کد مقاله | کد نشریه | سال انتشار | مقاله انگلیسی | نسخه تمام متن |
---|---|---|---|---|
4573989 | 1629502 | 2012 | 10 صفحه PDF | دانلود رایگان |
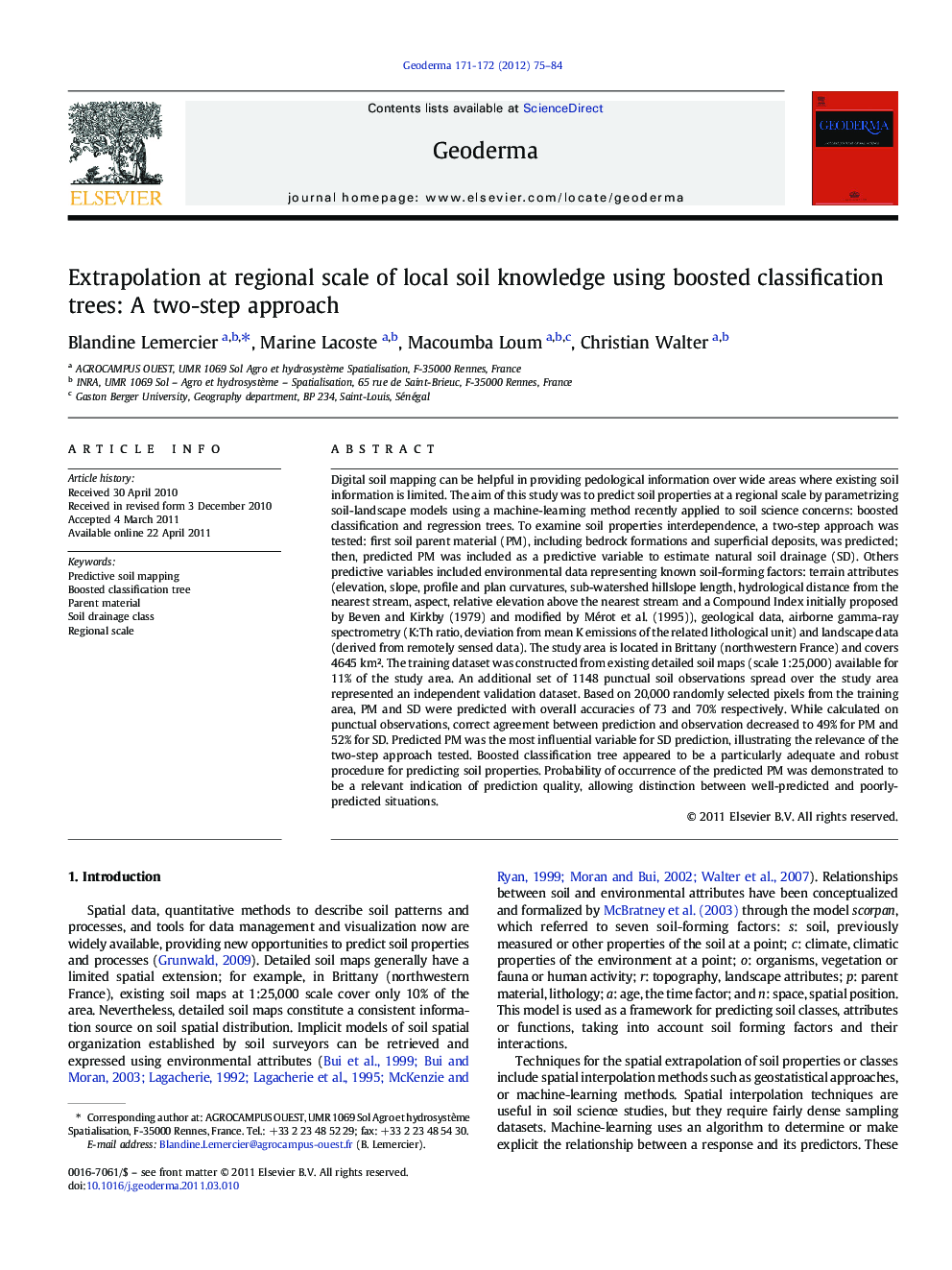
Digital soil mapping can be helpful in providing pedological information over wide areas where existing soil information is limited. The aim of this study was to predict soil properties at a regional scale by parametrizing soil-landscape models using a machine-learning method recently applied to soil science concerns: boosted classification and regression trees. To examine soil properties interdependence, a two-step approach was tested: first soil parent material (PM), including bedrock formations and superficial deposits, was predicted; then, predicted PM was included as a predictive variable to estimate natural soil drainage (SD). Others predictive variables included environmental data representing known soil-forming factors: terrain attributes (elevation, slope, profile and plan curvatures, sub-watershed hillslope length, hydrological distance from the nearest stream, aspect, relative elevation above the nearest stream and a Compound Index initially proposed by Beven and Kirkby (1979) and modified by Mérot et al. (1995)), geological data, airborne gamma-ray spectrometry (K:Th ratio, deviation from mean K emissions of the related lithological unit) and landscape data (derived from remotely sensed data). The study area is located in Brittany (northwestern France) and covers 4645 km². The training dataset was constructed from existing detailed soil maps (scale 1:25,000) available for 11% of the study area. An additional set of 1148 punctual soil observations spread over the study area represented an independent validation dataset. Based on 20,000 randomly selected pixels from the training area, PM and SD were predicted with overall accuracies of 73 and 70% respectively. While calculated on punctual observations, correct agreement between prediction and observation decreased to 49% for PM and 52% for SD. Predicted PM was the most influential variable for SD prediction, illustrating the relevance of the two-step approach tested. Boosted classification tree appeared to be a particularly adequate and robust procedure for predicting soil properties. Probability of occurrence of the predicted PM was demonstrated to be a relevant indication of prediction quality, allowing distinction between well-predicted and poorly-predicted situations.
Research highlights
► We used soil-landscape models to predict parent material (PM) and soil drainage (SD).
► A two-step approach was tested: predicted PM was included in the SD predictive dataset.
► Overall prediction accuracies were of 73% for PM and 70% for SD.
► Predicted PM was the most influential variable for SD prediction.
► Probability of occurrence of the predicted PM is a relevant indicator of prediction quality.
Journal: Geoderma - Volumes 171–172, February 2012, Pages 75–84