کد مقاله | کد نشریه | سال انتشار | مقاله انگلیسی | نسخه تمام متن |
---|---|---|---|---|
410350 | 679137 | 2013 | 15 صفحه PDF | دانلود رایگان |
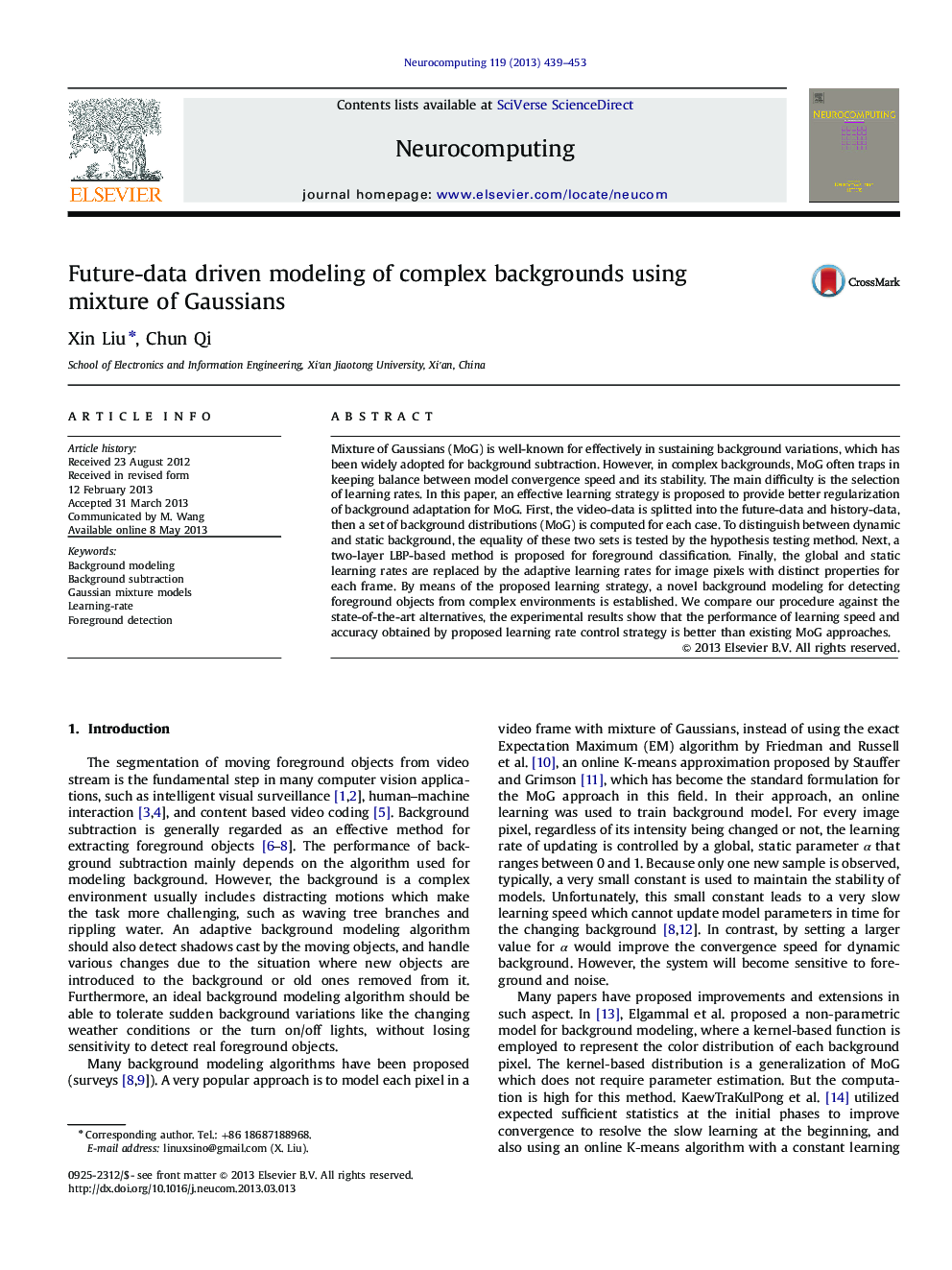
Mixture of Gaussians (MoG) is well-known for effectively in sustaining background variations, which has been widely adopted for background subtraction. However, in complex backgrounds, MoG often traps in keeping balance between model convergence speed and its stability. The main difficulty is the selection of learning rates. In this paper, an effective learning strategy is proposed to provide better regularization of background adaptation for MoG. First, the video-data is splitted into the future-data and history-data, then a set of background distributions (MoG) is computed for each case. To distinguish between dynamic and static background, the equality of these two sets is tested by the hypothesis testing method. Next, a two-layer LBP-based method is proposed for foreground classification. Finally, the global and static learning rates are replaced by the adaptive learning rates for image pixels with distinct properties for each frame. By means of the proposed learning strategy, a novel background modeling for detecting foreground objects from complex environments is established. We compare our procedure against the state-of-the-art alternatives, the experimental results show that the performance of learning speed and accuracy obtained by proposed learning rate control strategy is better than existing MoG approaches.
Journal: Neurocomputing - Volume 119, 7 November 2013, Pages 439–453