with K-means clustering is transformed into an adaptive and dynamic algorithm suitable for real-time processing. The performance of the proposed approach is evaluated through experiments using the well-known KDD Cup 1999 data set and further experiments using the honeypot data recently collected from Kyoto University. It is shown that the proposed approach can significantly increase the detection rate while the false alarm rate remains low. In particular, it is capable of detecting new types of attacks at the earliest possible time.
► We develop an online anomaly detection model based on unsupervised learning.
► The model gradually evolves according to online data without human intervention.
► Our adaptive and dynamic model is suitable for real-time processing.
► The model is capable of detecting new types of attacks at the earliest possible time.
Journal: Expert Systems with Applications - Volume 38, Issue 12, November–December 2011, Pages 14891–14898