کد مقاله | کد نشریه | سال انتشار | مقاله انگلیسی | نسخه تمام متن |
---|---|---|---|---|
4946138 | 1439269 | 2017 | 13 صفحه PDF | دانلود رایگان |
عنوان انگلیسی مقاله ISI
GMDH-based semi-supervised feature selection for customer classification
دانلود مقاله + سفارش ترجمه
دانلود مقاله ISI انگلیسی
رایگان برای ایرانیان
کلمات کلیدی
موضوعات مرتبط
مهندسی و علوم پایه
مهندسی کامپیوتر
هوش مصنوعی
پیش نمایش صفحه اول مقاله
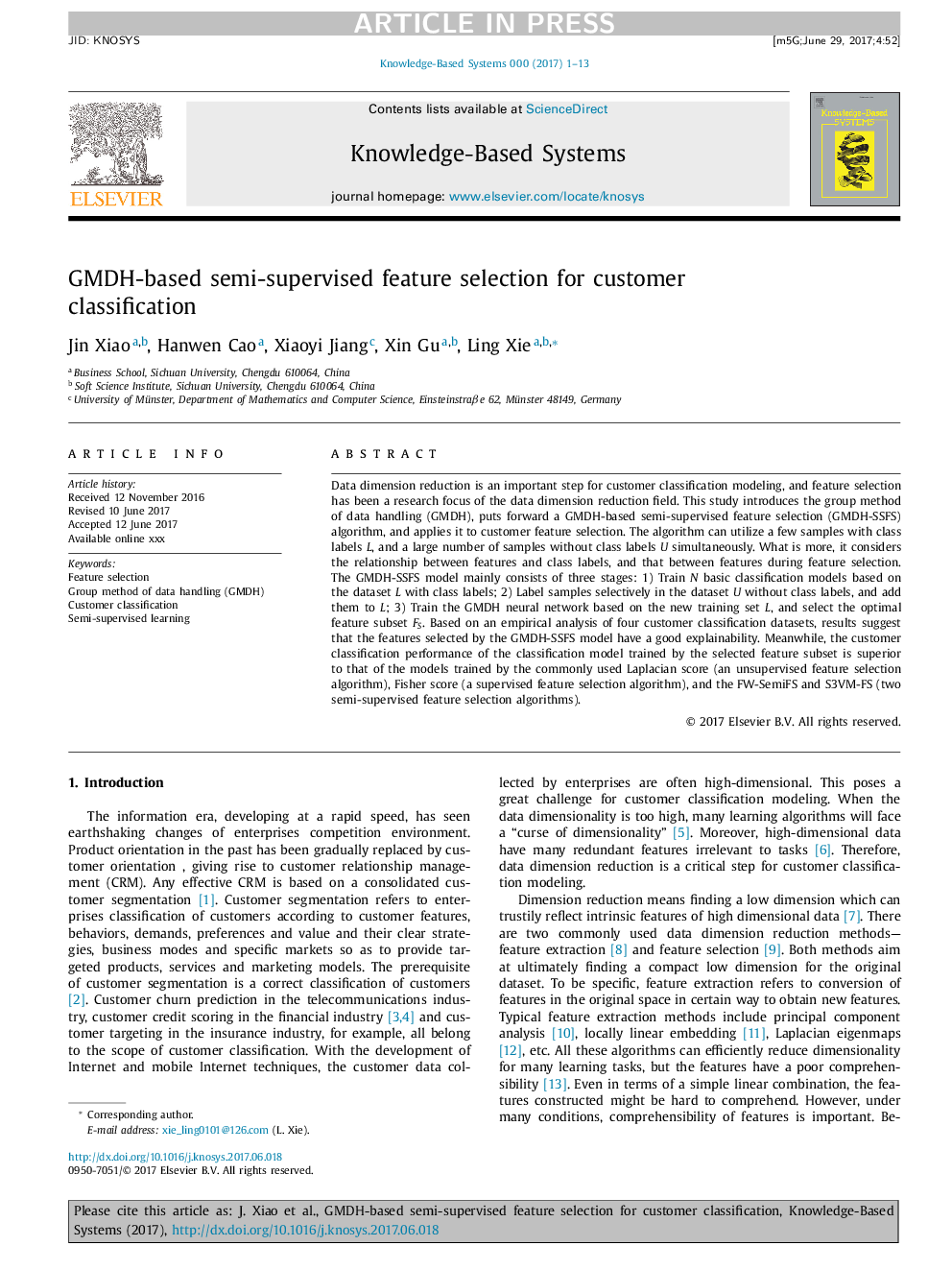
چکیده انگلیسی
Data dimension reduction is an important step for customer classification modeling, and feature selection has been a research focus of the data dimension reduction field. This study introduces the group method of data handling (GMDH), puts forward a GMDH-based semi-supervised feature selection (GMDH-SSFS) algorithm, and applies it to customer feature selection. The algorithm can utilize a few samples with class labels L, and a large number of samples without class labels U simultaneously. What is more, it considers the relationship between features and class labels, and that between features during feature selection. The GMDH-SSFS model mainly consists of three stages: 1) Train N basic classification models based on the dataset L with class labels; 2) Label samples selectively in the dataset U without class labels, and add them to L; 3) Train the GMDH neural network based on the new training set L, and select the optimal feature subset FS. Based on an empirical analysis of four customer classification datasets, results suggest that the features selected by the GMDH-SSFS model have a good explainability. Meanwhile, the customer classification performance of the classification model trained by the selected feature subset is superior to that of the models trained by the commonly used Laplacian score (an unsupervised feature selection algorithm), Fisher score (a supervised feature selection algorithm), and the FW-SemiFS and S3VM-FS (two semi-supervised feature selection algorithms).
ناشر
Database: Elsevier - ScienceDirect (ساینس دایرکت)
Journal: Knowledge-Based Systems - Volume 132, 15 September 2017, Pages 236-248
Journal: Knowledge-Based Systems - Volume 132, 15 September 2017, Pages 236-248
نویسندگان
Jin Xiao, Hanwen Cao, Xiaoyi Jiang, Xin Gu, Ling Xie,