کد مقاله | کد نشریه | سال انتشار | مقاله انگلیسی | نسخه تمام متن |
---|---|---|---|---|
409133 | 679057 | 2008 | 9 صفحه PDF | دانلود رایگان |
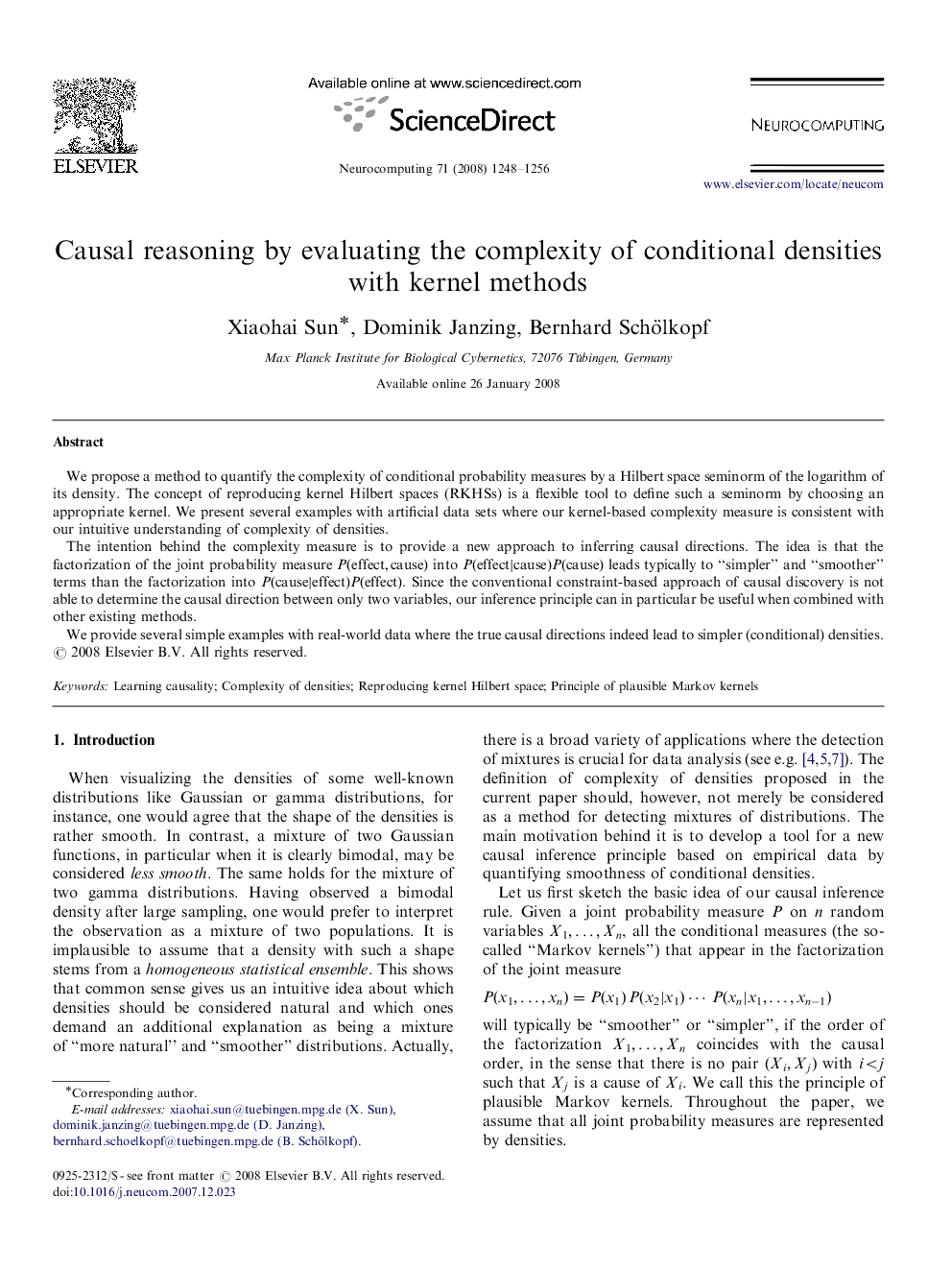
We propose a method to quantify the complexity of conditional probability measures by a Hilbert space seminorm of the logarithm of its density. The concept of reproducing kernel Hilbert spaces (RKHSs) is a flexible tool to define such a seminorm by choosing an appropriate kernel. We present several examples with artificial data sets where our kernel-based complexity measure is consistent with our intuitive understanding of complexity of densities.The intention behind the complexity measure is to provide a new approach to inferring causal directions. The idea is that the factorization of the joint probability measure P(effect,cause)P(effect,cause) into P(effect|cause)P(cause)P(effect|cause)P(cause) leads typically to “simpler” and “smoother” terms than the factorization into P(cause|effect)P(effect)P(cause|effect)P(effect). Since the conventional constraint-based approach of causal discovery is not able to determine the causal direction between only two variables, our inference principle can in particular be useful when combined with other existing methods.We provide several simple examples with real-world data where the true causal directions indeed lead to simpler (conditional) densities.
Journal: Neurocomputing - Volume 71, Issues 7–9, March 2008, Pages 1248–1256