کد مقاله | کد نشریه | سال انتشار | مقاله انگلیسی | نسخه تمام متن |
---|---|---|---|---|
407257 | 678134 | 2010 | 5 صفحه PDF | دانلود رایگان |
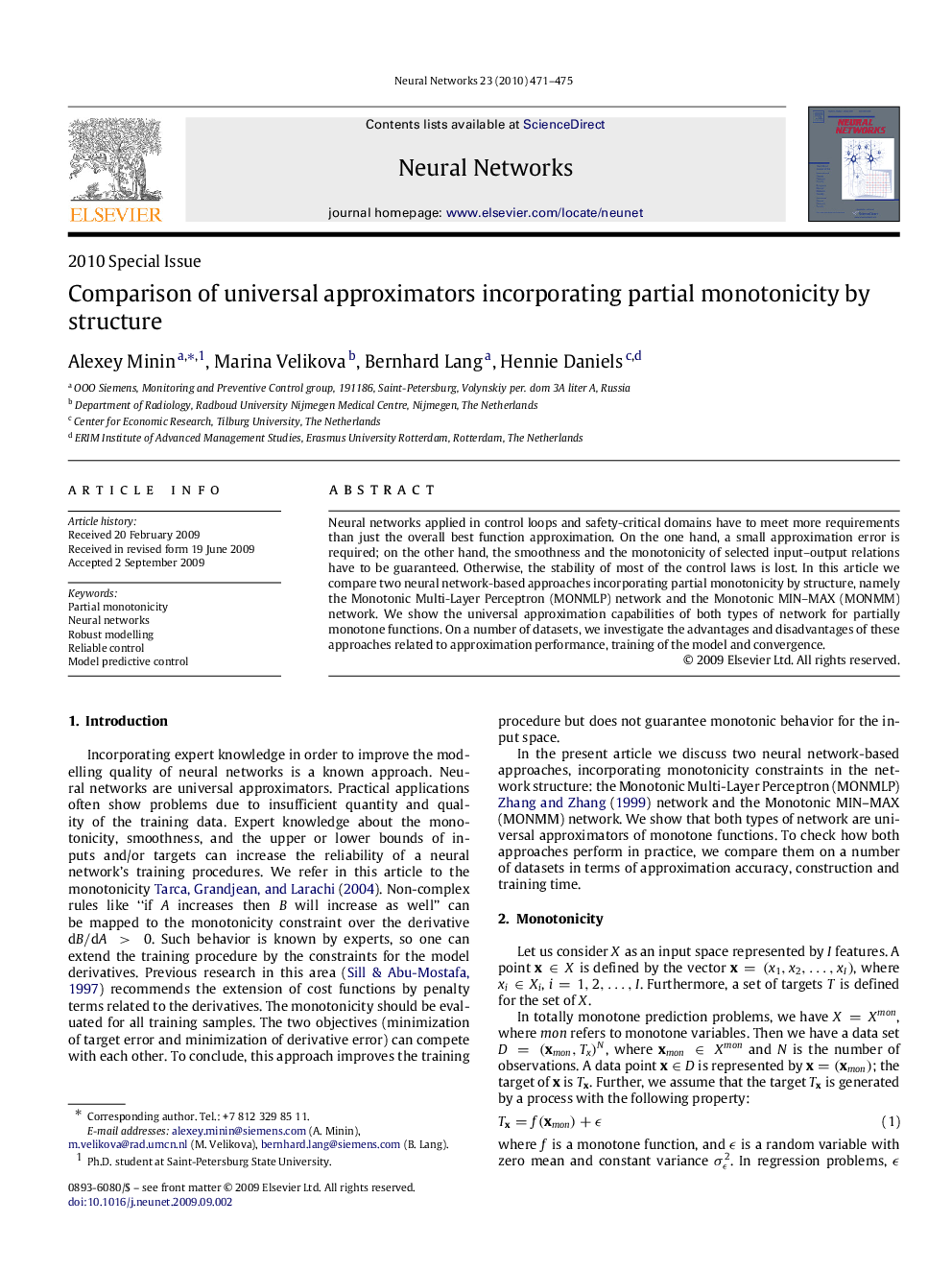
Neural networks applied in control loops and safety-critical domains have to meet more requirements than just the overall best function approximation. On the one hand, a small approximation error is required; on the other hand, the smoothness and the monotonicity of selected input–output relations have to be guaranteed. Otherwise, the stability of most of the control laws is lost. In this article we compare two neural network-based approaches incorporating partial monotonicity by structure, namely the Monotonic Multi-Layer Perceptron (MONMLP) network and the Monotonic MIN–MAX (MONMM) network. We show the universal approximation capabilities of both types of network for partially monotone functions. On a number of datasets, we investigate the advantages and disadvantages of these approaches related to approximation performance, training of the model and convergence.
Journal: Neural Networks - Volume 23, Issue 4, May 2010, Pages 471–475