کد مقاله | کد نشریه | سال انتشار | مقاله انگلیسی | نسخه تمام متن |
---|---|---|---|---|
4459048 | 1621276 | 2012 | 17 صفحه PDF | دانلود رایگان |
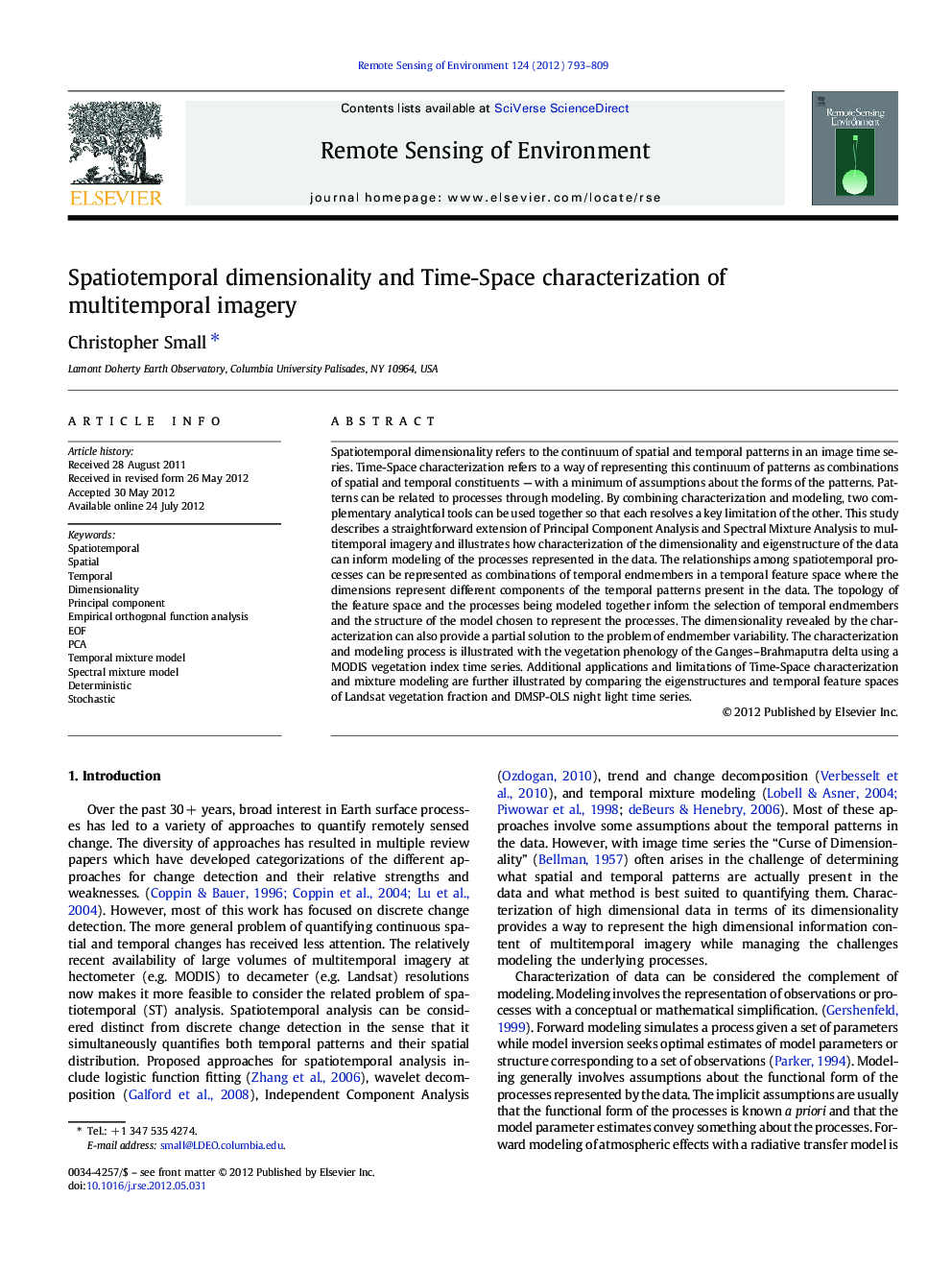
Spatiotemporal dimensionality refers to the continuum of spatial and temporal patterns in an image time series. Time-Space characterization refers to a way of representing this continuum of patterns as combinations of spatial and temporal constituents — with a minimum of assumptions about the forms of the patterns. Patterns can be related to processes through modeling. By combining characterization and modeling, two complementary analytical tools can be used together so that each resolves a key limitation of the other. This study describes a straightforward extension of Principal Component Analysis and Spectral Mixture Analysis to multitemporal imagery and illustrates how characterization of the dimensionality and eigenstructure of the data can inform modeling of the processes represented in the data. The relationships among spatiotemporal processes can be represented as combinations of temporal endmembers in a temporal feature space where the dimensions represent different components of the temporal patterns present in the data. The topology of the feature space and the processes being modeled together inform the selection of temporal endmembers and the structure of the model chosen to represent the processes. The dimensionality revealed by the characterization can also provide a partial solution to the problem of endmember variability. The characterization and modeling process is illustrated with the vegetation phenology of the Ganges–Brahmaputra delta using a MODIS vegetation index time series. Additional applications and limitations of Time-Space characterization and mixture modeling are further illustrated by comparing the eigenstructures and temporal feature spaces of Landsat vegetation fraction and DMSP-OLS night light time series.
► A general approach for multitemporal image analysis combining characterization and modeling.
► Characterization reveals number and structure of spatiotemporal patterns in image time series.
► Temporal feature space informs model design and reveals endmembers for temporal mixture model.
Journal: Remote Sensing of Environment - Volume 124, September 2012, Pages 793–809