کد مقاله | کد نشریه | سال انتشار | مقاله انگلیسی | نسخه تمام متن |
---|---|---|---|---|
404362 | 677415 | 2011 | 14 صفحه PDF | دانلود رایگان |
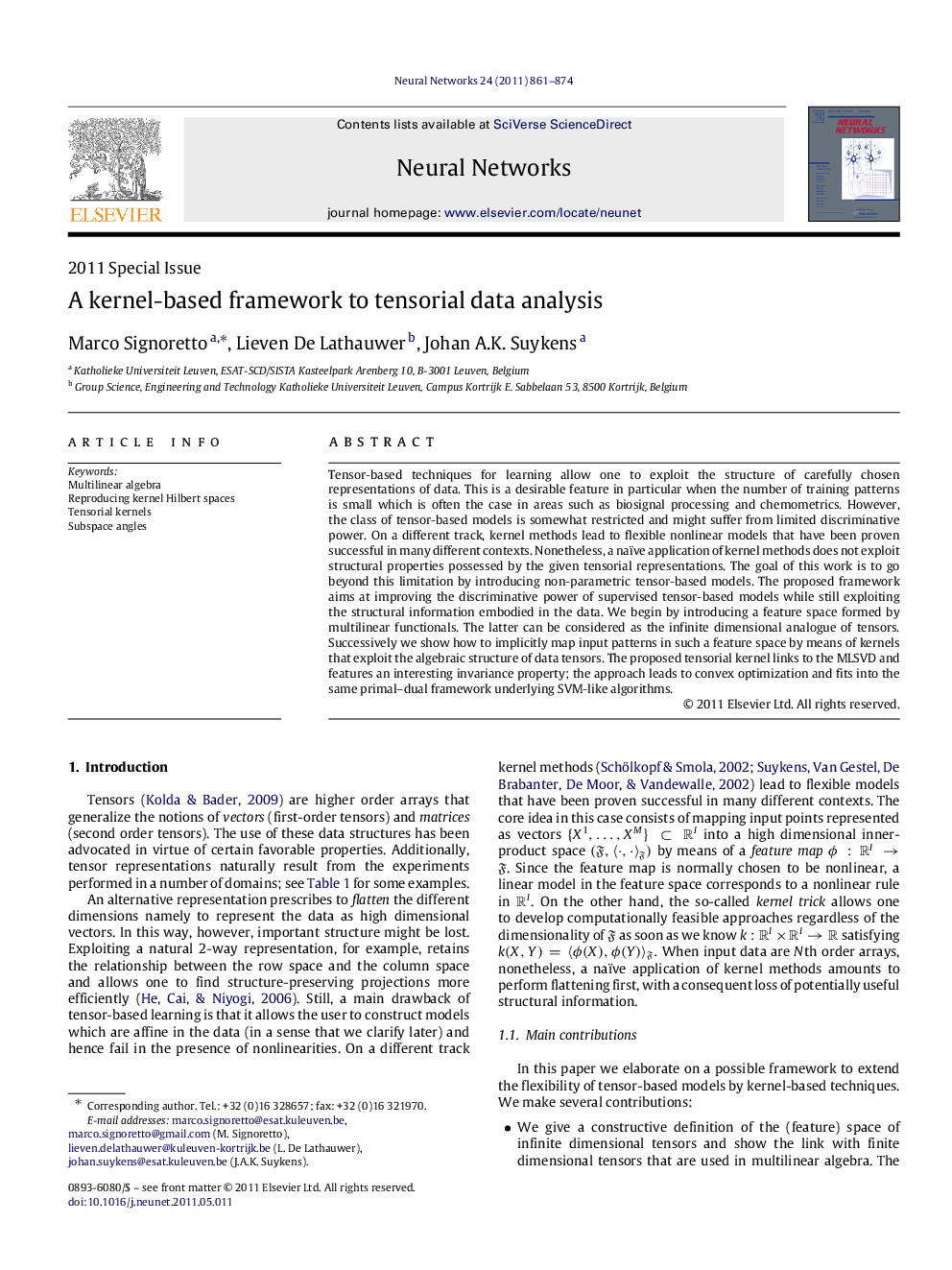
Tensor-based techniques for learning allow one to exploit the structure of carefully chosen representations of data. This is a desirable feature in particular when the number of training patterns is small which is often the case in areas such as biosignal processing and chemometrics. However, the class of tensor-based models is somewhat restricted and might suffer from limited discriminative power. On a different track, kernel methods lead to flexible nonlinear models that have been proven successful in many different contexts. Nonetheless, a naïve application of kernel methods does not exploit structural properties possessed by the given tensorial representations. The goal of this work is to go beyond this limitation by introducing non-parametric tensor-based models. The proposed framework aims at improving the discriminative power of supervised tensor-based models while still exploiting the structural information embodied in the data. We begin by introducing a feature space formed by multilinear functionals. The latter can be considered as the infinite dimensional analogue of tensors. Successively we show how to implicitly map input patterns in such a feature space by means of kernels that exploit the algebraic structure of data tensors. The proposed tensorial kernel links to the MLSVD and features an interesting invariance property; the approach leads to convex optimization and fits into the same primal–dual framework underlying SVM-like algorithms.
► Multilinear algebra and kernels: feature space of infinite dimensional tensors.
► Our formalism give rise to product kernels, Gaussian-RBF as a special case.
► All purpose kernels do not capture the topology of tensorial representations.
► We introduce structure-preserving product kernels for tensors.
► We study the invariance fulfilled by the proposed kernels and show their relevance for pattern recognition.
Journal: Neural Networks - Volume 24, Issue 8, October 2011, Pages 861–874