کد مقاله | کد نشریه | سال انتشار | مقاله انگلیسی | نسخه تمام متن |
---|---|---|---|---|
525722 | 869018 | 2015 | 12 صفحه PDF | دانلود رایگان |
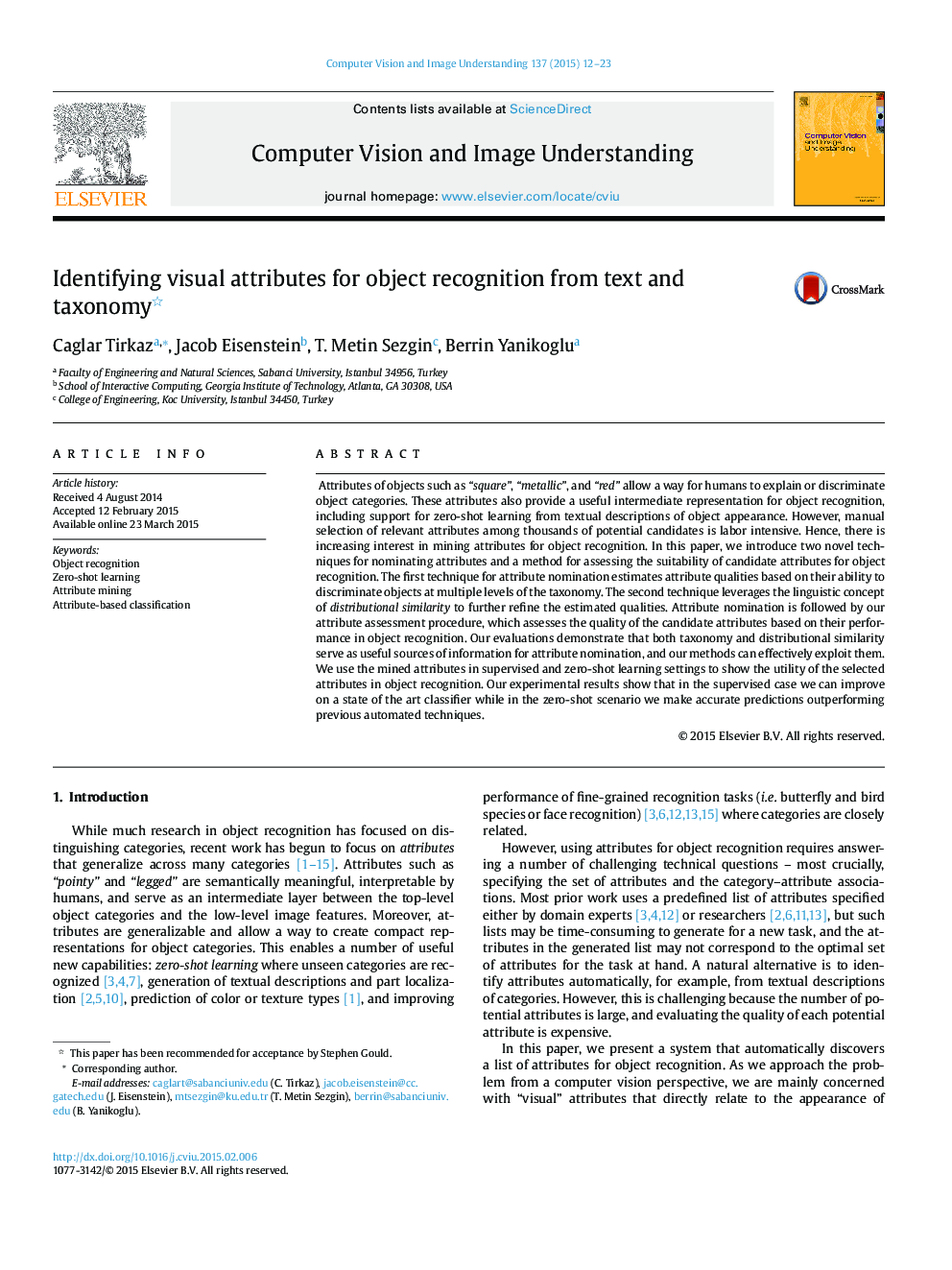
• A system to automatically mine attributes for object recognition is proposed.
• A taxonomy defined over classes is used to mine attributes.
• We integrate distributional similarity to improve the quality of mined attributes.
• The mined attributes are used in supervised and zero-shot learning settings.
• We report improved recognition accuracies compared to state of the art methods.
Attributes of objects such as “square”, “metallic”, and “red” allow a way for humans to explain or discriminate object categories. These attributes also provide a useful intermediate representation for object recognition, including support for zero-shot learning from textual descriptions of object appearance. However, manual selection of relevant attributes among thousands of potential candidates is labor intensive. Hence, there is increasing interest in mining attributes for object recognition. In this paper, we introduce two novel techniques for nominating attributes and a method for assessing the suitability of candidate attributes for object recognition. The first technique for attribute nomination estimates attribute qualities based on their ability to discriminate objects at multiple levels of the taxonomy. The second technique leverages the linguistic concept of distributional similarity to further refine the estimated qualities. Attribute nomination is followed by our attribute assessment procedure, which assesses the quality of the candidate attributes based on their performance in object recognition. Our evaluations demonstrate that both taxonomy and distributional similarity serve as useful sources of information for attribute nomination, and our methods can effectively exploit them. We use the mined attributes in supervised and zero-shot learning settings to show the utility of the selected attributes in object recognition. Our experimental results show that in the supervised case we can improve on a state of the art classifier while in the zero-shot scenario we make accurate predictions outperforming previous automated techniques.
Journal: Computer Vision and Image Understanding - Volume 137, August 2015, Pages 12–23