کد مقاله | کد نشریه | سال انتشار | مقاله انگلیسی | نسخه تمام متن |
---|---|---|---|---|
5132336 | 1491518 | 2017 | 8 صفحه PDF | دانلود رایگان |
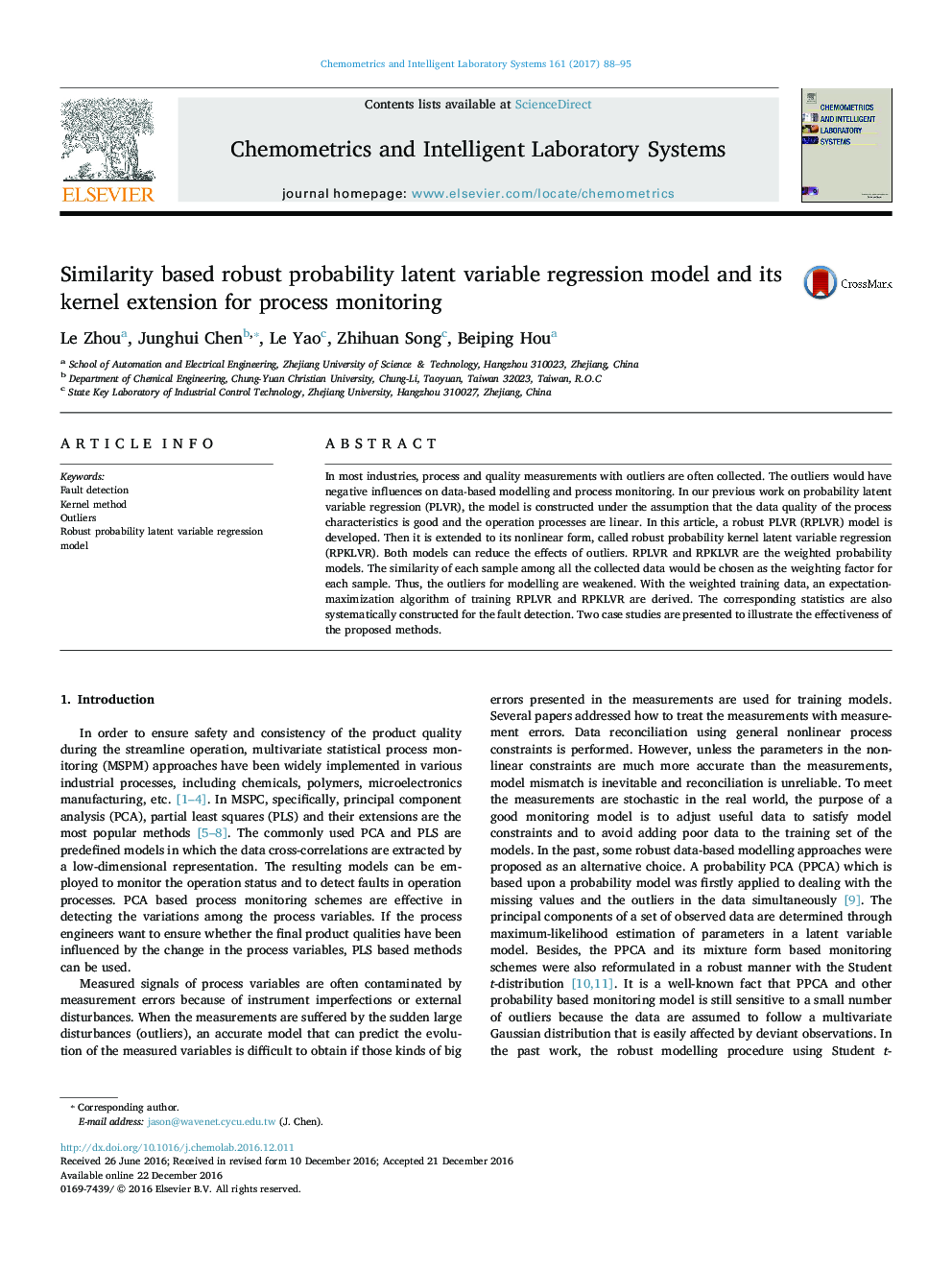
- A robust probability latent variable regression (RPLVR) is proposed.
- A robust probability kernel latent variable regression (RPKLVR) is proposed.
- RPLVR is extended to its nonlinear RKPLVR model.
- Statistics of RKPLVR for fault detection is derived utilizing the kernel trick.
- RPLVR and RKPLVR based monitoring methods for fault detection are applied.
In most industries, process and quality measurements with outliers are often collected. The outliers would have negative influences on data-based modelling and process monitoring. In our previous work on probability latent variable regression (PLVR), the model is constructed under the assumption that the data quality of the process characteristics is good and the operation processes are linear. In this article, a robust PLVR (RPLVR) model is developed. Then it is extended to its nonlinear form, called robust probability kernel latent variable regression (RPKLVR). Both models can reduce the effects of outliers. RPLVR and RPKLVR are the weighted probability models. The similarity of each sample among all the collected data would be chosen as the weighting factor for each sample. Thus, the outliers for modelling are weakened. With the weighted training data, an expectation-maximization algorithm of training RPLVR and RPKLVR are derived. The corresponding statistics are also systematically constructed for the fault detection. Two case studies are presented to illustrate the effectiveness of the proposed methods.
Journal: Chemometrics and Intelligent Laboratory Systems - Volume 161, 15 February 2017, Pages 88-95