کد مقاله | کد نشریه | سال انتشار | مقاله انگلیسی | نسخه تمام متن |
---|---|---|---|---|
5132209 | 1491515 | 2017 | 14 صفحه PDF | دانلود رایگان |
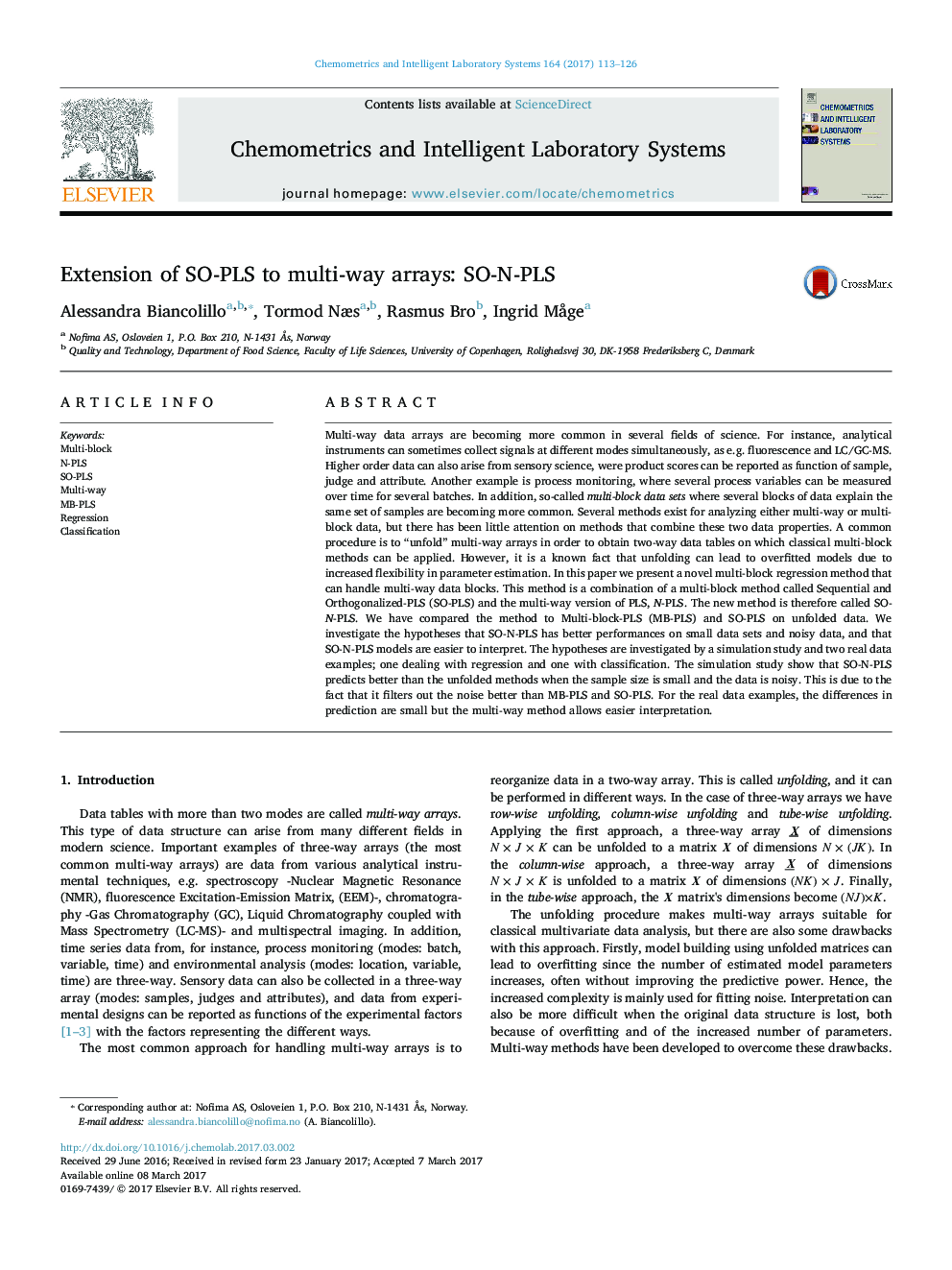
- Extension of SO-PLS to multi-way arrays: SO-N-PLS does not require unfolding.
- SO-N-PLS filters out the noise better than SO-PLS and MB-PLS.
- In simulations, SO-N-PLS outperforms SO-PLS and MB-PLS handling small and noisy data.
- Interpretation tools take into account the three-way nature of the data.
Multi-way data arrays are becoming more common in several fields of science. For instance, analytical instruments can sometimes collect signals at different modes simultaneously, as e.g. fluorescence and LC/GC-MS. Higher order data can also arise from sensory science, were product scores can be reported as function of sample, judge and attribute. Another example is process monitoring, where several process variables can be measured over time for several batches. In addition, so-called multi-block data sets where several blocks of data explain the same set of samples are becoming more common. Several methods exist for analyzing either multi-way or multi-block data, but there has been little attention on methods that combine these two data properties. A common procedure is to “unfold” multi-way arrays in order to obtain two-way data tables on which classical multi-block methods can be applied. However, it is a known fact that unfolding can lead to overfitted models due to increased flexibility in parameter estimation. In this paper we present a novel multi-block regression method that can handle multi-way data blocks. This method is a combination of a multi-block method called Sequential and Orthogonalized-PLS (SO-PLS) and the multi-way version of PLS, N-PLS. The new method is therefore called SO-N-PLS. We have compared the method to Multi-block-PLS (MB-PLS) and SO-PLS on unfolded data. We investigate the hypotheses that SO-N-PLS has better performances on small data sets and noisy data, and that SO-N-PLS models are easier to interpret. The hypotheses are investigated by a simulation study and two real data examples; one dealing with regression and one with classification. The simulation study show that SO-N-PLS predicts better than the unfolded methods when the sample size is small and the data is noisy. This is due to the fact that it filters out the noise better than MB-PLS and SO-PLS. For the real data examples, the differences in prediction are small but the multi-way method allows easier interpretation.
Journal: Chemometrics and Intelligent Laboratory Systems - Volume 164, 15 May 2017, Pages 113-126