کد مقاله | کد نشریه | سال انتشار | مقاله انگلیسی | نسخه تمام متن |
---|---|---|---|---|
4375429 | 1303267 | 2007 | 8 صفحه PDF | دانلود رایگان |
عنوان انگلیسی مقاله ISI
Assessing daily tropical rainfall variations using a neuro-fuzzy classification model
دانلود مقاله + سفارش ترجمه
دانلود مقاله ISI انگلیسی
رایگان برای ایرانیان
کلمات کلیدی
موضوعات مرتبط
علوم زیستی و بیوفناوری
علوم کشاورزی و بیولوژیک
بوم شناسی، تکامل، رفتار و سامانه شناسی
پیش نمایش صفحه اول مقاله
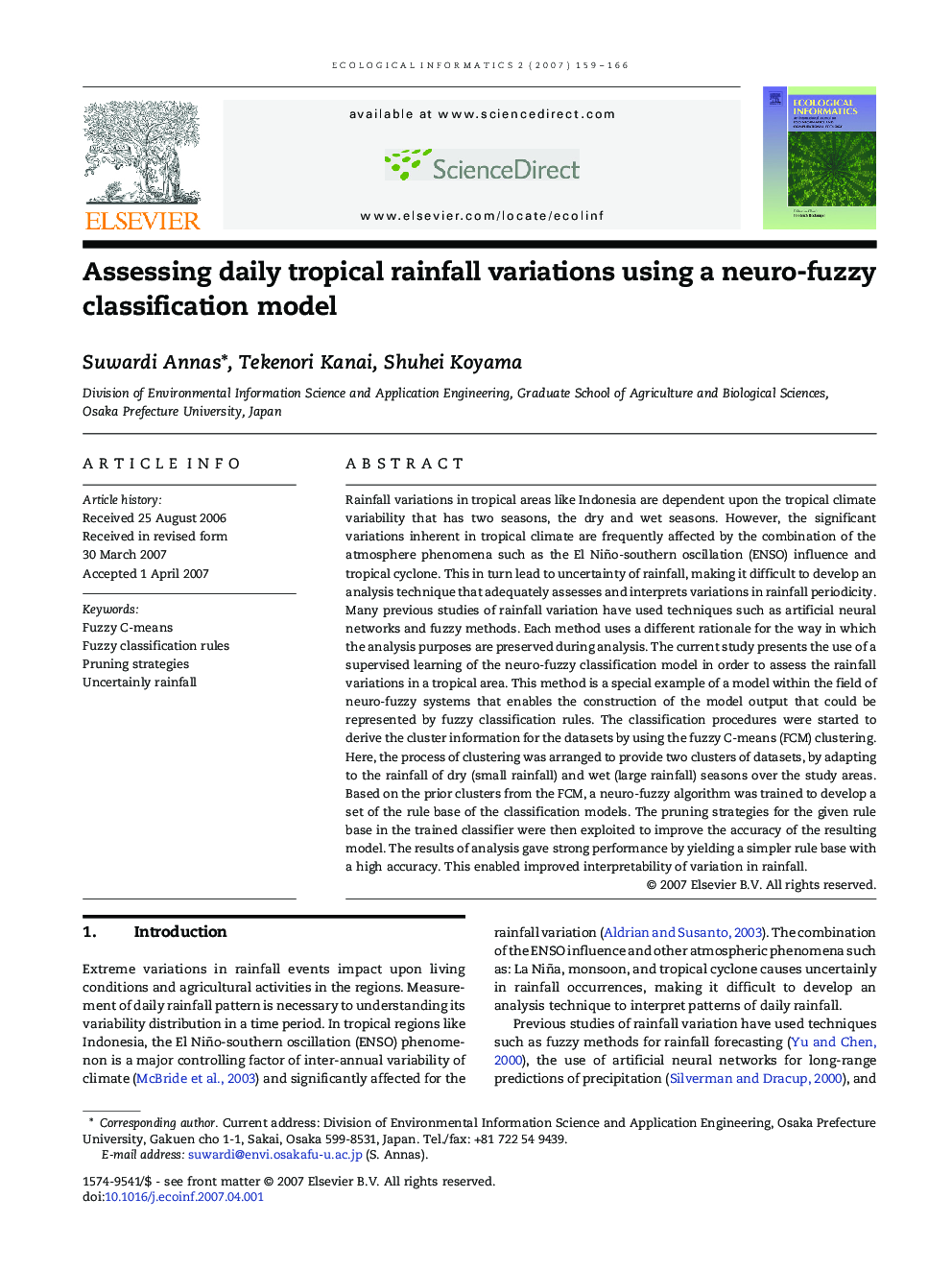
چکیده انگلیسی
Rainfall variations in tropical areas like Indonesia are dependent upon the tropical climate variability that has two seasons, the dry and wet seasons. However, the significant variations inherent in tropical climate are frequently affected by the combination of the atmosphere phenomena such as the El Niño-southern oscillation (ENSO) influence and tropical cyclone. This in turn lead to uncertainty of rainfall, making it difficult to develop an analysis technique that adequately assesses and interprets variations in rainfall periodicity. Many previous studies of rainfall variation have used techniques such as artificial neural networks and fuzzy methods. Each method uses a different rationale for the way in which the analysis purposes are preserved during analysis. The current study presents the use of a supervised learning of the neuro-fuzzy classification model in order to assess the rainfall variations in a tropical area. This method is a special example of a model within the field of neuro-fuzzy systems that enables the construction of the model output that could be represented by fuzzy classification rules. The classification procedures were started to derive the cluster information for the datasets by using the fuzzy C-means (FCM) clustering. Here, the process of clustering was arranged to provide two clusters of datasets, by adapting to the rainfall of dry (small rainfall) and wet (large rainfall) seasons over the study areas. Based on the prior clusters from the FCM, a neuro-fuzzy algorithm was trained to develop a set of the rule base of the classification models. The pruning strategies for the given rule base in the trained classifier were then exploited to improve the accuracy of the resulting model. The results of analysis gave strong performance by yielding a simpler rule base with a high accuracy. This enabled improved interpretability of variation in rainfall.
ناشر
Database: Elsevier - ScienceDirect (ساینس دایرکت)
Journal: Ecological Informatics - Volume 2, Issue 2, 1 June 2007, Pages 159-166
Journal: Ecological Informatics - Volume 2, Issue 2, 1 June 2007, Pages 159-166
نویسندگان
Suwardi Annas, Tekenori Kanai, Shuhei Koyama,