کد مقاله | کد نشریه | سال انتشار | مقاله انگلیسی | نسخه تمام متن |
---|---|---|---|---|
390524 | 661265 | 2009 | 14 صفحه PDF | دانلود رایگان |
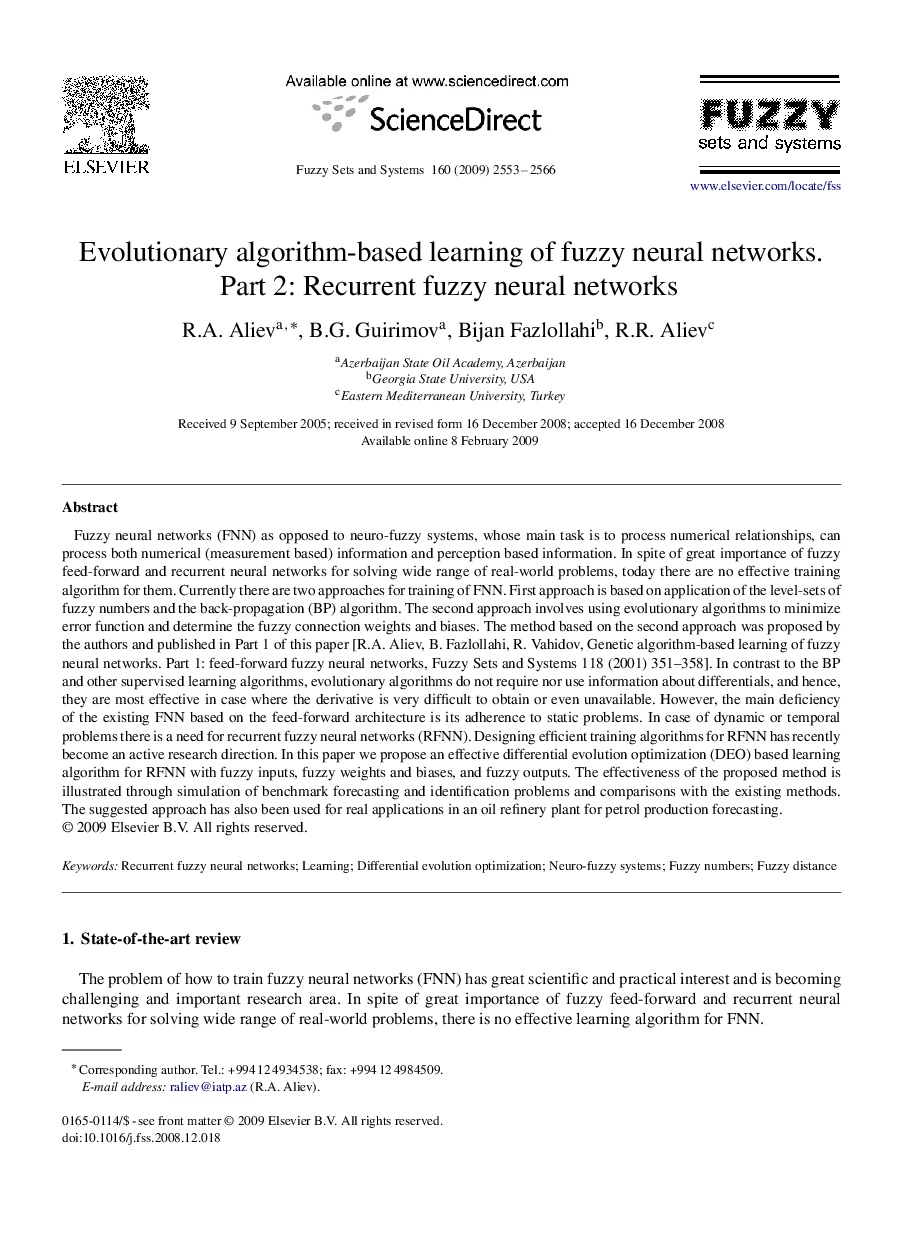
Fuzzy neural networks (FNN) as opposed to neuro-fuzzy systems, whose main task is to process numerical relationships, can process both numerical (measurement based) information and perception based information. In spite of great importance of fuzzy feed-forward and recurrent neural networks for solving wide range of real-world problems, today there are no effective training algorithm for them. Currently there are two approaches for training of FNN. First approach is based on application of the level-sets of fuzzy numbers and the back-propagation (BP) algorithm. The second approach involves using evolutionary algorithms to minimize error function and determine the fuzzy connection weights and biases. The method based on the second approach was proposed by the authors and published in Part 1 of this paper [R.A. Aliev, B. Fazlollahi, R. Vahidov, Genetic algorithm-based learning of fuzzy neural networks. Part 1: feed-forward fuzzy neural networks, Fuzzy Sets and Systems 118 (2001) 351–358]. In contrast to the BP and other supervised learning algorithms, evolutionary algorithms do not require nor use information about differentials, and hence, they are most effective in case where the derivative is very difficult to obtain or even unavailable. However, the main deficiency of the existing FNN based on the feed-forward architecture is its adherence to static problems. In case of dynamic or temporal problems there is a need for recurrent fuzzy neural networks (RFNN). Designing efficient training algorithms for RFNN has recently become an active research direction. In this paper we propose an effective differential evolution optimization (DEO) based learning algorithm for RFNN with fuzzy inputs, fuzzy weights and biases, and fuzzy outputs. The effectiveness of the proposed method is illustrated through simulation of benchmark forecasting and identification problems and comparisons with the existing methods. The suggested approach has also been used for real applications in an oil refinery plant for petrol production forecasting.
Journal: Fuzzy Sets and Systems - Volume 160, Issue 17, 1 September 2009, Pages 2553-2566