کد مقاله | کد نشریه | سال انتشار | مقاله انگلیسی | نسخه تمام متن |
---|---|---|---|---|
410306 | 679137 | 2013 | 7 صفحه PDF | دانلود رایگان |
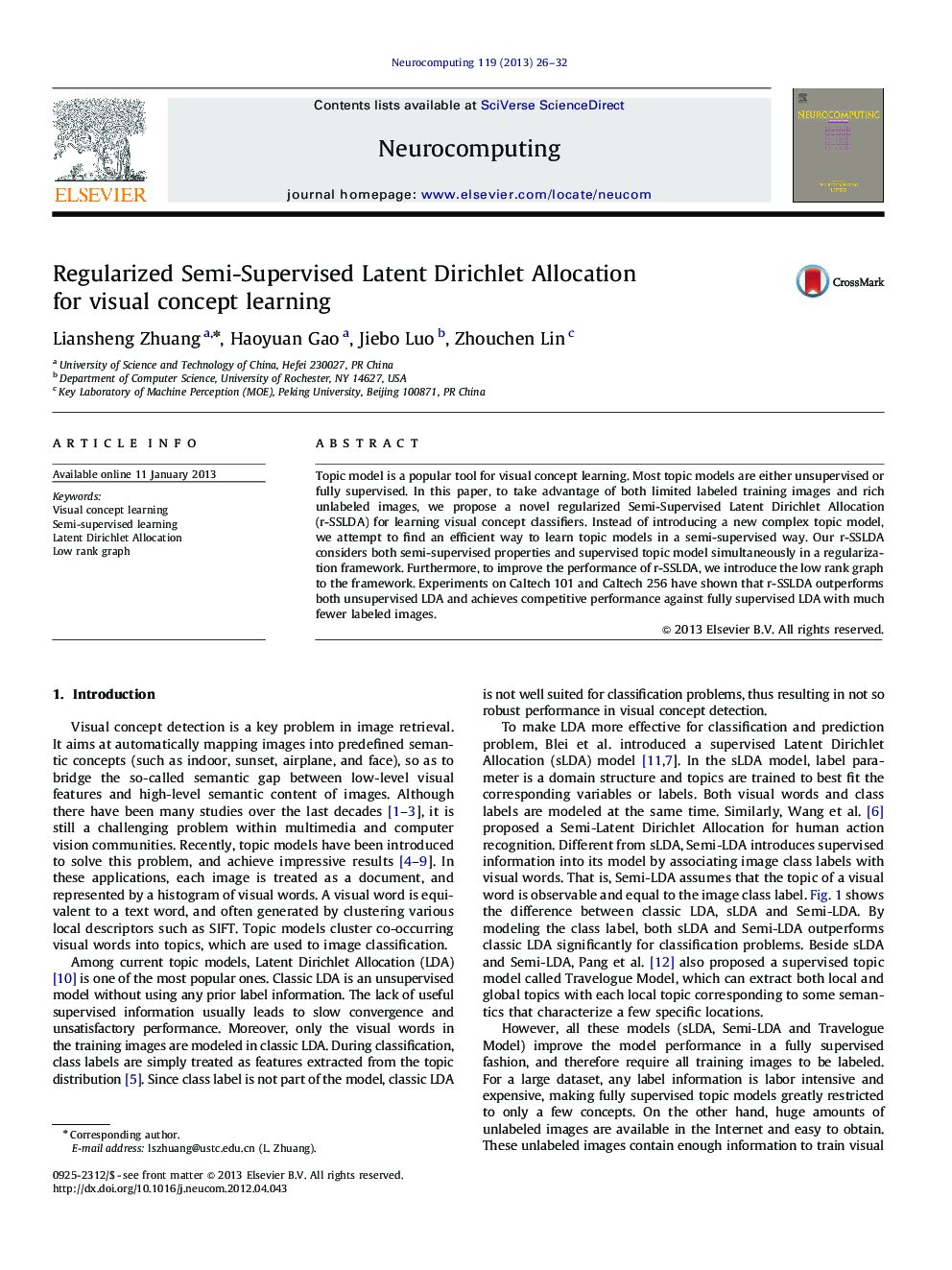
Topic model is a popular tool for visual concept learning. Most topic models are either unsupervised or fully supervised. In this paper, to take advantage of both limited labeled training images and rich unlabeled images, we propose a novel regularized Semi-Supervised Latent Dirichlet Allocation (r-SSLDA) for learning visual concept classifiers. Instead of introducing a new complex topic model, we attempt to find an efficient way to learn topic models in a semi-supervised way. Our r-SSLDA considers both semi-supervised properties and supervised topic model simultaneously in a regularization framework. Furthermore, to improve the performance of r-SSLDA, we introduce the low rank graph to the framework. Experiments on Caltech 101 and Caltech 256 have shown that r-SSLDA outperforms both unsupervised LDA and achieves competitive performance against fully supervised LDA with much fewer labeled images.
Journal: Neurocomputing - Volume 119, 7 November 2013, Pages 26–32