کد مقاله | کد نشریه | سال انتشار | مقاله انگلیسی | نسخه تمام متن |
---|---|---|---|---|
397893 | 1438455 | 2014 | 22 صفحه PDF | دانلود رایگان |
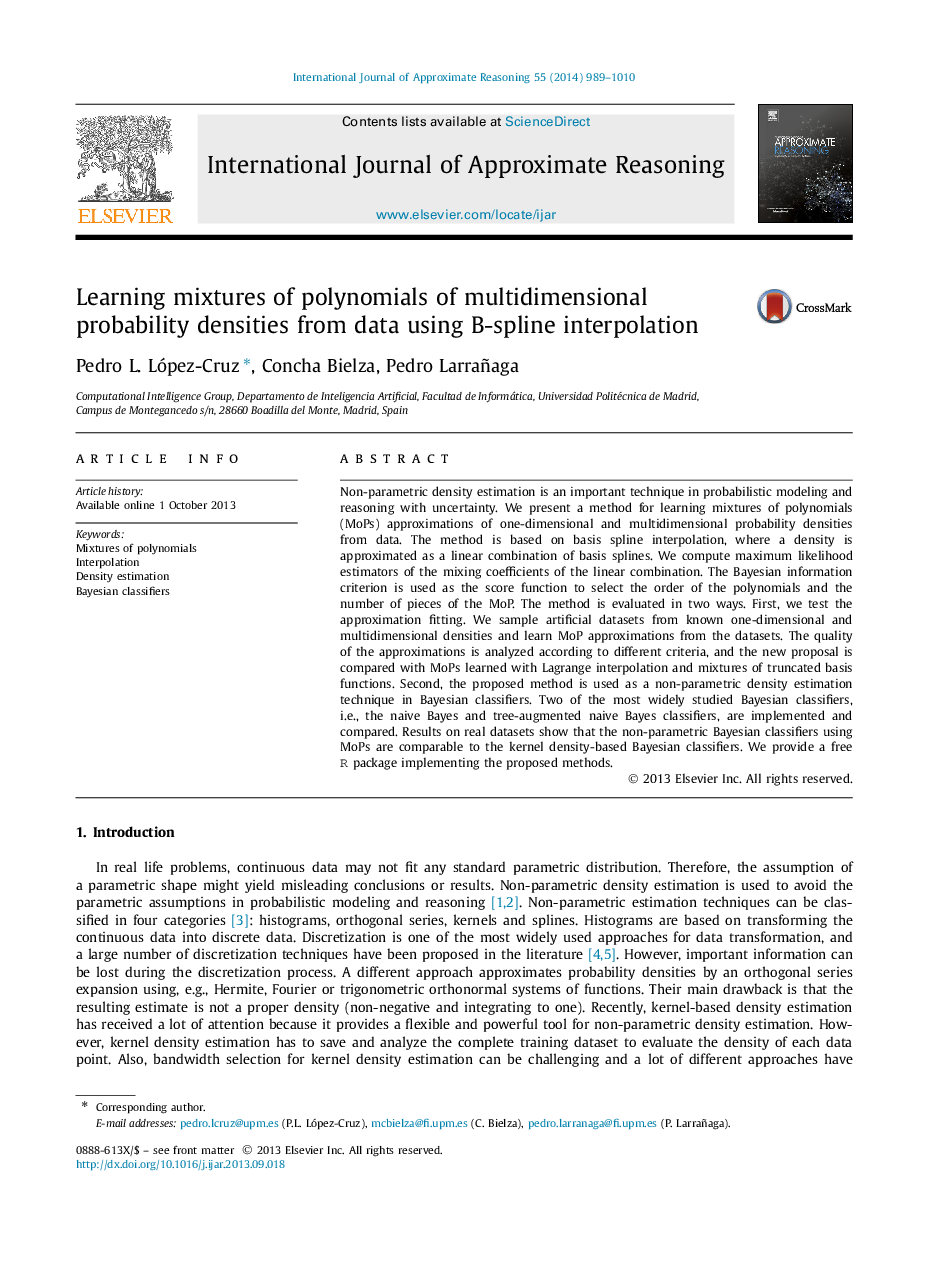
• We propose a method for learning mixtures of polynomials from data.
• We estimate one-dimensional and multidimensional probability densities.
• We build non-parametric Bayesian classifiers, yielding competitive accuracies.
• We implement the methods in an R package.
Non-parametric density estimation is an important technique in probabilistic modeling and reasoning with uncertainty. We present a method for learning mixtures of polynomials (MoPs) approximations of one-dimensional and multidimensional probability densities from data. The method is based on basis spline interpolation, where a density is approximated as a linear combination of basis splines. We compute maximum likelihood estimators of the mixing coefficients of the linear combination. The Bayesian information criterion is used as the score function to select the order of the polynomials and the number of pieces of the MoP. The method is evaluated in two ways. First, we test the approximation fitting. We sample artificial datasets from known one-dimensional and multidimensional densities and learn MoP approximations from the datasets. The quality of the approximations is analyzed according to different criteria, and the new proposal is compared with MoPs learned with Lagrange interpolation and mixtures of truncated basis functions. Second, the proposed method is used as a non-parametric density estimation technique in Bayesian classifiers. Two of the most widely studied Bayesian classifiers, i.e., the naive Bayes and tree-augmented naive Bayes classifiers, are implemented and compared. Results on real datasets show that the non-parametric Bayesian classifiers using MoPs are comparable to the kernel density-based Bayesian classifiers. We provide a free R package implementing the proposed methods.
Journal: International Journal of Approximate Reasoning - Volume 55, Issue 4, June 2014, Pages 989–1010