کد مقاله | کد نشریه | سال انتشار | مقاله انگلیسی | نسخه تمام متن |
---|---|---|---|---|
4969590 | 1449974 | 2018 | 12 صفحه PDF | دانلود رایگان |
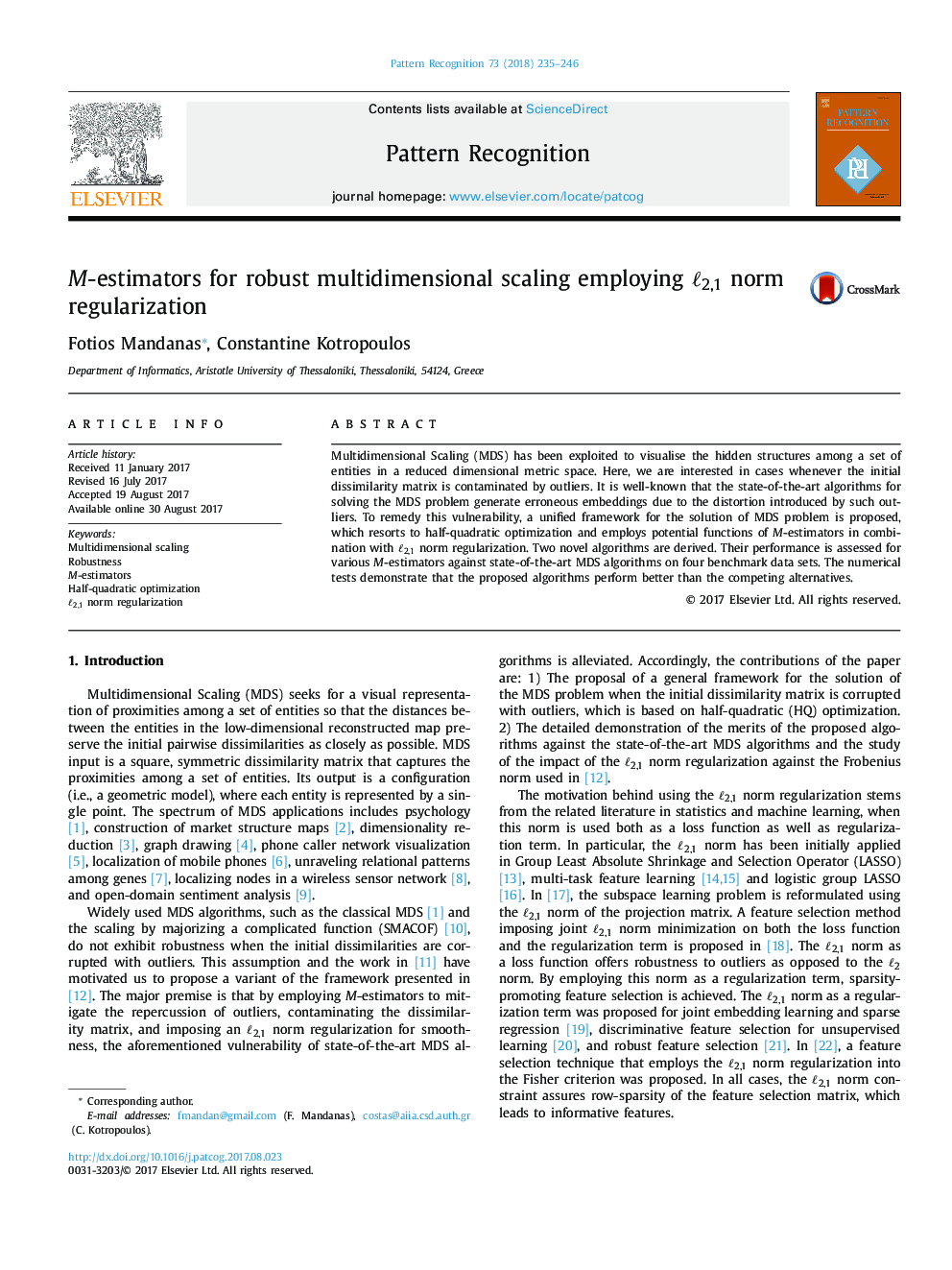
- The development of a general framework based on half quadratic minimization for the solution of the MDS problem when M-estimators are employed to mitigate the outliers influence and â2, 1 norm regularization is imposed for smoothness.
- The proposal of two novel algorithms derived by the general framework.
- The detailed demonstration of the merits of the proposed algorithms against the state-of-the art MDS algorithms.
- The assessment of various M-estimators.
- The study of the impact of the â2, 1 norm regularization against the Frobenius norm.
Multidimensional Scaling (MDS) has been exploited to visualise the hidden structures among a set of entities in a reduced dimensional metric space. Here, we are interested in cases whenever the initial dissimilarity matrix is contaminated by outliers. It is well-known that the state-of-the-art algorithms for solving the MDS problem generate erroneous embeddings due to the distortion introduced by such outliers. To remedy this vulnerability, a unified framework for the solution of MDS problem is proposed, which resorts to half-quadratic optimization and employs potential functions of M-estimators in combination with â2,1 norm regularization. Two novel algorithms are derived. Their performance is assessed for various M-estimators against state-of-the-art MDS algorithms on four benchmark data sets. The numerical tests demonstrate that the proposed algorithms perform better than the competing alternatives.
97HQMMDSL21 algorithm outperforms RMDS for λ2â¯ââ¯[1, 100] with respect to raw stress Ïr(X^). On the contrary, raw stress Ïr(X^) of the HQMMDS1 algorithm admits smaller values than those of the RMDS for λ2â¯ââ¯[1, 38]. The completely different performance of the â2, 1 norm, compared to the Frobenius one, can be attributed to the fact that â2, 1 norm regularization, being an intermediate state between â1 and Frobenius norms, mitigates the over-smoothness imposed by the Frobenius norm. Thus, for the same kernel size a, the range of λ2 values for which HQMMDSL21 attains a smaller raw stress Ïr(X^) than RMDS is always wider than that for HQMMDS1 algorithm.
Journal: Pattern Recognition - Volume 73, January 2018, Pages 235-246