کد مقاله | کد نشریه | سال انتشار | مقاله انگلیسی | نسخه تمام متن |
---|---|---|---|---|
411463 | 679563 | 2016 | 8 صفحه PDF | دانلود رایگان |
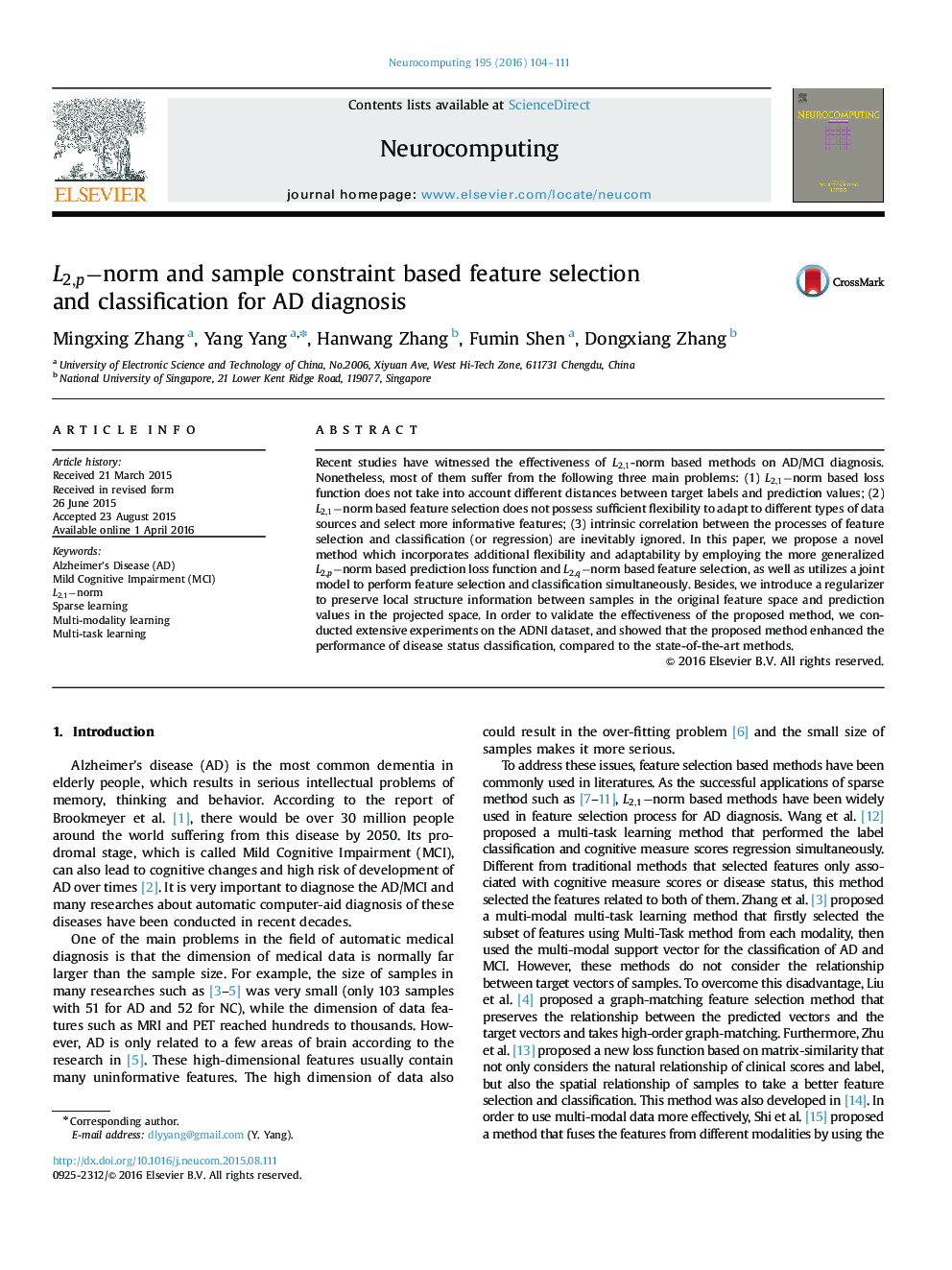
Recent studies have witnessed the effectiveness of L2,1L2,1-norm based methods on AD/MCI diagnosis. Nonetheless, most of them suffer from the following three main problems: (1) L2,1-normL2,1-norm based loss function does not take into account different distances between target labels and prediction values; (2) L2,1-normL2,1-norm based feature selection does not possess sufficient flexibility to adapt to different types of data sources and select more informative features; (3) intrinsic correlation between the processes of feature selection and classification (or regression) are inevitably ignored. In this paper, we propose a novel method which incorporates additional flexibility and adaptability by employing the more generalized L2,p-normL2,p-norm based prediction loss function and L2,q-normL2,q-norm based feature selection, as well as utilizes a joint model to perform feature selection and classification simultaneously. Besides, we introduce a regularizer to preserve local structure information between samples in the original feature space and prediction values in the projected space. In order to validate the effectiveness of the proposed method, we conducted extensive experiments on the ADNI dataset, and showed that the proposed method enhanced the performance of disease status classification, compared to the state-of-the-art methods.
Journal: Neurocomputing - Volume 195, 26 June 2016, Pages 104–111