کد مقاله | کد نشریه | سال انتشار | مقاله انگلیسی | نسخه تمام متن |
---|---|---|---|---|
527784 | 869360 | 2013 | 13 صفحه PDF | دانلود رایگان |
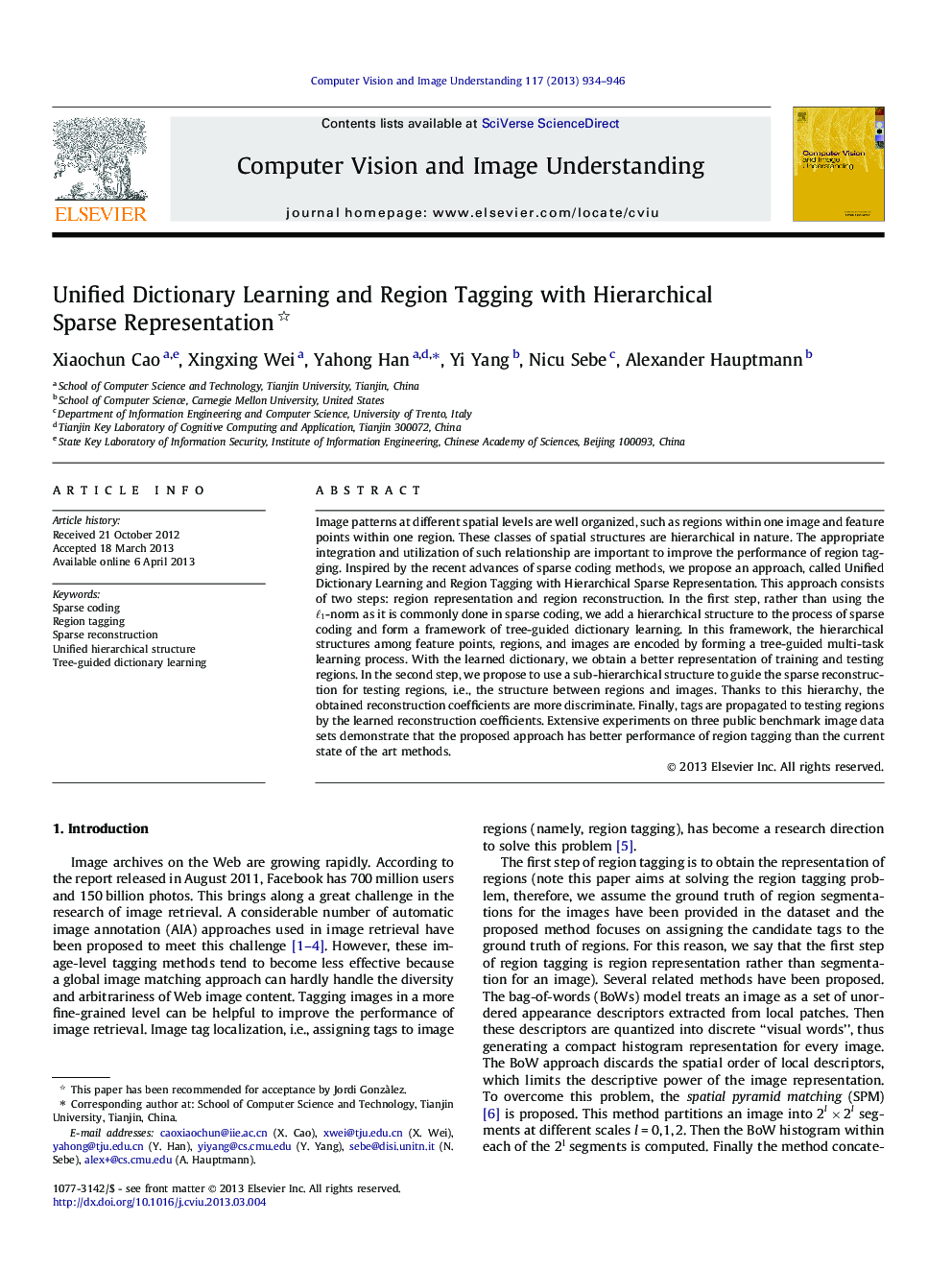
• Hierarchical structure among feature points, regions, and images are explored.
• We add a hierarchical structure to the process of sparse coding.
• Region sparse reconstruction is guided by the hierarchical structure.
• Dictionary learning and region tagging are unified into a new framework.
• Achieve better performance compared with state-of-the-art methods.
Image patterns at different spatial levels are well organized, such as regions within one image and feature points within one region. These classes of spatial structures are hierarchical in nature. The appropriate integration and utilization of such relationship are important to improve the performance of region tagging. Inspired by the recent advances of sparse coding methods, we propose an approach, called Unified Dictionary Learning and Region Tagging with Hierarchical Sparse Representation. This approach consists of two steps: region representation and region reconstruction. In the first step, rather than using the ℓ1ℓ1-norm as it is commonly done in sparse coding, we add a hierarchical structure to the process of sparse coding and form a framework of tree-guided dictionary learning. In this framework, the hierarchical structures among feature points, regions, and images are encoded by forming a tree-guided multi-task learning process. With the learned dictionary, we obtain a better representation of training and testing regions. In the second step, we propose to use a sub-hierarchical structure to guide the sparse reconstruction for testing regions, i.e., the structure between regions and images. Thanks to this hierarchy, the obtained reconstruction coefficients are more discriminate. Finally, tags are propagated to testing regions by the learned reconstruction coefficients. Extensive experiments on three public benchmark image data sets demonstrate that the proposed approach has better performance of region tagging than the current state of the art methods.
Journal: Computer Vision and Image Understanding - Volume 117, Issue 8, August 2013, Pages 934–946