کد مقاله | کد نشریه | سال انتشار | مقاله انگلیسی | نسخه تمام متن |
---|---|---|---|---|
4969001 | 1449849 | 2017 | 11 صفحه PDF | دانلود رایگان |
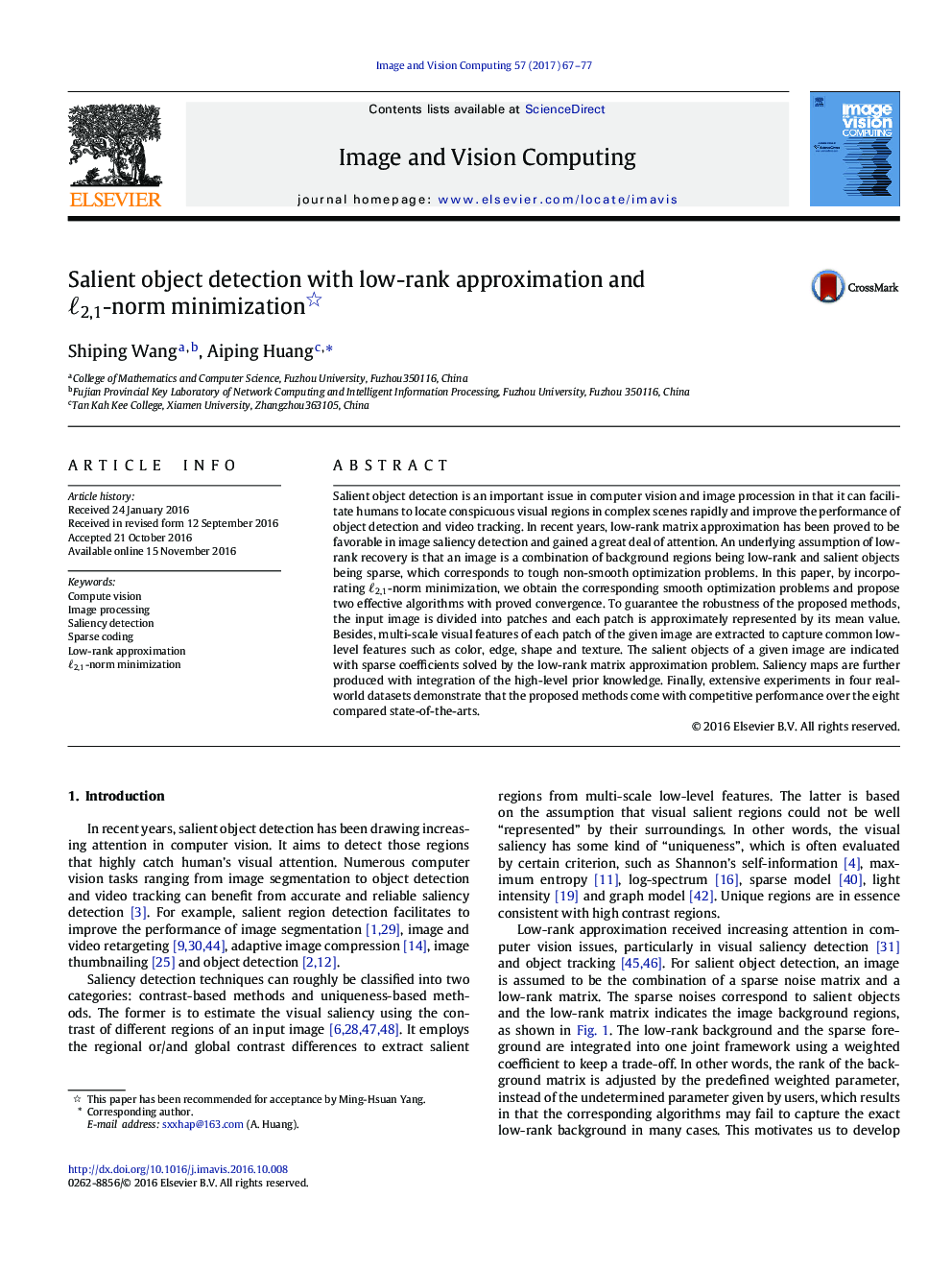
- Propose an efficient algorithm for low-rank approximation
- Develop a new method with proved convergence to learn sparsity
- Incorporate low-rank recovery and sparse coding into one joint framework
- Validate the proposed algorithm with parameter sensitivity and performance
Salient object detection is an important issue in computer vision and image procession in that it can facilitate humans to locate conspicuous visual regions in complex scenes rapidly and improve the performance of object detection and video tracking. In recent years, low-rank matrix approximation has been proved to be favorable in image saliency detection and gained a great deal of attention. An underlying assumption of low-rank recovery is that an image is a combination of background regions being low-rank and salient objects being sparse, which corresponds to tough non-smooth optimization problems. In this paper, by incorporating â2,1-norm minimization, we obtain the corresponding smooth optimization problems and propose two effective algorithms with proved convergence. To guarantee the robustness of the proposed methods, the input image is divided into patches and each patch is approximately represented by its mean value. Besides, multi-scale visual features of each patch of the given image are extracted to capture common low-level features such as color, edge, shape and texture. The salient objects of a given image are indicated with sparse coefficients solved by the low-rank matrix approximation problem. Saliency maps are further produced with integration of the high-level prior knowledge. Finally, extensive experiments in four real-world datasets demonstrate that the proposed methods come with competitive performance over the eight compared state-of-the-arts.
Graphical Abstract342
Journal: Image and Vision Computing - Volume 57, January 2017, Pages 67-77