کد مقاله | کد نشریه | سال انتشار | مقاله انگلیسی | نسخه تمام متن |
---|---|---|---|---|
490380 | 707462 | 2013 | 10 صفحه PDF | دانلود رایگان |
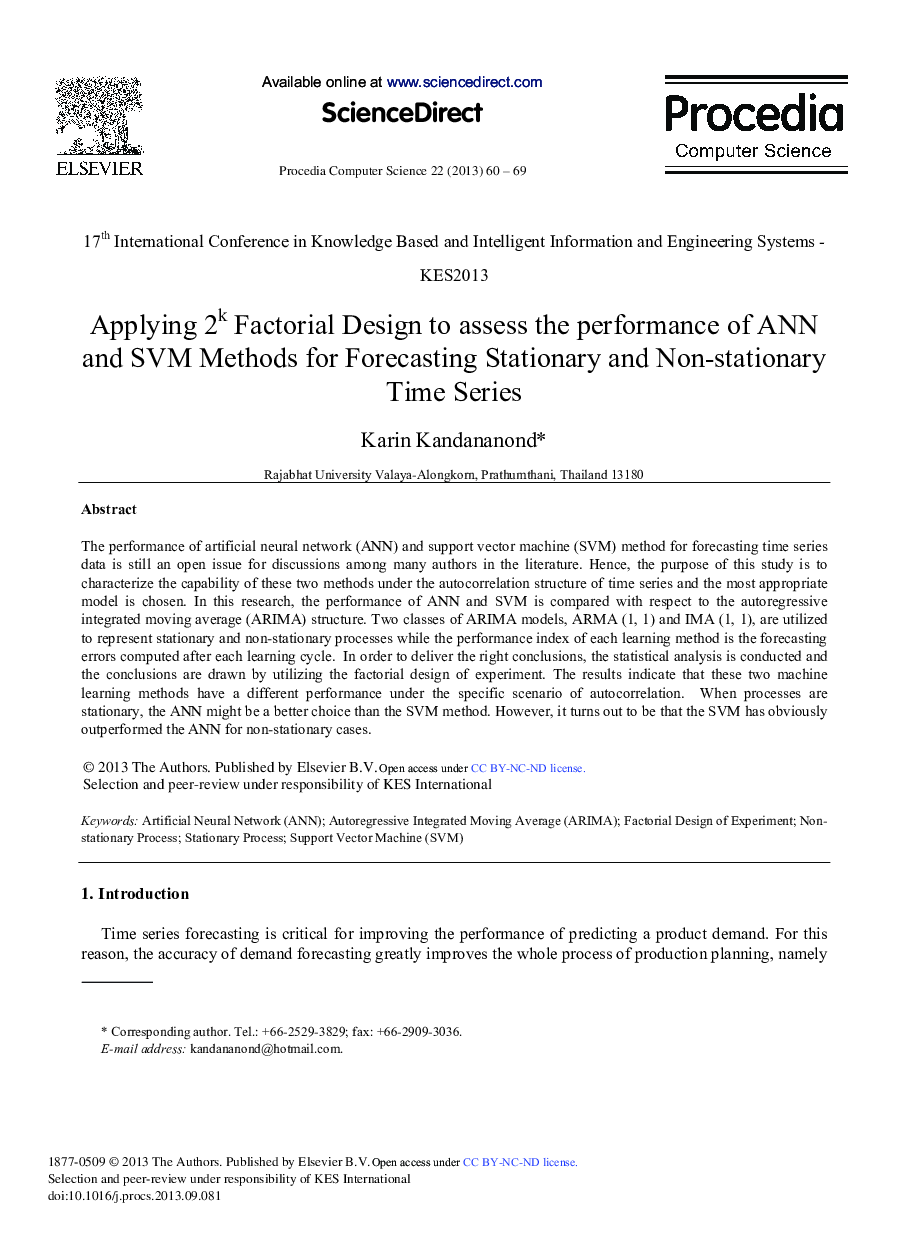
The performance of artificial neural network (ANN) and support vector machine (SVM) method for forecasting time series data is still an open issue for discussions among many authors in the literature. Hence, the purpose of this study is to characterize the capability of these two methods under the autocorrelation structure of time series and the most appropriate model is chosen. In this research, the performance of ANN and SVM is compared with respect to the autoregressive integrated moving average (ARIMA) structure. Two classes of ARIMA models, ARMA (1, 1) and IMA (1, 1), are utilized to represent stationary and non-stationary processes while the performance index of each learning method is the forecasting errors computed after each learning cycle. In order to deliver the right conclusions, the statistical analysis is conducted and the conclusions are drawn by utilizing the factorial design of experiment. The results indicate that these two machine learning methods have a different performance under the specific scenario of autocorrelation. When processes are stationary, the ANN might be a better choice than the SVM method. However, it turns out to be that the SVM has obviously outperformed the ANN for non-stationary cases.
Journal: Procedia Computer Science - Volume 22, 2013, Pages 60-69