کد مقاله | کد نشریه | سال انتشار | مقاله انگلیسی | نسخه تمام متن |
---|---|---|---|---|
408230 | 679010 | 2011 | 9 صفحه PDF | دانلود رایگان |
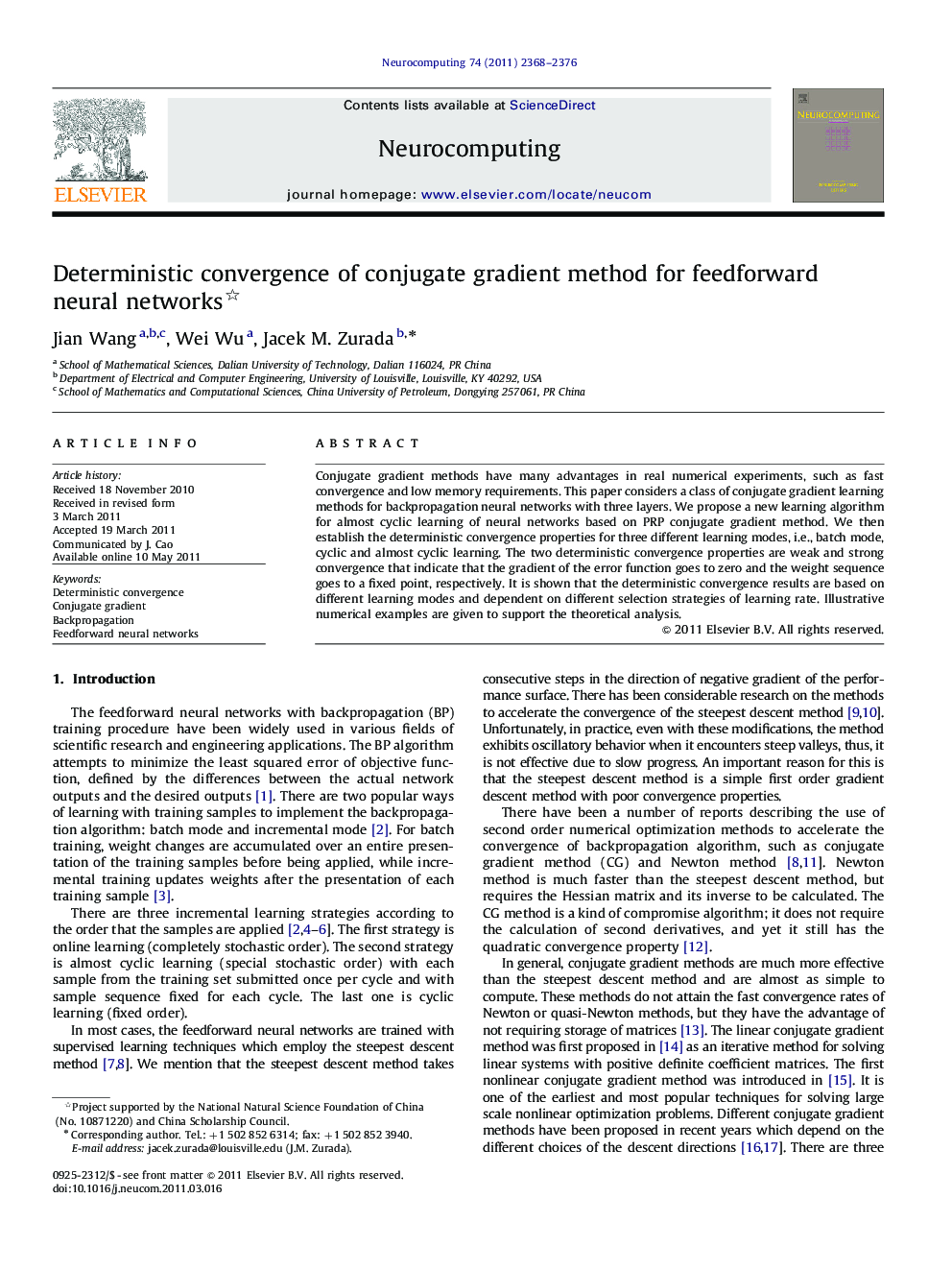
Conjugate gradient methods have many advantages in real numerical experiments, such as fast convergence and low memory requirements. This paper considers a class of conjugate gradient learning methods for backpropagation neural networks with three layers. We propose a new learning algorithm for almost cyclic learning of neural networks based on PRP conjugate gradient method. We then establish the deterministic convergence properties for three different learning modes, i.e., batch mode, cyclic and almost cyclic learning. The two deterministic convergence properties are weak and strong convergence that indicate that the gradient of the error function goes to zero and the weight sequence goes to a fixed point, respectively. It is shown that the deterministic convergence results are based on different learning modes and dependent on different selection strategies of learning rate. Illustrative numerical examples are given to support the theoretical analysis.
Journal: Neurocomputing - Volume 74, Issues 14–15, July 2011, Pages 2368–2376