کد مقاله | کد نشریه | سال انتشار | مقاله انگلیسی | نسخه تمام متن |
---|---|---|---|---|
4977780 | 1452008 | 2017 | 13 صفحه PDF | دانلود رایگان |
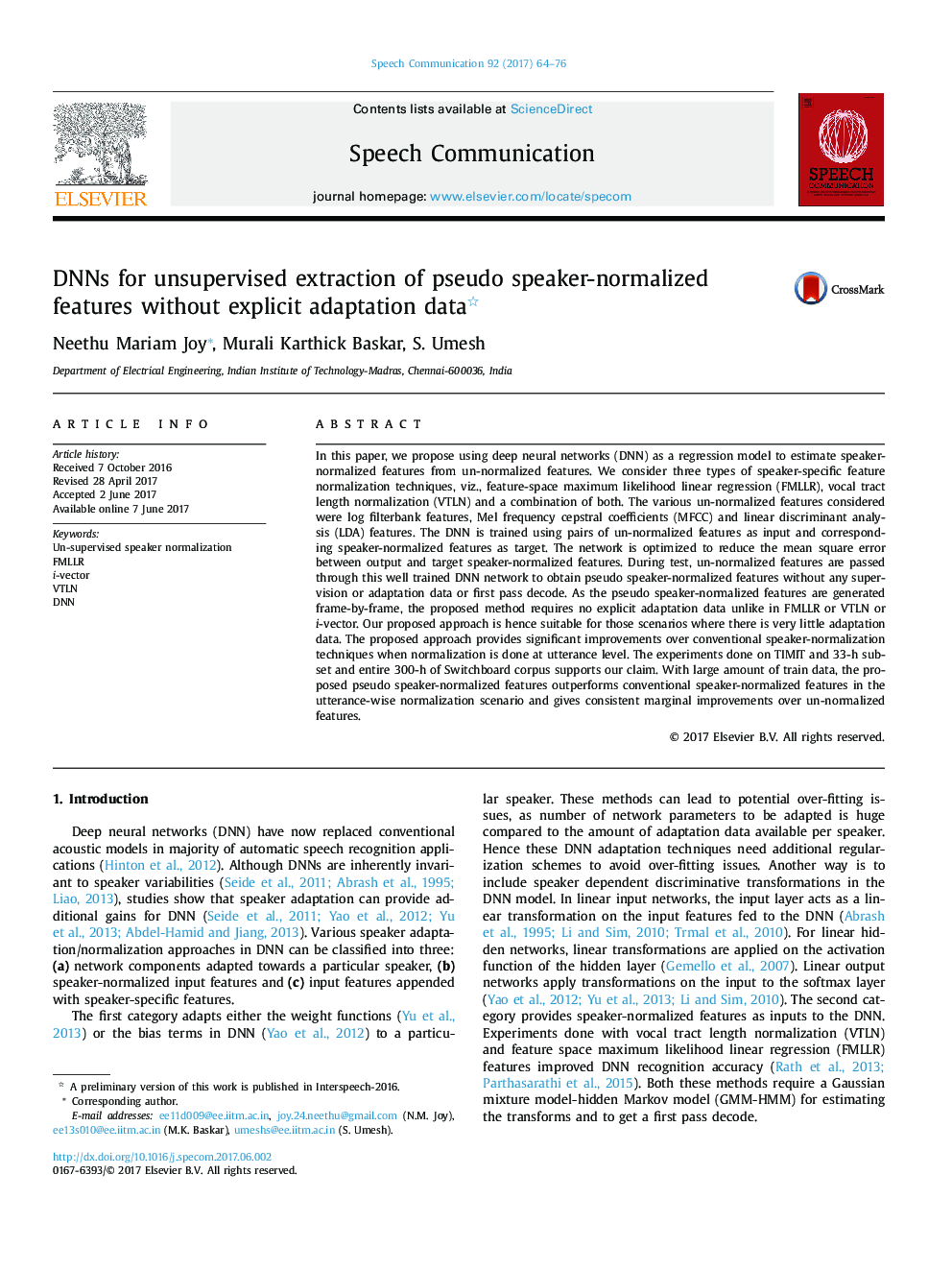
In this paper, we propose using deep neural networks (DNN) as a regression model to estimate speaker-normalized features from un-normalized features. We consider three types of speaker-specific feature normalization techniques, viz., feature-space maximum likelihood linear regression (FMLLR), vocal tract length normalization (VTLN) and a combination of both. The various un-normalized features considered were log filterbank features, Mel frequency cepstral coefficients (MFCC) and linear discriminant analysis (LDA) features. The DNN is trained using pairs of un-normalized features as input and corresponding speaker-normalized features as target. The network is optimized to reduce the mean square error between output and target speaker-normalized features. During test, un-normalized features are passed through this well trained DNN network to obtain pseudo speaker-normalized features without any supervision or adaptation data or first pass decode. As the pseudo speaker-normalized features are generated frame-by-frame, the proposed method requires no explicit adaptation data unlike in FMLLR or VTLN or i-vector. Our proposed approach is hence suitable for those scenarios where there is very little adaptation data. The proposed approach provides significant improvements over conventional speaker-normalization techniques when normalization is done at utterance level. The experiments done on TIMIT and 33-h subset and entire 300-h of Switchboard corpus supports our claim. With large amount of train data, the proposed pseudo speaker-normalized features outperforms conventional speaker-normalized features in the utterance-wise normalization scenario and gives consistent marginal improvements over un-normalized features.
Journal: Speech Communication - Volume 92, September 2017, Pages 64-76