کد مقاله | کد نشریه | سال انتشار | مقاله انگلیسی | نسخه تمام متن |
---|---|---|---|---|
4973658 | 1451683 | 2017 | 24 صفحه PDF | دانلود رایگان |
عنوان انگلیسی مقاله ISI
Regularization of neural network model with distance metric learning for i-vector based spoken language identification
دانلود مقاله + سفارش ترجمه
دانلود مقاله ISI انگلیسی
رایگان برای ایرانیان
موضوعات مرتبط
مهندسی و علوم پایه
مهندسی کامپیوتر
پردازش سیگنال
پیش نمایش صفحه اول مقاله
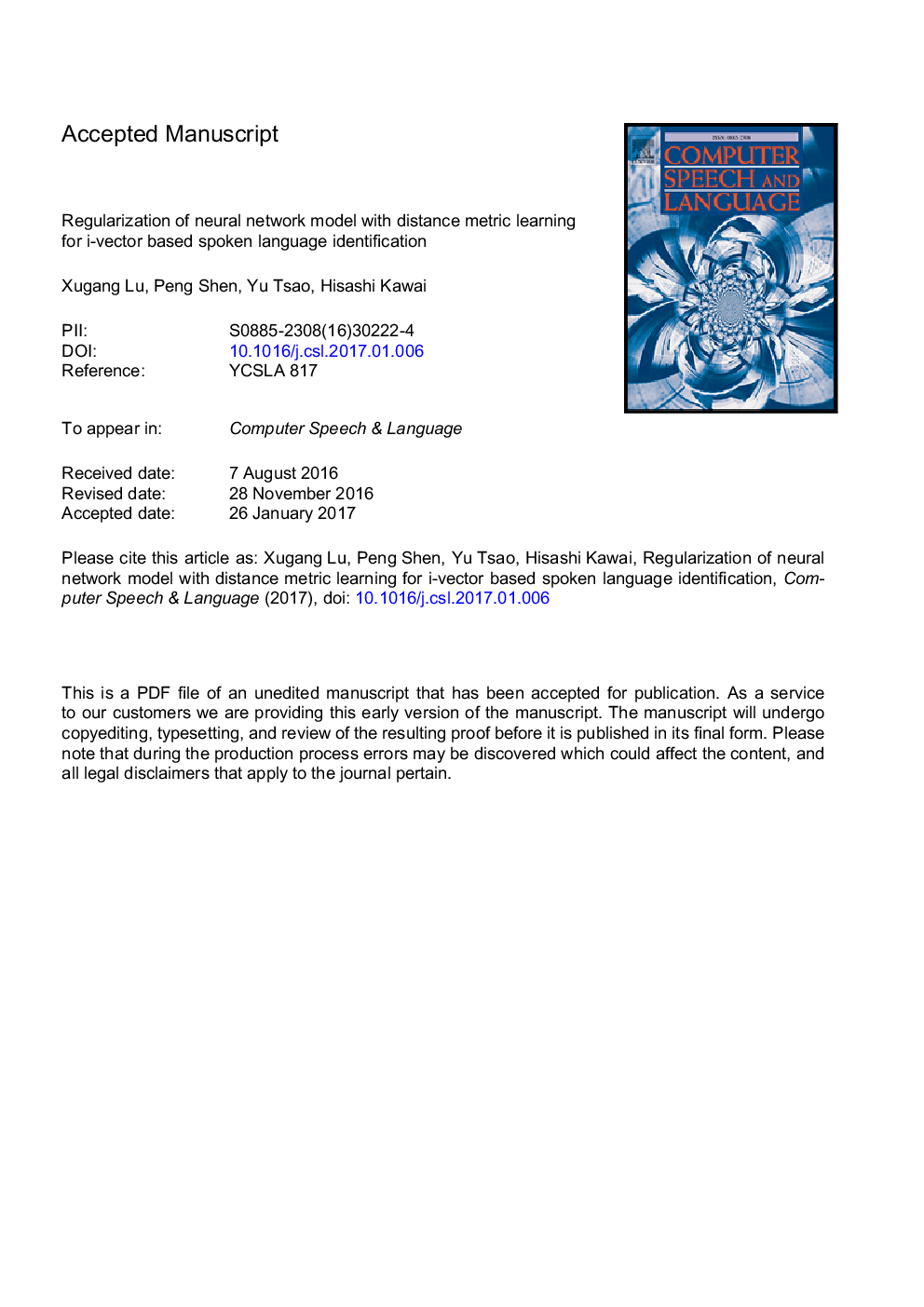
چکیده انگلیسی
The i-vector representation and modeling technique has been successfully applied in spoken language identification (SLI). The advantage of using the i-vector representation is that any speech utterance with a variable duration length can be represented as a fixed length vector. In modeling, a discriminative transform or classifier must be applied to emphasize the variations correlated to language identity since the i-vector representation encodes several types of the acoustic variations (e.g., speaker variation, transmission channel variation, etc.). Owing to the strong nonlinear discriminative power, the neural network model has been directly used to learn the mapping function between the i-vector representation and the language identity labels. In most studies, only the point-wise feature-label information is fed to the model for parameter learning that may result in model overfitting, particularly when with limited training data. In this study, we propose to integrate pair-wise distance metric learning as the regularization of model parameter optimization. In the representation space of nonlinear transforms in the hidden layers, a distance metric learning is explicitly designed to minimize the pair-wise intra-class variation and maximize the inter-class variation. Using the pair-wise distance metric learning, the i-vectors are transformed to a new feature space, wherein they are much more discriminative for samples belonging to different languages while being much more similar for samples belonging to the same language. We tested the algorithm on an SLI task, and obtained promising results, which outperformed conventional regularization methods.
ناشر
Database: Elsevier - ScienceDirect (ساینس دایرکت)
Journal: Computer Speech & Language - Volume 44, July 2017, Pages 48-60
Journal: Computer Speech & Language - Volume 44, July 2017, Pages 48-60
نویسندگان
Xugang Lu, Peng Shen, Yu Tsao, Hisashi Kawai,