کد مقاله | کد نشریه | سال انتشار | مقاله انگلیسی | نسخه تمام متن |
---|---|---|---|---|
412278 | 679623 | 2014 | 9 صفحه PDF | دانلود رایگان |
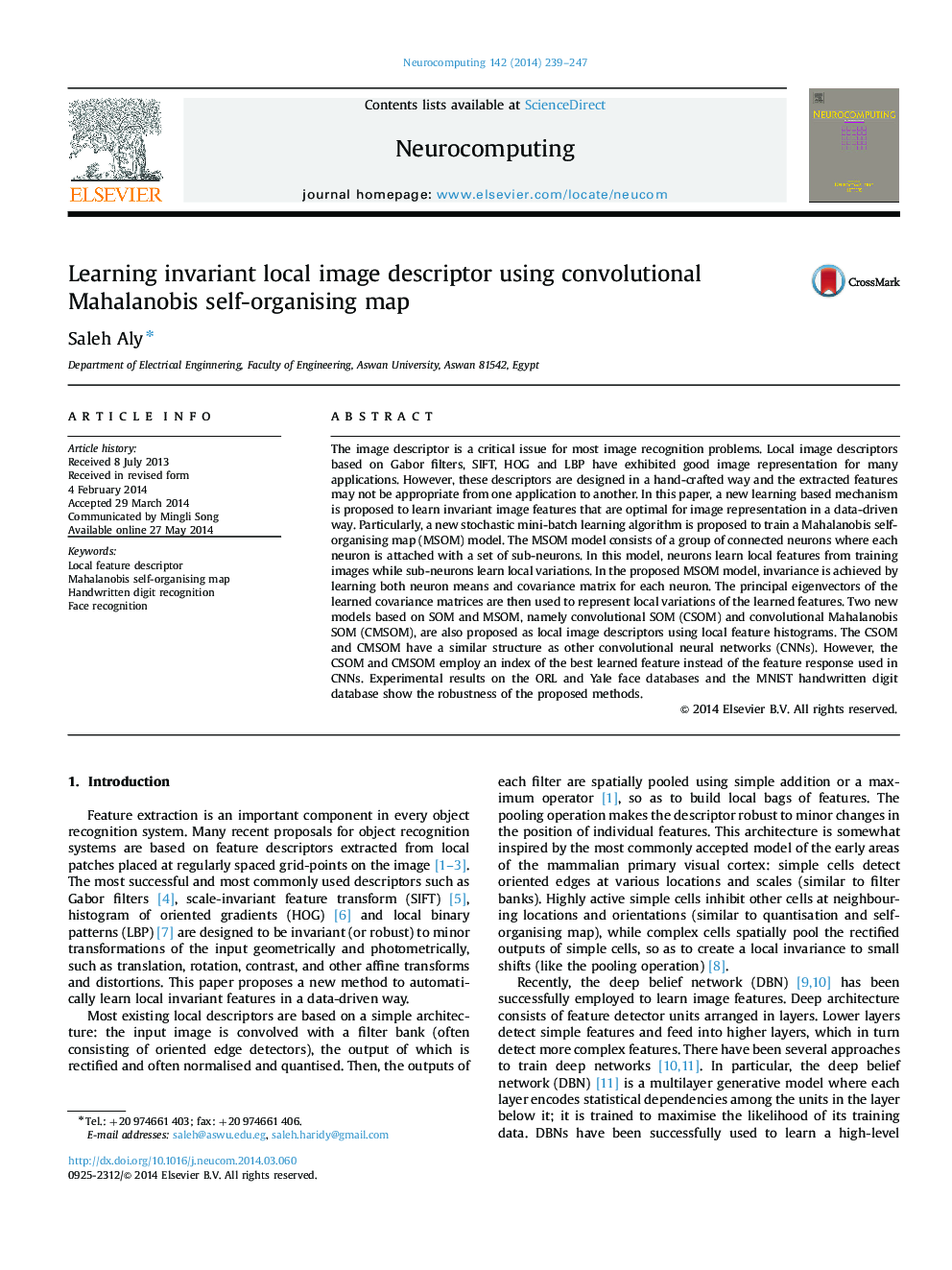
The image descriptor is a critical issue for most image recognition problems. Local image descriptors based on Gabor filters, SIFT, HOG and LBP have exhibited good image representation for many applications. However, these descriptors are designed in a hand-crafted way and the extracted features may not be appropriate from one application to another. In this paper, a new learning based mechanism is proposed to learn invariant image features that are optimal for image representation in a data-driven way. Particularly, a new stochastic mini-batch learning algorithm is proposed to train a Mahalanobis self-organising map (MSOM) model. The MSOM model consists of a group of connected neurons where each neuron is attached with a set of sub-neurons. In this model, neurons learn local features from training images while sub-neurons learn local variations. In the proposed MSOM model, invariance is achieved by learning both neuron means and covariance matrix for each neuron. The principal eigenvectors of the learned covariance matrices are then used to represent local variations of the learned features. Two new models based on SOM and MSOM, namely convolutional SOM (CSOM) and convolutional Mahalanobis SOM (CMSOM), are also proposed as local image descriptors using local feature histograms. The CSOM and CMSOM have a similar structure as other convolutional neural networks (CNNs). However, the CSOM and CMSOM employ an index of the best learned feature instead of the feature response used in CNNs. Experimental results on the ORL and Yale face databases and the MNIST handwritten digit database show the robustness of the proposed methods.
Journal: Neurocomputing - Volume 142, 22 October 2014, Pages 239–247