کد مقاله | کد نشریه | سال انتشار | مقاله انگلیسی | نسخه تمام متن |
---|---|---|---|---|
456217 | 695675 | 2008 | 8 صفحه PDF | دانلود رایگان |
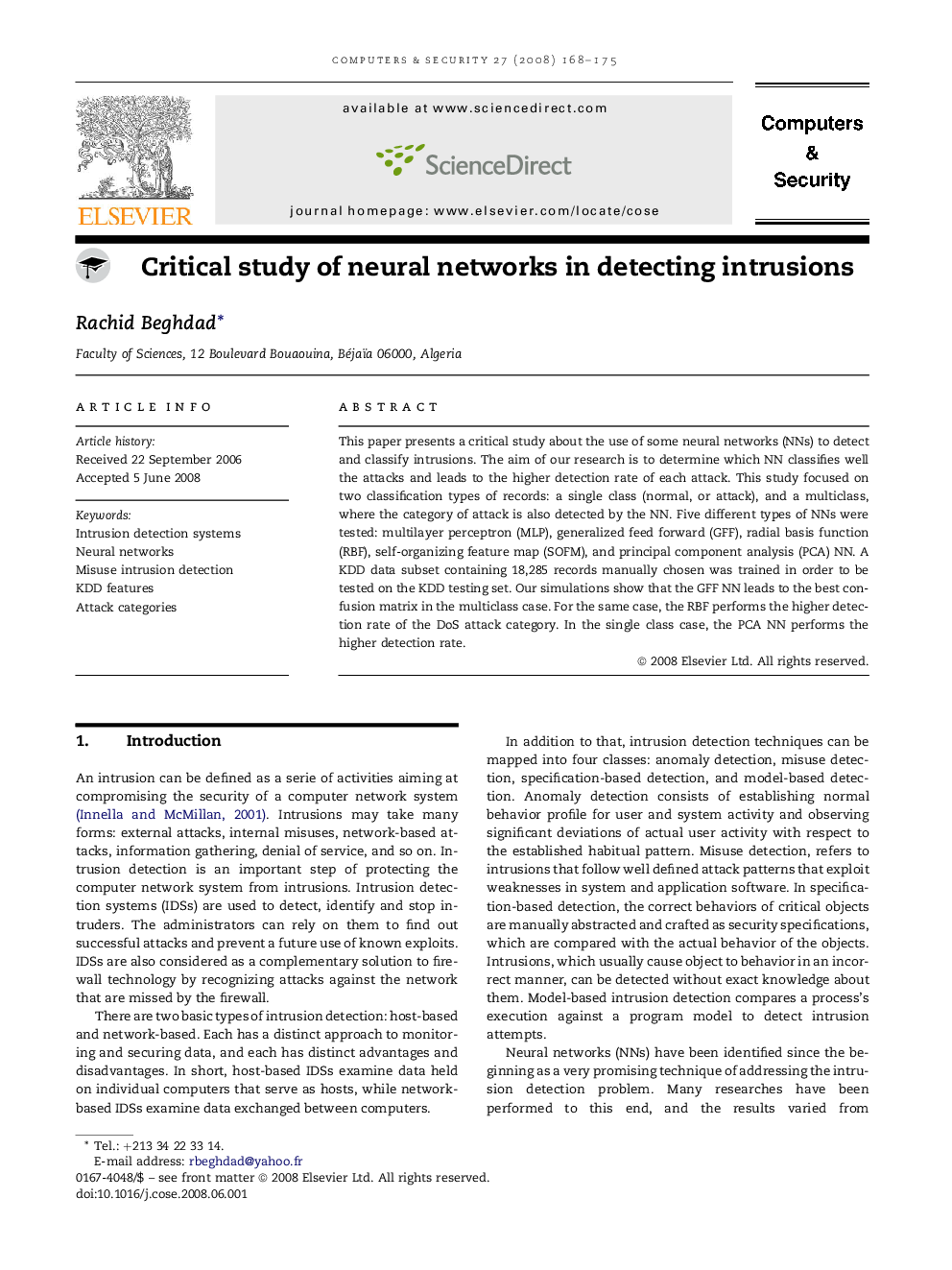
This paper presents a critical study about the use of some neural networks (NNs) to detect and classify intrusions. The aim of our research is to determine which NN classifies well the attacks and leads to the higher detection rate of each attack. This study focused on two classification types of records: a single class (normal, or attack), and a multiclass, where the category of attack is also detected by the NN. Five different types of NNs were tested: multilayer perceptron (MLP), generalized feed forward (GFF), radial basis function (RBF), self-organizing feature map (SOFM), and principal component analysis (PCA) NN. A KDD data subset containing 18,285 records manually chosen was trained in order to be tested on the KDD testing set. Our simulations show that the GFF NN leads to the best confusion matrix in the multiclass case. For the same case, the RBF performs the higher detection rate of the DoS attack category. In the single class case, the PCA NN performs the higher detection rate.
Journal: Computers & Security - Volume 27, Issues 5–6, October 2008, Pages 168–175