کد مقاله | کد نشریه | سال انتشار | مقاله انگلیسی | نسخه تمام متن |
---|---|---|---|---|
409265 | 679062 | 2008 | 14 صفحه PDF | دانلود رایگان |
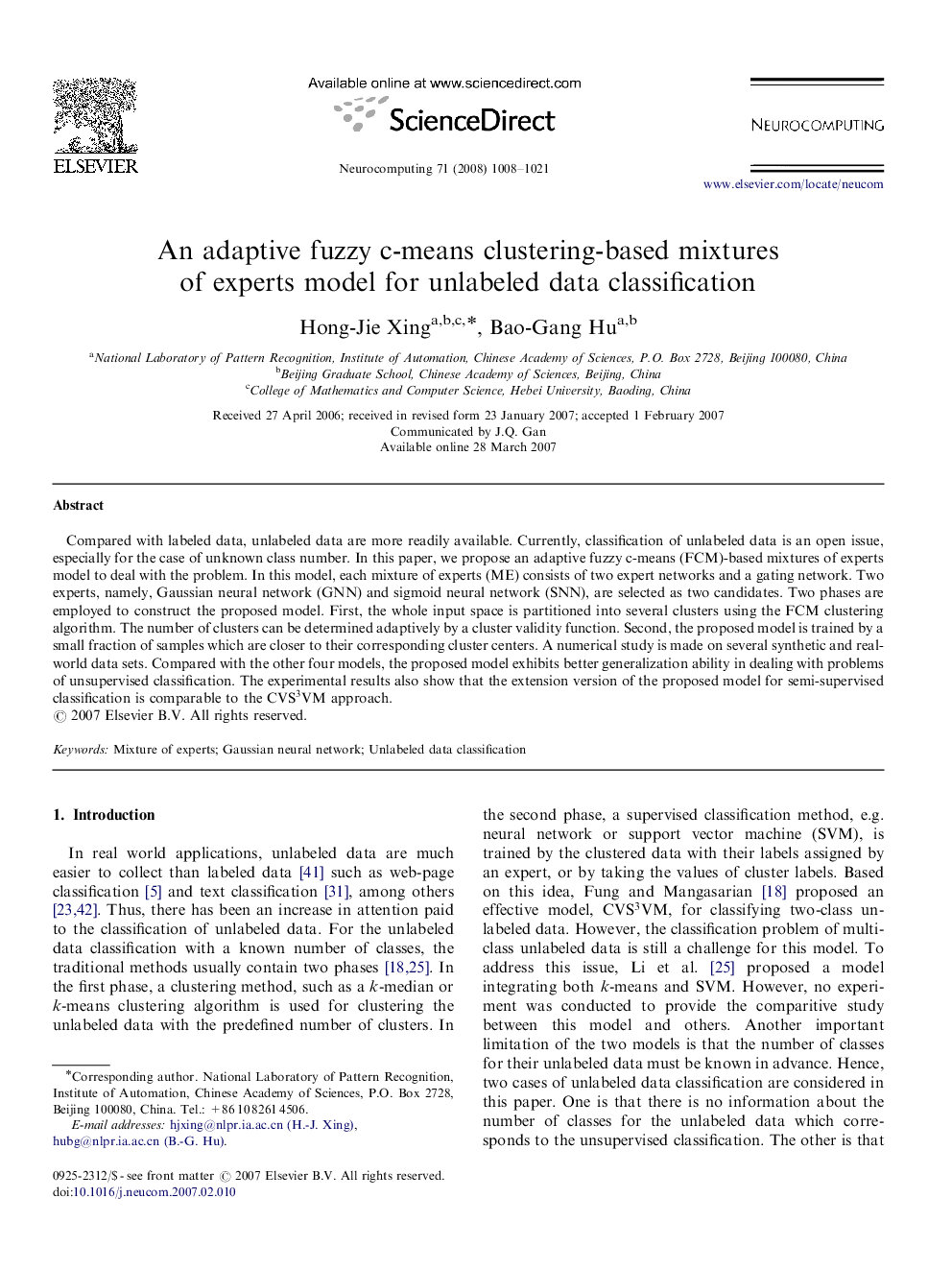
Compared with labeled data, unlabeled data are more readily available. Currently, classification of unlabeled data is an open issue, especially for the case of unknown class number. In this paper, we propose an adaptive fuzzy c-means (FCM)-based mixtures of experts model to deal with the problem. In this model, each mixture of experts (ME) consists of two expert networks and a gating network. Two experts, namely, Gaussian neural network (GNN) and sigmoid neural network (SNN), are selected as two candidates. Two phases are employed to construct the proposed model. First, the whole input space is partitioned into several clusters using the FCM clustering algorithm. The number of clusters can be determined adaptively by a cluster validity function. Second, the proposed model is trained by a small fraction of samples which are closer to their corresponding cluster centers. A numerical study is made on several synthetic and real-world data sets. Compared with the other four models, the proposed model exhibits better generalization ability in dealing with problems of unsupervised classification. The experimental results also show that the extension version of the proposed model for semi-supervised classification is comparable to the CVS3VMCVS3VM approach.
Journal: Neurocomputing - Volume 71, Issues 4–6, January 2008, Pages 1008–1021