کد مقاله | کد نشریه | سال انتشار | مقاله انگلیسی | نسخه تمام متن |
---|---|---|---|---|
4972425 | 1451048 | 2017 | 46 صفحه PDF | دانلود رایگان |
عنوان انگلیسی مقاله ISI
Incorporating association rule networks in feature category-weighted naive Bayes model to support weaning decision making
دانلود مقاله + سفارش ترجمه
دانلود مقاله ISI انگلیسی
رایگان برای ایرانیان
موضوعات مرتبط
مهندسی و علوم پایه
مهندسی کامپیوتر
سیستم های اطلاعاتی
پیش نمایش صفحه اول مقاله
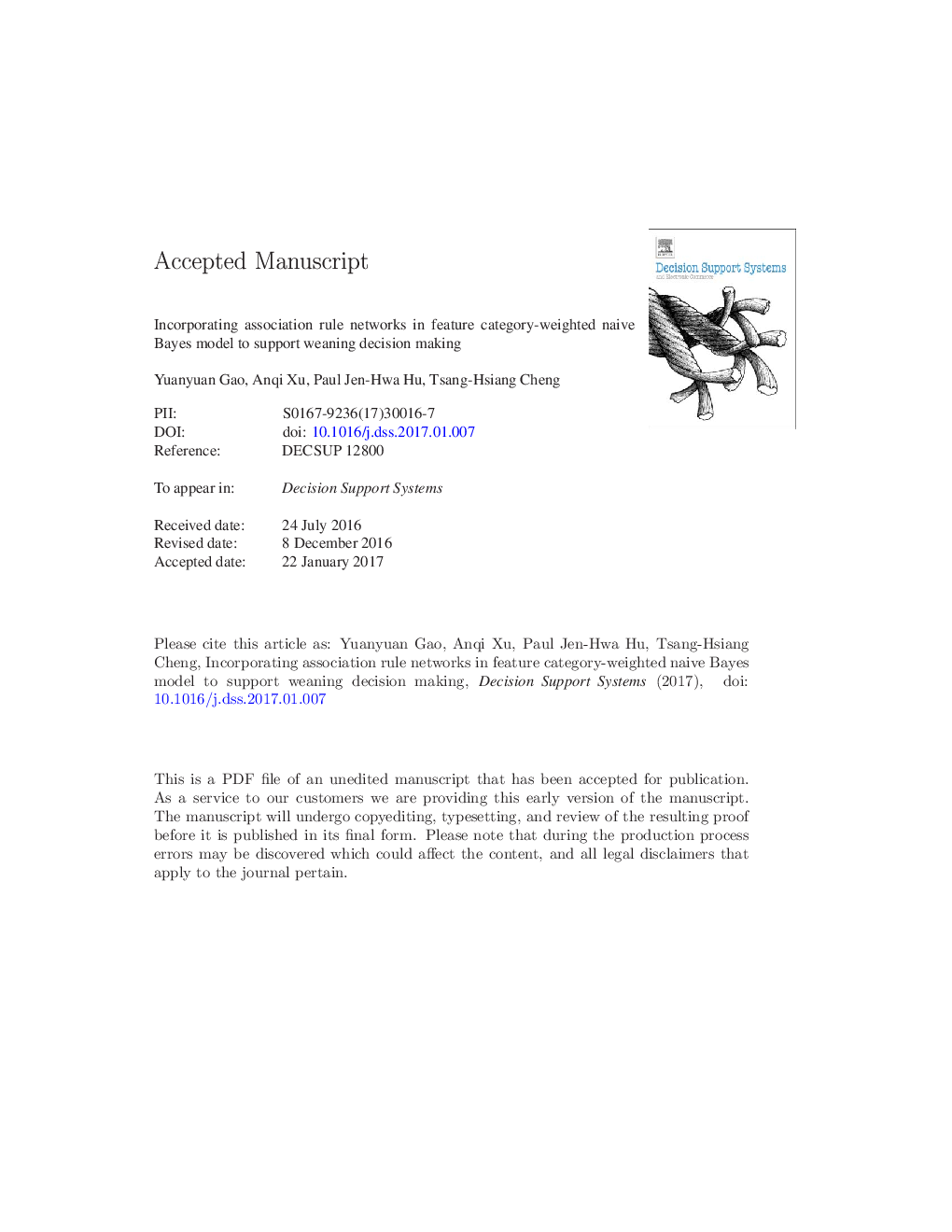
چکیده انگلیسی
Mechanical ventilation is an invasive intervention commonly used in the intensive care unit to assist patients' respirations. Physicians' decisions to wean patients from ventilation are critical: Effective weaning decisions improve patient care and well-being, but ineffective decisions can create serious severe consequences and complications. Data-driven approaches, enabled by appropriate data mining techniques, can support physicians' weaning decisions. A review of the existing techniques reveals several gaps. Specifically, most techniques assume that a feature can contribute equally to different outcome classes, overlook the “fuzzy region” issue, and assess the importance of individual features holistically rather than scrutinize the discriminant power of distinctive categories of a feature toward each decision outcome class. To address these backdrops, we propose an association rule network-based feature category-weighted naive Bayes method capable of dealing with the inherent challenges in weaning decision making. Our method analyzes feature category weights for each decision outcome by incorporating association rule learning with weighted network analysis, then applies a category-weighted naive Bayes model that can assign differential weights to various feature categories. The results of our empirical evaluation, including several prevalent techniques-artificial neural network (ANN), ANN with backward feature selection, support vector machine (SVM), and SVM with logistical regression based feature selection-indicate that the proposed method consistently outperforms all the benchmark techniques in terms of accuracy, precision, recall and F measure.
ناشر
Database: Elsevier - ScienceDirect (ساینس دایرکت)
Journal: Decision Support Systems - Volume 96, April 2017, Pages 27-38
Journal: Decision Support Systems - Volume 96, April 2017, Pages 27-38
نویسندگان
Yuanyuan Gao, Anqi Xu, Paul Jen-Hwa Hu, Tsang-Hsiang Cheng,