کد مقاله | کد نشریه | سال انتشار | مقاله انگلیسی | نسخه تمام متن |
---|---|---|---|---|
410334 | 679137 | 2013 | 8 صفحه PDF | دانلود رایگان |
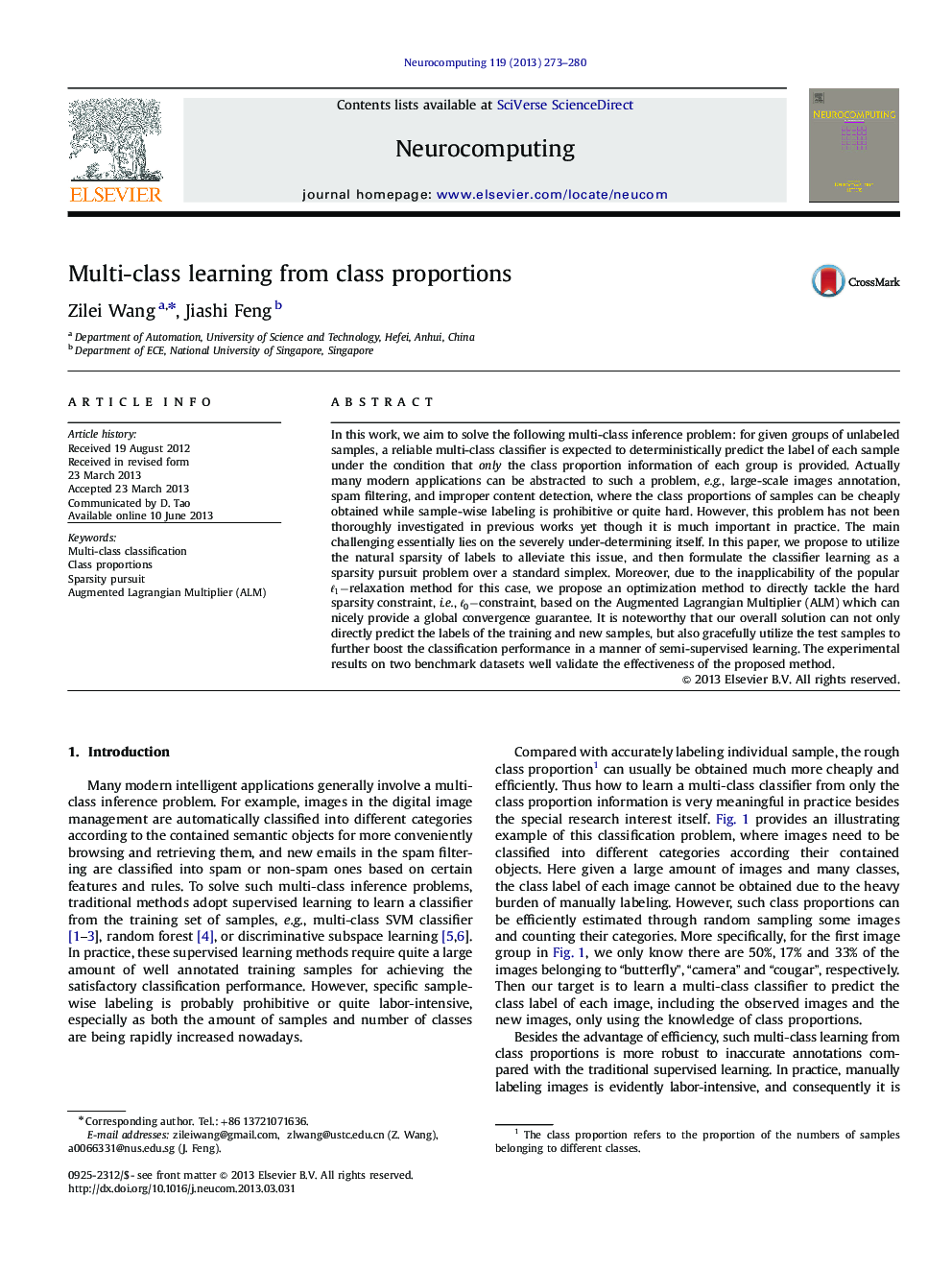
In this work, we aim to solve the following multi-class inference problem: for given groups of unlabeled samples, a reliable multi-class classifier is expected to deterministically predict the label of each sample under the condition that only the class proportion information of each group is provided. Actually many modern applications can be abstracted to such a problem, e.g. , large-scale images annotation, spam filtering, and improper content detection, where the class proportions of samples can be cheaply obtained while sample-wise labeling is prohibitive or quite hard. However, this problem has not been thoroughly investigated in previous works yet though it is much important in practice. The main challenging essentially lies on the severely under-determining itself. In this paper, we propose to utilize the natural sparsity of labels to alleviate this issue, and then formulate the classifier learning as a sparsity pursuit problem over a standard simplex. Moreover, due to the inapplicability of the popular ℓ1-relaxationℓ1-relaxation method for this case, we propose an optimization method to directly tackle the hard sparsity constraint, i.e. , ℓ0-constraintℓ0-constraint, based on the Augmented Lagrangian Multiplier (ALM) which can nicely provide a global convergence guarantee. It is noteworthy that our overall solution can not only directly predict the labels of the training and new samples, but also gracefully utilize the test samples to further boost the classification performance in a manner of semi-supervised learning. The experimental results on two benchmark datasets well validate the effectiveness of the proposed method.
Journal: Neurocomputing - Volume 119, 7 November 2013, Pages 273–280