کد مقاله | کد نشریه | سال انتشار | مقاله انگلیسی | نسخه تمام متن |
---|---|---|---|---|
4948067 | 1439602 | 2017 | 22 صفحه PDF | دانلود رایگان |
عنوان انگلیسی مقاله ISI
Sparse Bayesian multiway canonical correlation analysis for EEG pattern recognition
دانلود مقاله + سفارش ترجمه
دانلود مقاله ISI انگلیسی
رایگان برای ایرانیان
کلمات کلیدی
موضوعات مرتبط
مهندسی و علوم پایه
مهندسی کامپیوتر
هوش مصنوعی
پیش نمایش صفحه اول مقاله
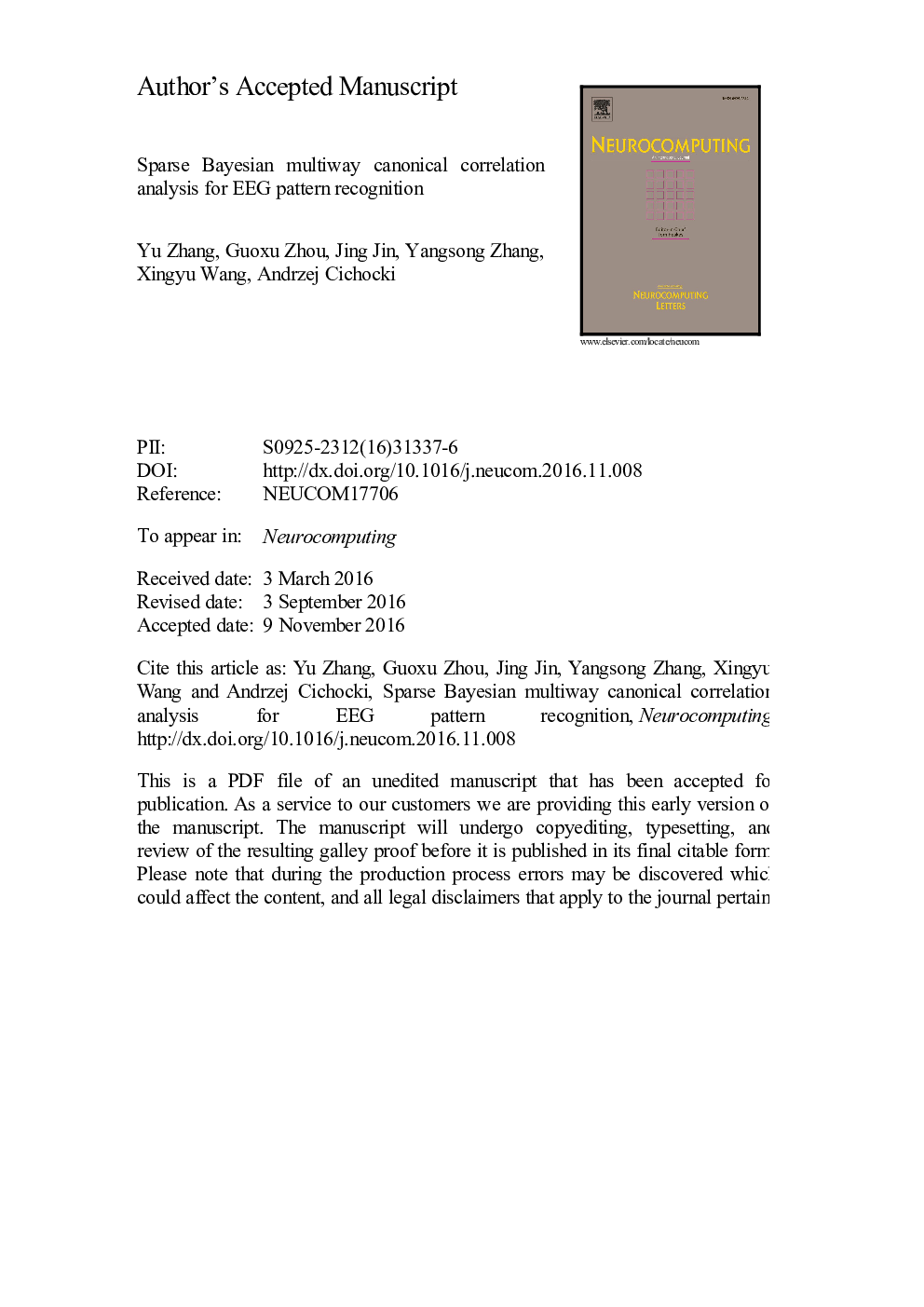
چکیده انگلیسی
L1-regularized multiway canonical correlation analysis (L1-MCCA) has been introduced to reference signal optimization in steady-state visual evoked potential (SSVEP)-based brain-computer interface (BCI). The effectiveness of L1-regularization on significant trial selection highly depends on the regularization parameter setting, which can be typically determined by cross-validation (CV). However, CV will substantially reduce the practicability of BCI system due to additional data requirement for the parameter validation and relatively high computational cost. To solve the problem, this study proposes a Bayesian version of L1-MCCA (called SBMCCA) by exploiting sparse Bayesian learning. The SBMCCA method avoids CV and can efficiently estimate the model parameters under the Bayesian evidence framework. Experimental results show that the SBMCCA method achieved comparable recognition accuracy but much higher computational efficiency in contrast to the L1-MCCA method.
ناشر
Database: Elsevier - ScienceDirect (ساینس دایرکت)
Journal: Neurocomputing - Volume 225, 15 February 2017, Pages 103-110
Journal: Neurocomputing - Volume 225, 15 February 2017, Pages 103-110
نویسندگان
Yu Zhang, Guoxu Zhou, Jing Jin, Yangsong Zhang, Xingyu Wang, Andrzej Cichocki,