کد مقاله | کد نشریه | سال انتشار | مقاله انگلیسی | نسخه تمام متن |
---|---|---|---|---|
4969598 | 1449975 | 2017 | 10 صفحه PDF | دانلود رایگان |
عنوان انگلیسی مقاله ISI
Centroid-aware local discriminative metric learning in speaker verification
دانلود مقاله + سفارش ترجمه
دانلود مقاله ISI انگلیسی
رایگان برای ایرانیان
موضوعات مرتبط
مهندسی و علوم پایه
مهندسی کامپیوتر
چشم انداز کامپیوتر و تشخیص الگو
پیش نمایش صفحه اول مقاله
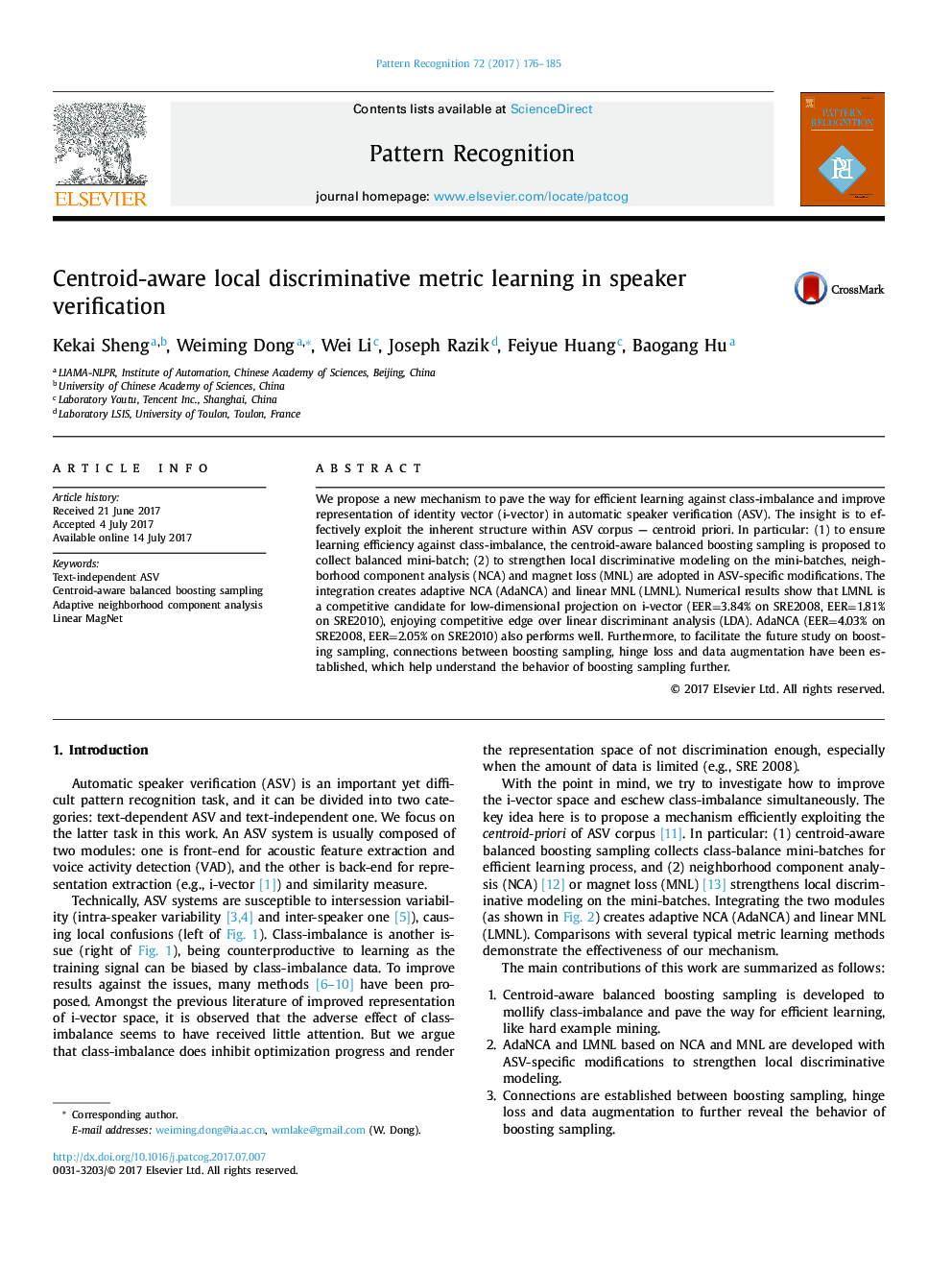
چکیده انگلیسی
We propose a new mechanism to pave the way for efficient learning against class-imbalance and improve representation of identity vector (i-vector) in automatic speaker verification (ASV). The insight is to effectively exploit the inherent structure within ASV corpus - centroid priori. In particular: (1) to ensure learning efficiency against class-imbalance, the centroid-aware balanced boosting sampling is proposed to collect balanced mini-batch; (2) to strengthen local discriminative modeling on the mini-batches, neighborhood component analysis (NCA) and magnet loss (MNL) are adopted in ASV-specific modifications. The integration creates adaptive NCA (AdaNCA) and linear MNL (LMNL). Numerical results show that LMNL is a competitive candidate for low-dimensional projection on i-vector (EER=3.84% on SRE2008, EER=1.81% on SRE2010), enjoying competitive edge over linear discriminant analysis (LDA). AdaNCA (EER=4.03% on SRE2008, EER=2.05% on SRE2010) also performs well. Furthermore, to facilitate the future study on boosting sampling, connections between boosting sampling, hinge loss and data augmentation have been established, which help understand the behavior of boosting sampling further.
ناشر
Database: Elsevier - ScienceDirect (ساینس دایرکت)
Journal: Pattern Recognition - Volume 72, December 2017, Pages 176-185
Journal: Pattern Recognition - Volume 72, December 2017, Pages 176-185
نویسندگان
Kekai Sheng, Weiming Dong, Wei Li, Joseph Razik, Feiyue Huang, Baogang Hu,