کد مقاله | کد نشریه | سال انتشار | مقاله انگلیسی | نسخه تمام متن |
---|---|---|---|---|
397924 | 1438481 | 2011 | 15 صفحه PDF | دانلود رایگان |
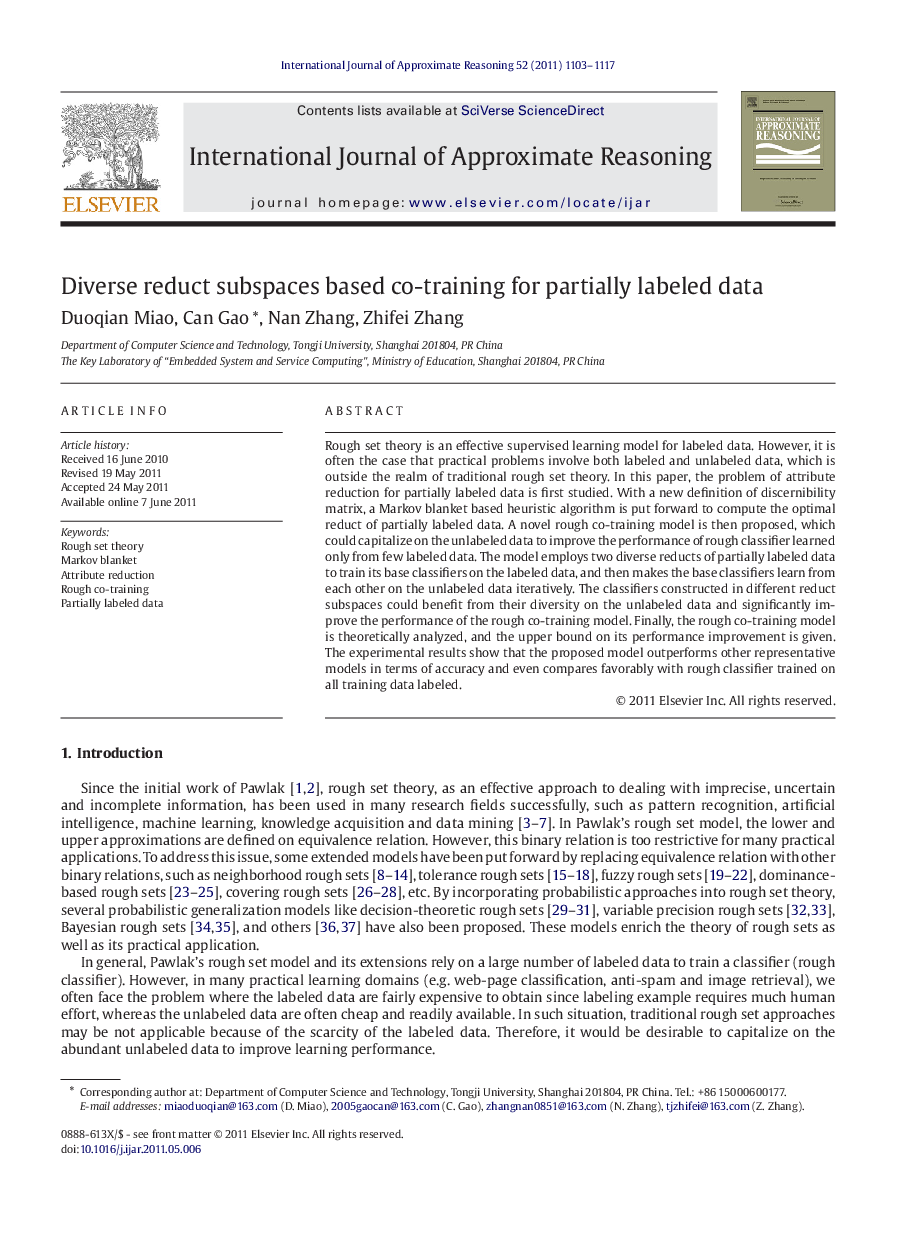
Rough set theory is an effective supervised learning model for labeled data. However, it is often the case that practical problems involve both labeled and unlabeled data, which is outside the realm of traditional rough set theory. In this paper, the problem of attribute reduction for partially labeled data is first studied. With a new definition of discernibility matrix, a Markov blanket based heuristic algorithm is put forward to compute the optimal reduct of partially labeled data. A novel rough co-training model is then proposed, which could capitalize on the unlabeled data to improve the performance of rough classifier learned only from few labeled data. The model employs two diverse reducts of partially labeled data to train its base classifiers on the labeled data, and then makes the base classifiers learn from each other on the unlabeled data iteratively. The classifiers constructed in different reduct subspaces could benefit from their diversity on the unlabeled data and significantly improve the performance of the rough co-training model. Finally, the rough co-training model is theoretically analyzed, and the upper bound on its performance improvement is given. The experimental results show that the proposed model outperforms other representative models in terms of accuracy and even compares favorably with rough classifier trained on all training data labeled.
► A new discernibility matrix is proposed for partially labeled data.
► Markov blanket theory is introduced to conduct the discernibility matrix based semi-supervised attribute reduction algorithm.
► A novel rough co-training model is put forward to deal with the problem of classification of partially labeled data.
► The upper bound on the performance improvement of the proposed model is given.
► Some salient features of the proposed model are showed in the experiments.
Journal: International Journal of Approximate Reasoning - Volume 52, Issue 8, November 2011, Pages 1103–1117