کد مقاله | کد نشریه | سال انتشار | مقاله انگلیسی | نسخه تمام متن |
---|---|---|---|---|
4969967 | 1450019 | 2017 | 7 صفحه PDF | دانلود رایگان |
عنوان انگلیسی مقاله ISI
Increasing the robustness of CNN acoustic models using autoregressive moving average spectrogram features and channel dropout
دانلود مقاله + سفارش ترجمه
دانلود مقاله ISI انگلیسی
رایگان برای ایرانیان
موضوعات مرتبط
مهندسی و علوم پایه
مهندسی کامپیوتر
چشم انداز کامپیوتر و تشخیص الگو
پیش نمایش صفحه اول مقاله
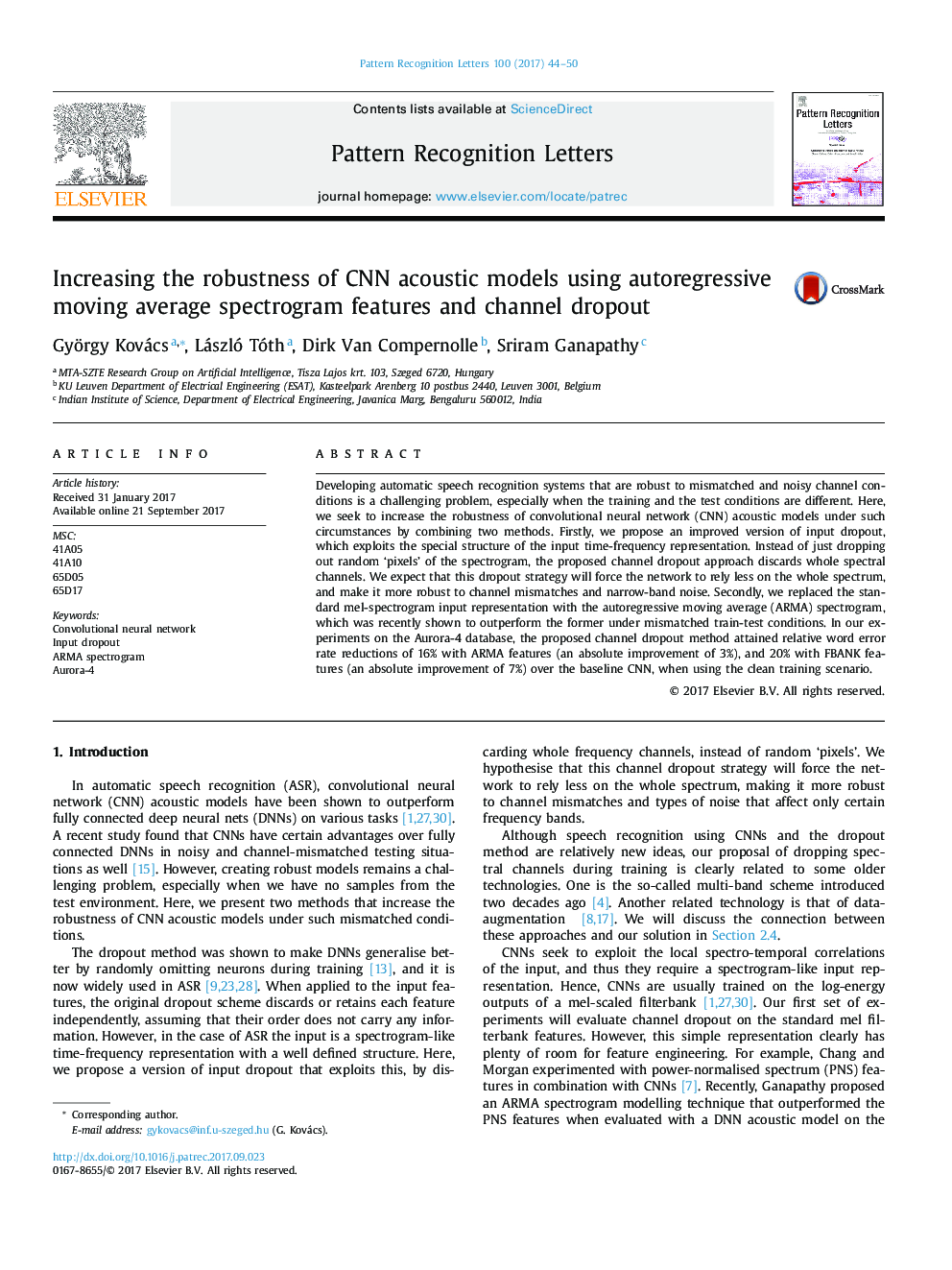
چکیده انگلیسی
Developing automatic speech recognition systems that are robust to mismatched and noisy channel conditions is a challenging problem, especially when the training and the test conditions are different. Here, we seek to increase the robustness of convolutional neural network (CNN) acoustic models under such circumstances by combining two methods. Firstly, we propose an improved version of input dropout, which exploits the special structure of the input time-frequency representation. Instead of just dropping out random 'pixels' of the spectrogram, the proposed channel dropout approach discards whole spectral channels. We expect that this dropout strategy will force the network to rely less on the whole spectrum, and make it more robust to channel mismatches and narrow-band noise. Secondly, we replaced the standard mel-spectrogram input representation with the autoregressive moving average (ARMA) spectrogram, which was recently shown to outperform the former under mismatched train-test conditions. In our experiments on the Aurora-4 database, the proposed channel dropout method attained relative word error rate reductions of 16% with ARMA features (an absolute improvement of 3%), and 20% with FBANK features (an absolute improvement of 7%) over the baseline CNN, when using the clean training scenario.
ناشر
Database: Elsevier - ScienceDirect (ساینس دایرکت)
Journal: Pattern Recognition Letters - Volume 100, 1 December 2017, Pages 44-50
Journal: Pattern Recognition Letters - Volume 100, 1 December 2017, Pages 44-50
نویسندگان
György Kovács, László Tóth, Dirk Van Compernolle, Sriram Ganapathy,