کد مقاله | کد نشریه | سال انتشار | مقاله انگلیسی | نسخه تمام متن |
---|---|---|---|---|
4968700 | 1449740 | 2017 | 43 صفحه PDF | دانلود رایگان |
عنوان انگلیسی مقاله ISI
Cluster-based adaptive SVM: A latent subdomains discovery method for domain adaptation problems
دانلود مقاله + سفارش ترجمه
دانلود مقاله ISI انگلیسی
رایگان برای ایرانیان
کلمات کلیدی
موضوعات مرتبط
مهندسی و علوم پایه
مهندسی کامپیوتر
چشم انداز کامپیوتر و تشخیص الگو
پیش نمایش صفحه اول مقاله
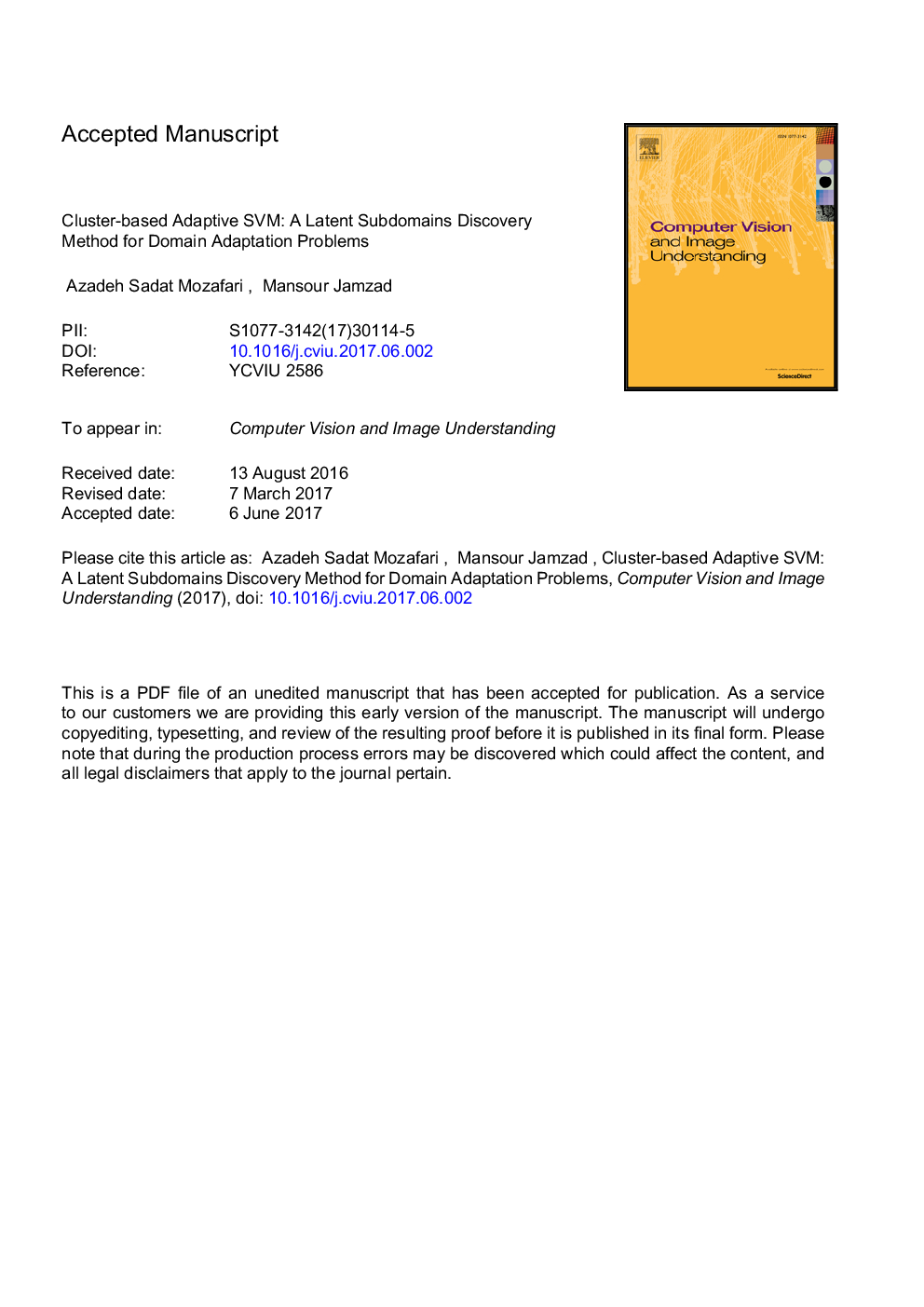
چکیده انگلیسی
Machine learning algorithms often suffer from good generalization in testing domains especially when the training (source) and test (target) domains do not have similar distributions. To address this problem, several domain adaptation techniques have been proposed to improve the performance of the learning algorithms when they face accuracy degradation caused by the domain shift problem. In this paper, we focus on the non-homogeneous distributed target domains and propose a new latent subdomain discovery model to divide the target domain into subdomains while adapting them. It is expected that applying adaptation on subdomains increase the rate of detection in comparing with the situation that the target domain is seen as one single domain. The proposed division method considers each subdomain as a cluster which has the definite ratio of positive to negative samples, linear discriminability and conditional distribution similarity to the source domain. This method divides the target domain into subdomains while adapting the trained target classifier for each subdomain using Adapt-SVM adaptation method. It also has a simple solution for selecting the appropriate number of subdomains. We call our proposed method Cluster-based Adaptive SVM or CA-SVM in short. We test CA-SVM on two different computer vision problems, pedestrian detection and image classification. The experimental results show the advantage in accuracy rate for our approach in comparison to several baselines.
ناشر
Database: Elsevier - ScienceDirect (ساینس دایرکت)
Journal: Computer Vision and Image Understanding - Volume 162, September 2017, Pages 116-134
Journal: Computer Vision and Image Understanding - Volume 162, September 2017, Pages 116-134
نویسندگان
Azadeh Sadat Mozafari, Mansour Jamzad,