کد مقاله | کد نشریه | سال انتشار | مقاله انگلیسی | نسخه تمام متن |
---|---|---|---|---|
411621 | 679578 | 2016 | 10 صفحه PDF | دانلود رایگان |
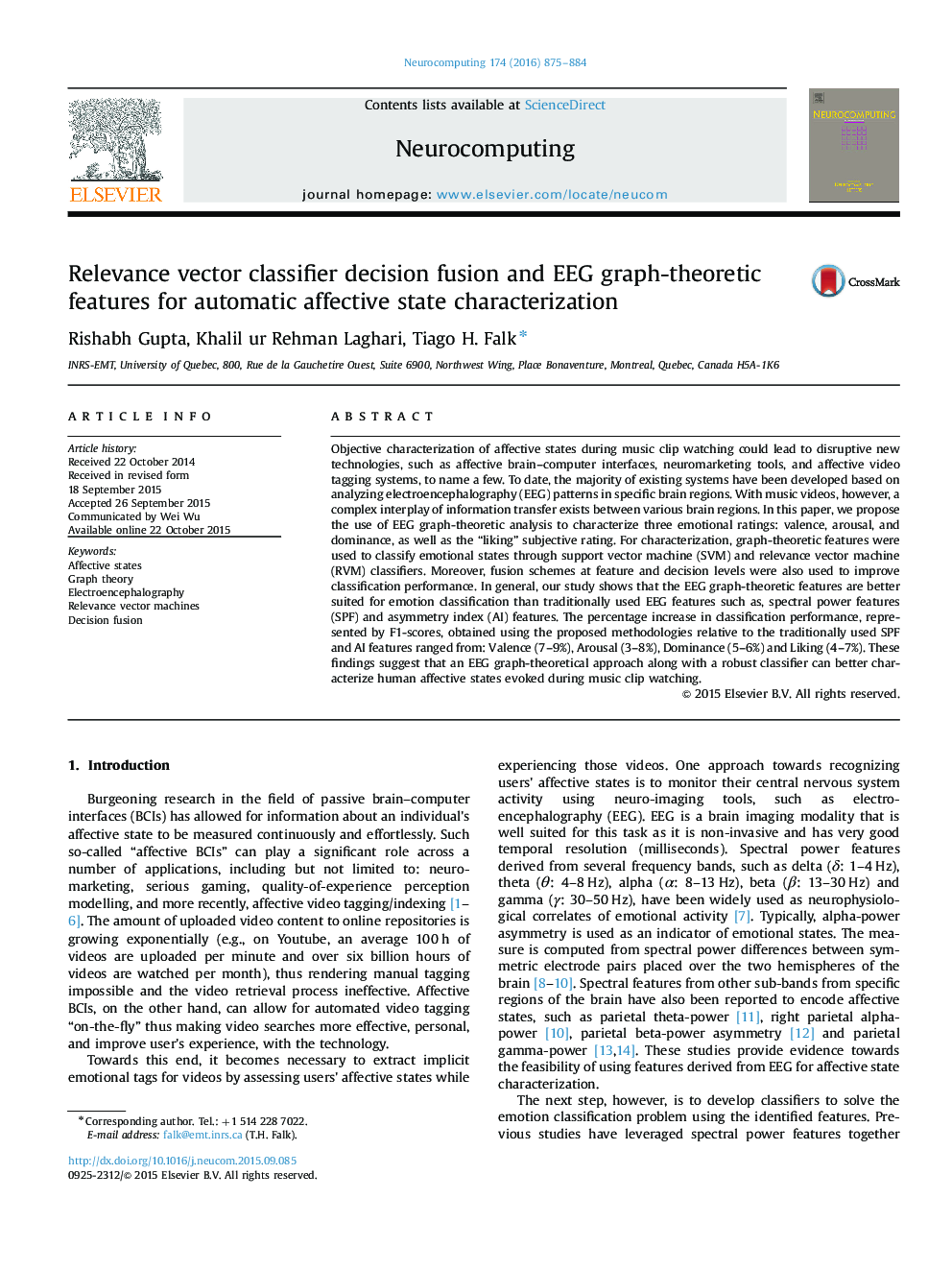
Objective characterization of affective states during music clip watching could lead to disruptive new technologies, such as affective brain–computer interfaces, neuromarketing tools, and affective video tagging systems, to name a few. To date, the majority of existing systems have been developed based on analyzing electroencephalography (EEG) patterns in specific brain regions. With music videos, however, a complex interplay of information transfer exists between various brain regions. In this paper, we propose the use of EEG graph-theoretic analysis to characterize three emotional ratings: valence, arousal, and dominance, as well as the “liking” subjective rating. For characterization, graph-theoretic features were used to classify emotional states through support vector machine (SVM) and relevance vector machine (RVM) classifiers. Moreover, fusion schemes at feature and decision levels were also used to improve classification performance. In general, our study shows that the EEG graph-theoretic features are better suited for emotion classification than traditionally used EEG features such as, spectral power features (SPF) and asymmetry index (AI) features. The percentage increase in classification performance, represented by F1-scores, obtained using the proposed methodologies relative to the traditionally used SPF and AI features ranged from: Valence (7–9%), Arousal (3–8%), Dominance (5–6%) and Liking (4–7%). These findings suggest that an EEG graph-theoretical approach along with a robust classifier can better characterize human affective states evoked during music clip watching.
Journal: Neurocomputing - Volume 174, Part B, 22 January 2016, Pages 875–884