کد مقاله | کد نشریه | سال انتشار | مقاله انگلیسی | نسخه تمام متن |
---|---|---|---|---|
507882 | 865152 | 2013 | 13 صفحه PDF | دانلود رایگان |
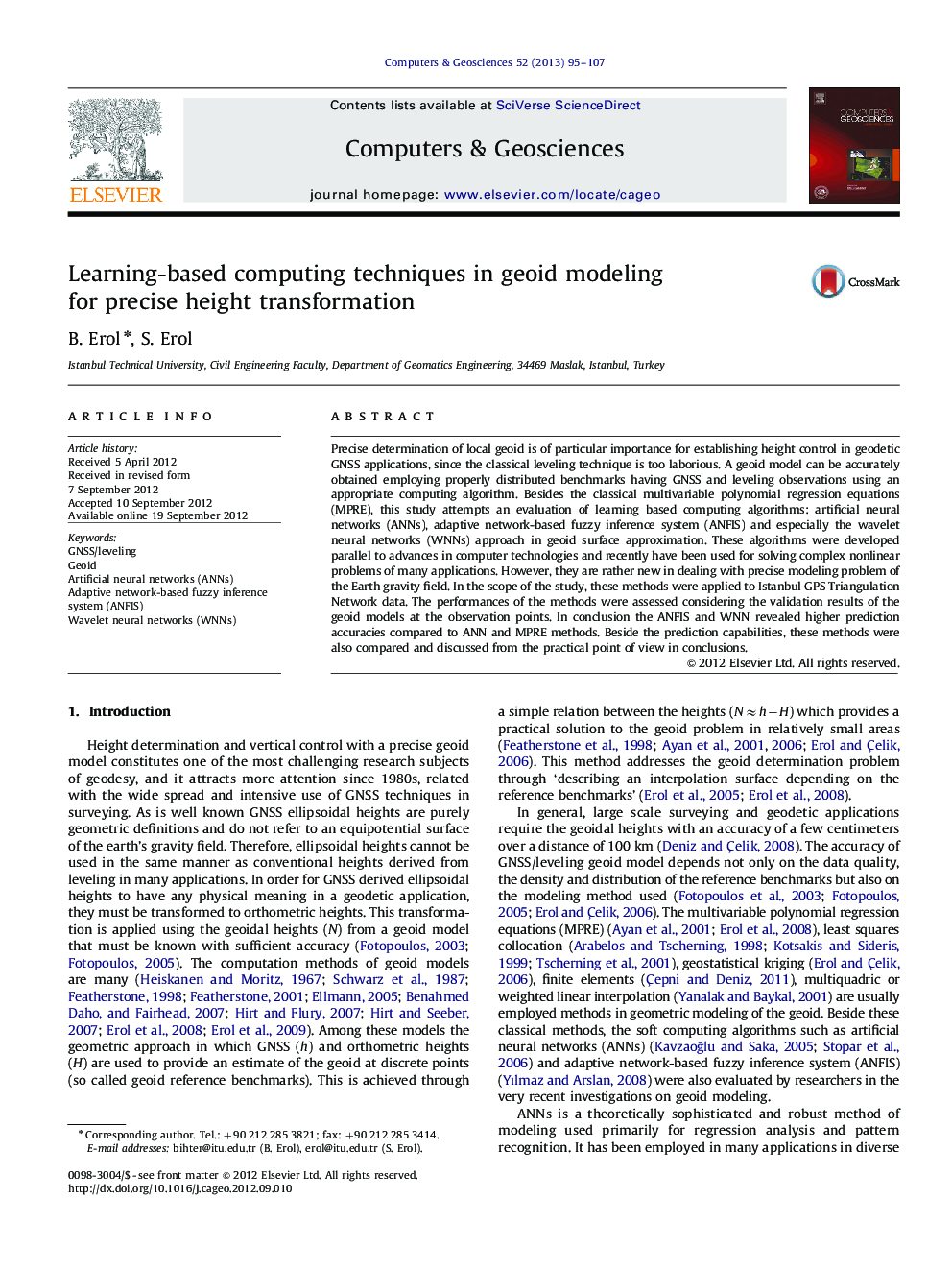
Precise determination of local geoid is of particular importance for establishing height control in geodetic GNSS applications, since the classical leveling technique is too laborious. A geoid model can be accurately obtained employing properly distributed benchmarks having GNSS and leveling observations using an appropriate computing algorithm. Besides the classical multivariable polynomial regression equations (MPRE), this study attempts an evaluation of learning based computing algorithms: artificial neural networks (ANNs), adaptive network-based fuzzy inference system (ANFIS) and especially the wavelet neural networks (WNNs) approach in geoid surface approximation. These algorithms were developed parallel to advances in computer technologies and recently have been used for solving complex nonlinear problems of many applications. However, they are rather new in dealing with precise modeling problem of the Earth gravity field. In the scope of the study, these methods were applied to Istanbul GPS Triangulation Network data. The performances of the methods were assessed considering the validation results of the geoid models at the observation points. In conclusion the ANFIS and WNN revealed higher prediction accuracies compared to ANN and MPRE methods. Beside the prediction capabilities, these methods were also compared and discussed from the practical point of view in conclusions.
► Precise determination of geoid is essential for establishing geodetic height control.
► We assessed soft-computing methods in precise local geoid modeling.
► Deciding optimum parameters is essential for precision in approximation.
► Superiority of WNN and ANFIS versus ANN and MPRE is due to their higher nonlinearity.
Journal: Computers & Geosciences - Volume 52, March 2013, Pages 95–107