کد مقاله | کد نشریه | سال انتشار | مقاله انگلیسی | نسخه تمام متن |
---|---|---|---|---|
4968982 | 1449843 | 2017 | 16 صفحه PDF | دانلود رایگان |
عنوان انگلیسی مقاله ISI
A learning-based variable size part extraction architecture for 6D object pose recovery in depth images
دانلود مقاله + سفارش ترجمه
دانلود مقاله ISI انگلیسی
رایگان برای ایرانیان
کلمات کلیدی
موضوعات مرتبط
مهندسی و علوم پایه
مهندسی کامپیوتر
چشم انداز کامپیوتر و تشخیص الگو
پیش نمایش صفحه اول مقاله
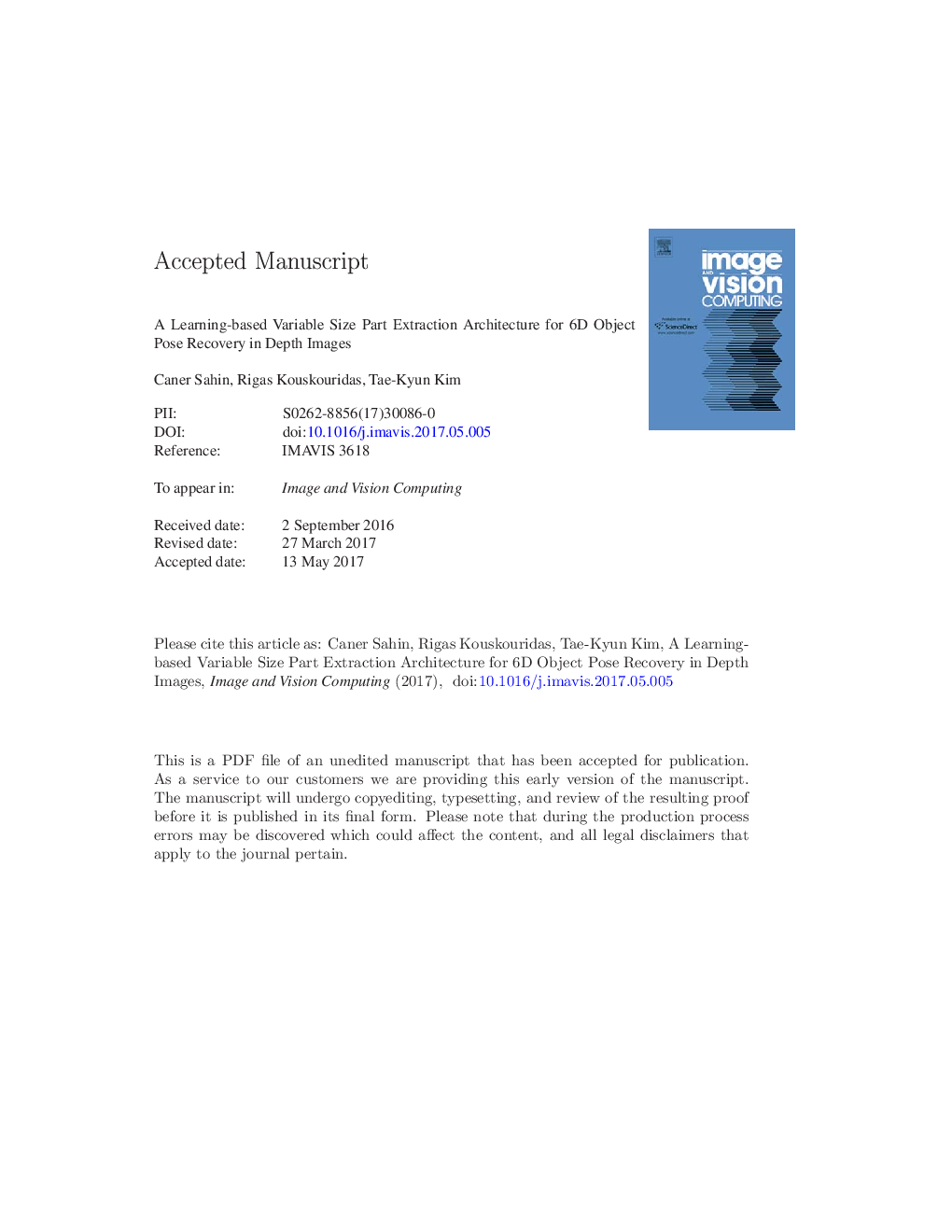
چکیده انگلیسی
State-of-the-art techniques for 6D object pose recovery depend on occlusion-free point clouds to accurately register objects in 3D space. To deal with this shortcoming, we introduce a novel architecture called Iterative Hough Forest with Histogram of Control Points that is capable of estimating the 6D pose of an occluded and cluttered object, given a candidate 2D bounding box. Our Iterative Hough Forest (IHF) is learnt using parts extracted only from the positive samples. These parts are represented with Histogram of Control Points (HoCP), a “scale-variant” implicit volumetric description, which we derive from recently introduced Implicit B-Splines (IBS). The rich discriminative information provided by the scale-variant HoCP features is leveraged during inference. An automatic variable size part extraction framework iteratively refines the object's roughly aligned initial pose due to the extraction of coarsest parts, the ones occupying the largest area in image pixels. The iterative refinement is accomplished based on finer (smaller) parts, which are represented with more discriminative control point descriptors by using our Iterative Hough Forest. Experiments conducted on a publicly available dataset report that our approach shows better registration performance than the state-of-the-art methods.
ناشر
Database: Elsevier - ScienceDirect (ساینس دایرکت)
Journal: Image and Vision Computing - Volume 63, July 2017, Pages 38-50
Journal: Image and Vision Computing - Volume 63, July 2017, Pages 38-50
نویسندگان
Caner Sahin, Rigas Kouskouridas, Tae-Kyun Kim,