کد مقاله | کد نشریه | سال انتشار | مقاله انگلیسی | نسخه تمام متن |
---|---|---|---|---|
504066 | 864267 | 2015 | 10 صفحه PDF | دانلود رایگان |
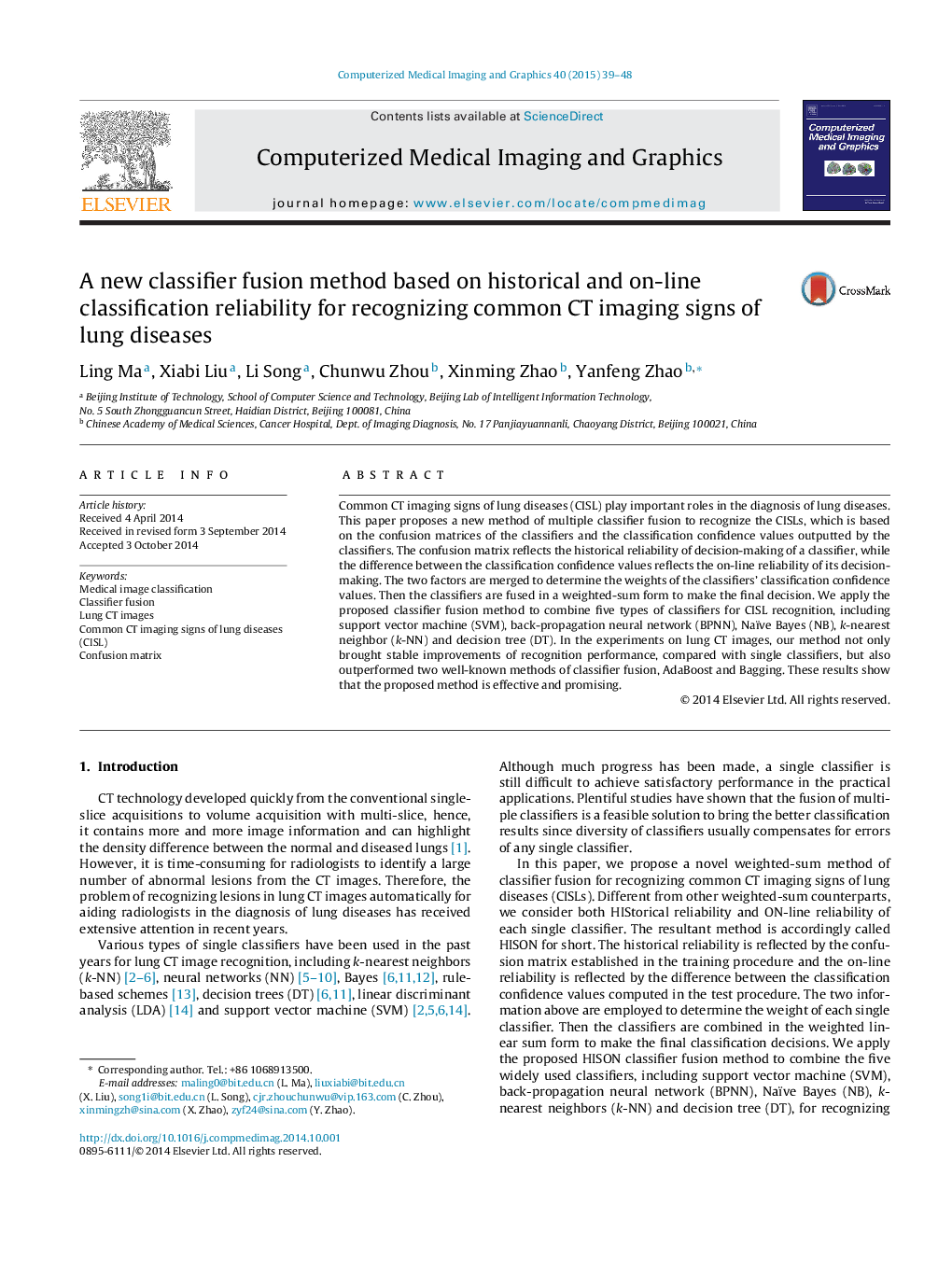
• CISLs play important roles in the diagnosis of lung diseases.
• We fuse the classifiers in a weighted-sum form for recognizing CISLs.
• The weights are based on the confusion matrix and classification confidence values.
• The weights reflect the historical and on-line reliability of decision-making of classifiers.
• Our method is robust to classifiers and features.
Common CT imaging signs of lung diseases (CISL) play important roles in the diagnosis of lung diseases. This paper proposes a new method of multiple classifier fusion to recognize the CISLs, which is based on the confusion matrices of the classifiers and the classification confidence values outputted by the classifiers. The confusion matrix reflects the historical reliability of decision-making of a classifier, while the difference between the classification confidence values reflects the on-line reliability of its decision-making. The two factors are merged to determine the weights of the classifiers’ classification confidence values. Then the classifiers are fused in a weighted-sum form to make the final decision. We apply the proposed classifier fusion method to combine five types of classifiers for CISL recognition, including support vector machine (SVM), back-propagation neural network (BPNN), Naïve Bayes (NB), k-nearest neighbor (k-NN) and decision tree (DT). In the experiments on lung CT images, our method not only brought stable improvements of recognition performance, compared with single classifiers, but also outperformed two well-known methods of classifier fusion, AdaBoost and Bagging. These results show that the proposed method is effective and promising.
Journal: Computerized Medical Imaging and Graphics - Volume 40, March 2015, Pages 39–48